DCNet: exploring fine-grained vision classification for 3D point clouds
VISUAL COMPUTER(2024)
摘要
Fine-grained 3D point cloud classification is vital for shape analysis and understanding. However, due to the subtle inter-class differences and the significant intra-class variations, applying the existing point cloud network directly to fine-grained visual classification tasks may suffer overfitting and cannot achieve good performance. To address this problem, we propose a unified and robust learning framework, named dynamic confusion network (DCNet), which helps the network capture the subtle differences between samples from different sub-categoriesmore robustly. Specifically, in the stage of feature extraction, we design a novel mutual complementary mechanism between an attention block and a dynamic sample confusion block to extract more abundant discriminative features. Furthermore, we construct robust adversarial learning between a dynamic sample confusion loss and a cross-entropy loss based on a siamese network framework to make the network learn more stable feature distributions. We conduct comprehensive experiments and show that DCNet achieves the best performance in three fine-grained categories, with relative accuracy improvements of 1.35%, 1.28%, and 2.30% on Airplane, Car, and Chair, respectively, compared to state-of-the-art point cloud methods. In addition, our approach also achieves comparable performance for the coarse-grained dataset on ModelNet40.
更多查看译文
关键词
3D point clouds,Fine-grained classification,Dynamic adjustment learning,Feature extraction
AI 理解论文
溯源树
样例
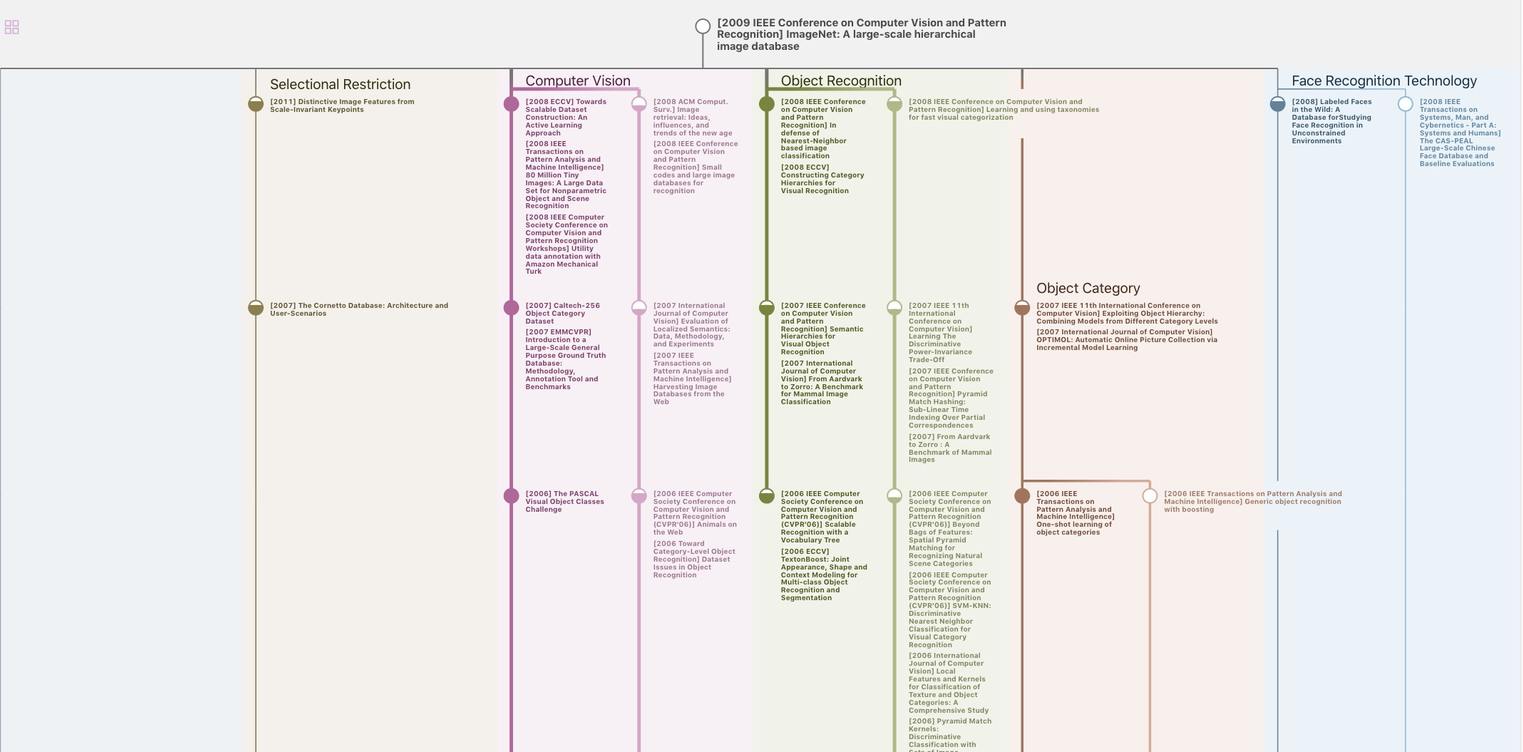
生成溯源树,研究论文发展脉络
Chat Paper
正在生成论文摘要