A transfer learning framework for well placement optimization based on denoising autoencoder
GEOENERGY SCIENCE AND ENGINEERING(2023)
摘要
Well placement optimization is directly related to the recovery factor of reservoir development, and at present, the mainstream solution is an evolutionary algorithm. However, time-consuming numerical simulators need to be called to evaluate each alternative well placement scheme. Since the rules of well placement problems are universal, similar reservoirs will have similar well locations. Thus, knowledge transfer across similar well placement optimization tasks can expedite searching effectively. To this end, this paper proposes a novel transfer learning framework for well placement optimization to extract the potential well placement rules based on the feature extraction capability of a single-layer denoising autoencoder. The reconstruction mapping between the previous and present tasks is established to make the randomly generated well locations inherit the knowledge from the optimal well locations of the previous task, which helps the search direction of the evolutionary al-gorithm quickly bias to the optimal solution, thus, the solving of present task can be accelerated. The simplified denoising autoencoder holds a closed-form solution after derivation of the loss function, and the corresponding reuse of knowledge will not bring much additional computational burden on the evolutionary search. In addition, a similarity measure method between well placement optimization tasks is proposed to avoid a negative transfer. At last, comprehensive experiments on benchmark functions and well placement optimization instances are presented to evaluate the effectiveness of the proposed framework.
更多查看译文
关键词
transfer learning framework,placement optimization,learning framework
AI 理解论文
溯源树
样例
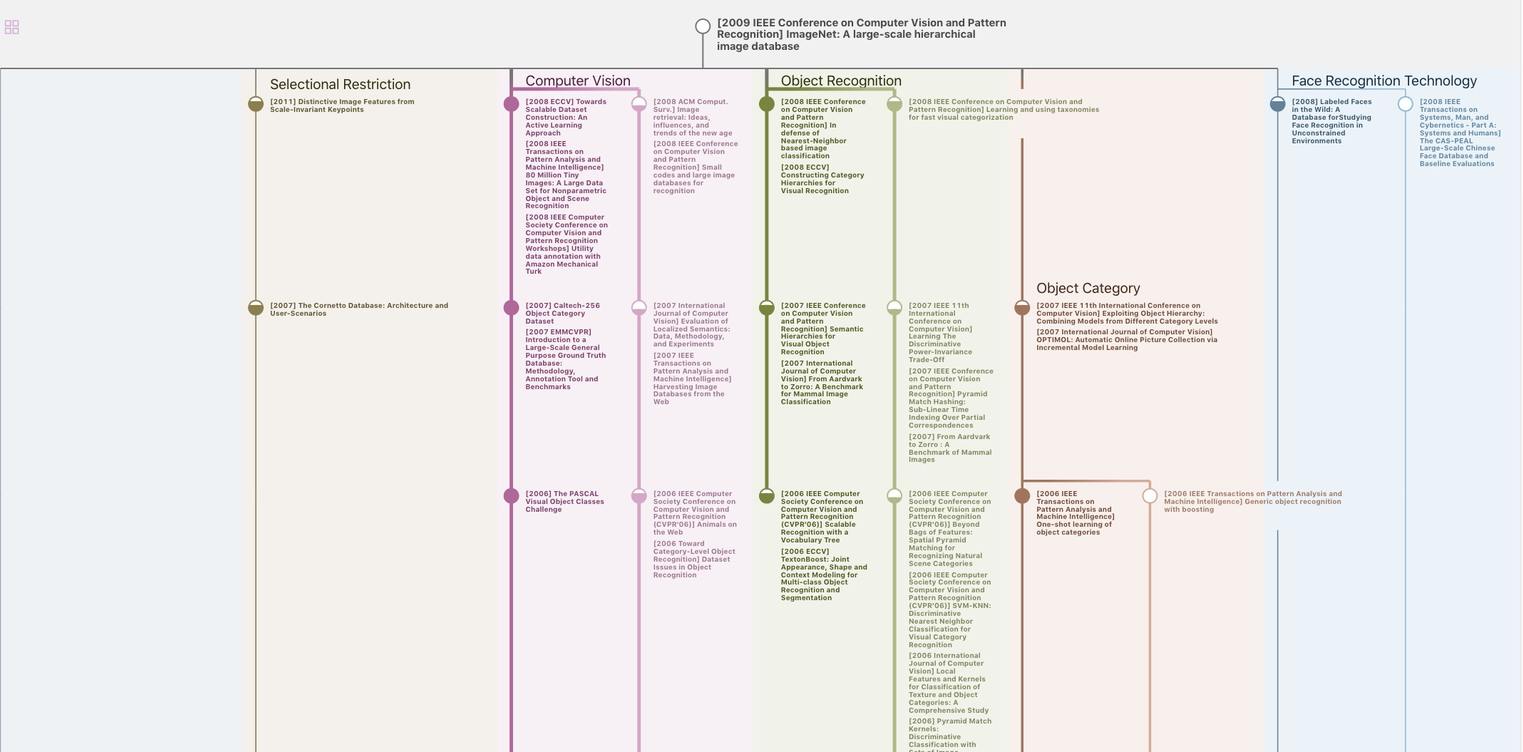
生成溯源树,研究论文发展脉络
Chat Paper
正在生成论文摘要