Research on Data-Driven Optimal Scheduling of Power System
ENERGIES(2023)
摘要
The uncertainty of output makes it difficult to effectively solve the economic security dispatching problem of the power grid when a high proportion of renewable energy generating units are integrated into the power grid. Based on the proximal policy optimization (PPO) algorithm, a safe and economical grid scheduling method is designed. First, constraints on the safe and economical operation of renewable energy power systems are defined. Then, the quintuple of Markov decision process is defined under the framework of deep reinforcement learning, and the dispatching optimization problem is transformed into Markov decision process. To solve the problem of low sample data utilization in online reinforcement learning strategies, a PPO optimization algorithm based on the Kullback-Leibler (KL) divergence penalty factor and importance sampling technique is proposed, which transforms on-policy into off-policy and improves sample utilization. Finally, the simulation analysis of the example shows that in a power system with a high proportion of renewable energy generating units connected to the grid, the proposed scheduling strategy can meet the load demand under different load trends. In the dispatch cycle with different renewable energy generation rates, renewable energy can be absorbed to the maximum extent to ensure the safe and economic operation of the grid.
更多查看译文
关键词
optimal scheduling,power system,data-driven
AI 理解论文
溯源树
样例
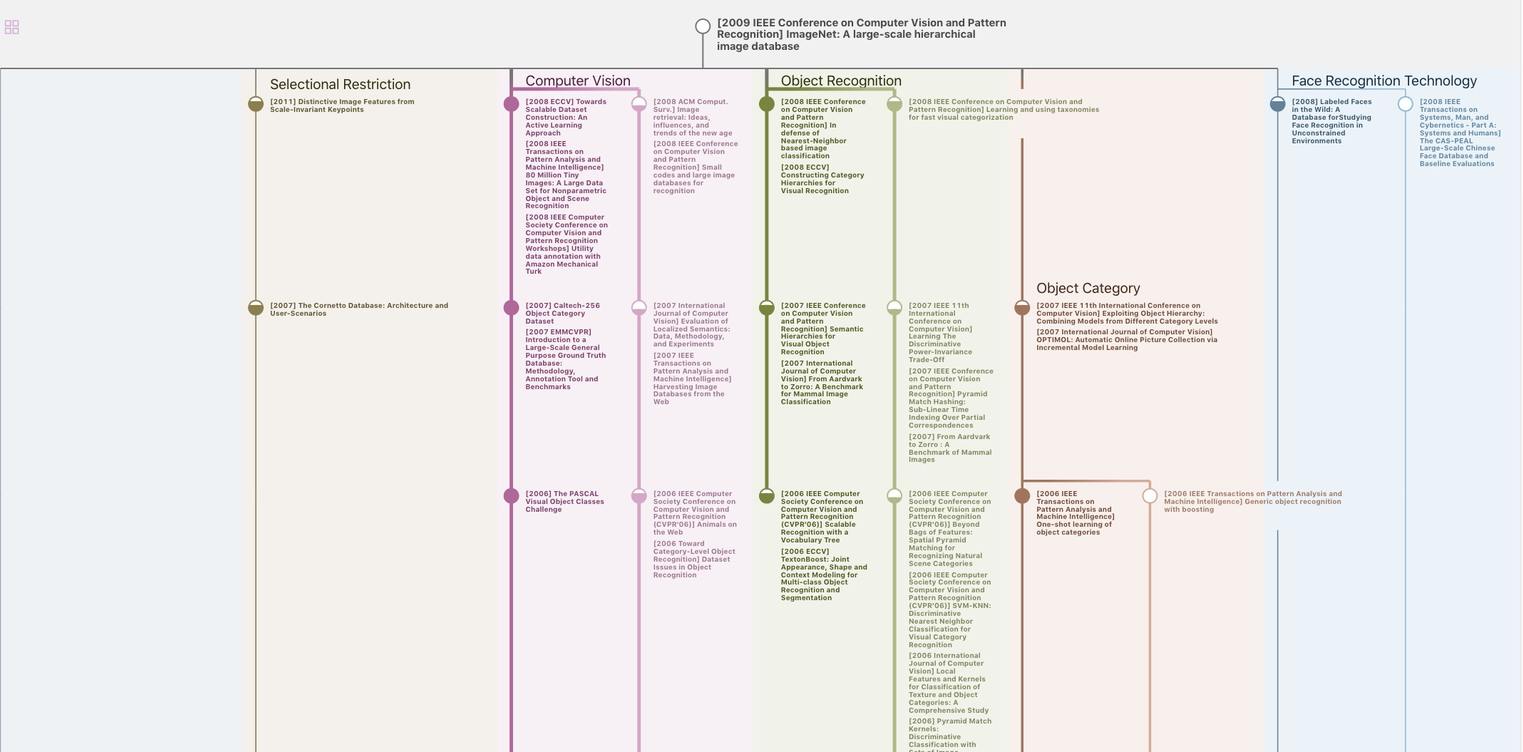
生成溯源树,研究论文发展脉络
Chat Paper
正在生成论文摘要