Administration of Emergency Medicine
JOURNAL OF EMERGENCY MEDICINE(2023)
摘要
Background: Work Relative Value Units (wRVUs) are a component of many compensation models, and a proxy for the effort required to care for a patient. Ac-curate prediction of wRVUs generated per patient at triage could facilitate real-time load balancing between physicians and provide many practical operational and clinical benefits. Objective: We examined whether deep-learning approaches could predict the wRVUs generated by a patient's visit using data commonly available at triage. Methods: Adult patients presenting to an urban, academic emergency department from July 1, 2016-March 1, 2020 were included. Deidenti-fied triage information included structured data (age, sex, vital signs, Emergency Severity Index score, language, race, standardized chief complaint) and unstructured data (free -text chief complaint) with wRVUs as outcome. Five models were examined: average wRVUs per chief complaint, lin-ear regression, neural network and gradient-boosted tree on structured data, and neural network on unstructured textual data. Models were evaluated using mean absolute error. Re-sults: We analyzed 204,064 visits between July 1, 2016 and March 1, 2020. The median wRVUs were 3.80 (interquar-tile range 2.56-4.21), with significant effects of age, gender, and race. Models demonstrated lower error as complexity increased. Predictions using averages from chief complaints alone demonstrated a mean error of 2.17 predicted wRVUs per visit (95% confidence interval [CI] 2.07-2.27), the lin- ear regression model: 1.00 wRVUs (95% CI 0.97-1.04), gradient-boosted tree: 0.85 wRVUs (95% CI 0.84-0.86), neu-ral network with structured data: 0.86 wRVUs (95% CI 0.85-0.87), and neural network with unstructured data: 0.78 wRVUs (95% CI 0.76-0.80). Conclusions: Chief complaints are a poor predictor of the effort needed to evaluate a patient; however, deep-learning techniques show promise. These algorithms have the potential to provide many prac-tical applications, including balancing workloads and com-pensation between emergency physicians, quantify crowding and mobilizing resources, and reducing bias in the triage process.
更多查看译文
关键词
clinical decision support, operations man-agement, machine learning, quality assurance
AI 理解论文
溯源树
样例
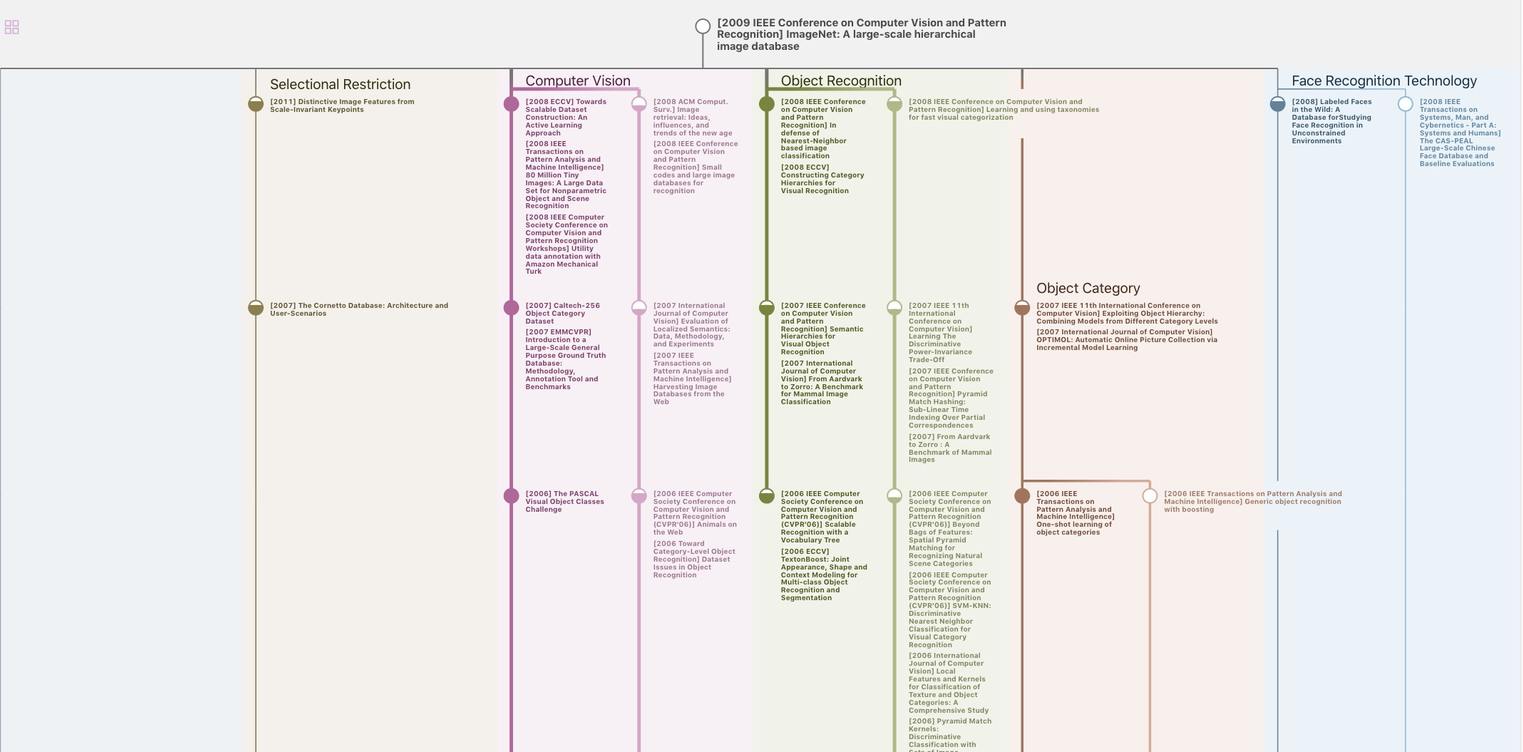
生成溯源树,研究论文发展脉络
Chat Paper
正在生成论文摘要