Image super-resolution using multi-scale non-local attention
JOURNAL OF ELECTRONIC IMAGING(2023)
摘要
Recent convolutional neural network (CNN)-based super-resolution (SR) studies incorporated non-local attention (NLA), to consider the long-range feature correlations and achieve considerable performance improvement. Here, we proposed an innovative NLA scheme called multi-scale NLA (MS-NLA) that computes NLAs at multiple scales and fuses them. To effectively fuse NLAs, we also proposed two learning-based methods and analyzed their performance on a recurrent SR network. Furthermore, the effect of weight sharing in the fusion methods is analyzed as well. In 2 x and 4 x SR experiments on benchmark datasets, our method had higher PSNR values of 0.295 and 0.148 dB on average than those using single-scale NLA and cross-scale NLA, respectively, and produced visually more pleasing SR results. The weight sharing had a limited but positive effect, depending on datasets.
更多查看译文
关键词
attention,image,super-resolution,multi-scale,non-local
AI 理解论文
溯源树
样例
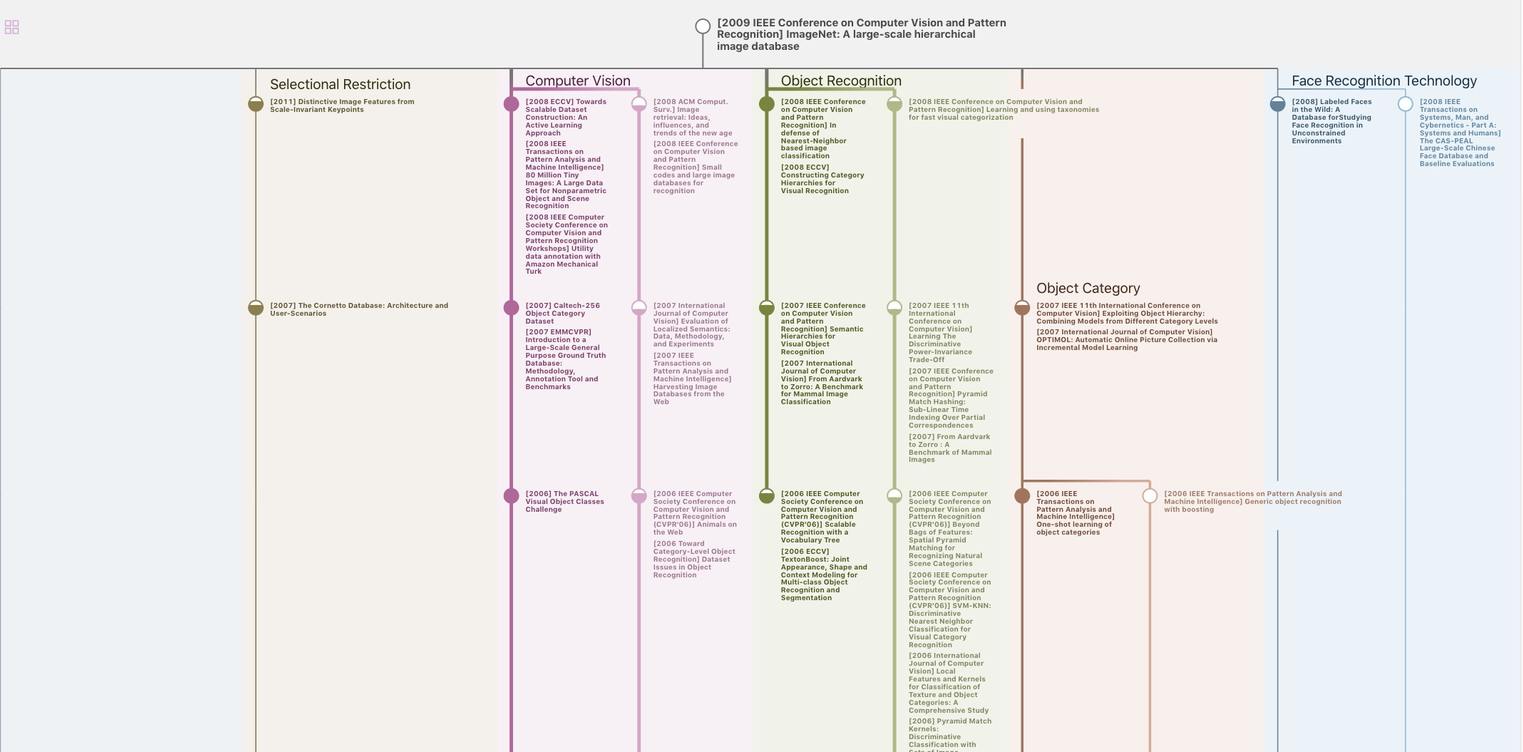
生成溯源树,研究论文发展脉络
Chat Paper
正在生成论文摘要