Integrative Structural Learning of Mixed Graphical Models via Pseudo-likelihood
STATISTICS IN BIOSCIENCES(2023)
摘要
Markov random field is a common tool to characterize interactions among a fixed collection of variables. In recent biomedical research, there arise new concerns about the discovery of regulatory and co-expression relationships among different types of features across multiple biological classes. Consequently, we propose a data integration framework to jointly learn multiple mixed graphical models simultaneously. To address the common asymmetry problem in neighborhood selection, we construct a new estimator using regularized pseudo-likelihood, which produces symmetric and consistent estimates of network topologies. We demonstrate the practical merits of our method through learning synthetic networks as well as constructing gene regulatory networks from TCGA data.
更多查看译文
关键词
Data integration,Group lasso,Joint modeling,Network,TCGA data
AI 理解论文
溯源树
样例
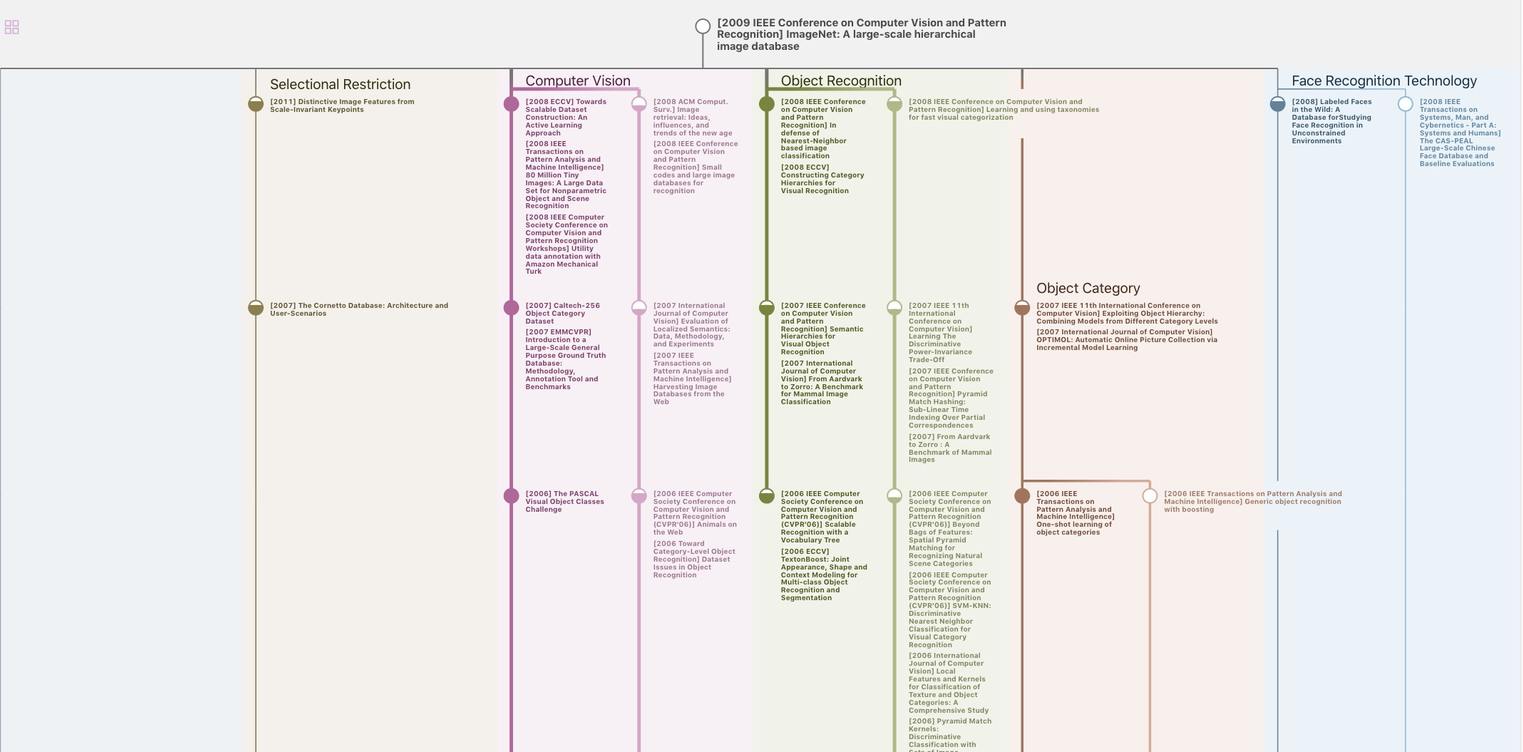
生成溯源树,研究论文发展脉络
Chat Paper
正在生成论文摘要