A Hybrid Model of Primary Ensemble Empirical Mode Decomposition and Quantum Neural Network in Financial Time Series Prediction
FLUCTUATION AND NOISE LETTERS(2023)
摘要
Financial time series are nonlinear, volatile and chaotic. Inspired by quantum computing, this paper proposed a new model, called primary ensemble empirical mode decomposition combined with quantum neural network (PEEMD-QNN) in predicting the stock index. PEEMD-QNN takes the advantages of the PEEMD which retains the main component of modal component and QNN. To demonstrate that our PEEMD-QNN model is robust, we used the new model to predict six major stock index time series in China at a specific time. Detailed experiments are implemented for both of the proposed prediction models, in which empirical mode decomposition combined with QNN (EMD-QNN), QNN and BP neural network are compared. The results demonstrate that the proposed PEEMD-QNN model has higher accuracy than BP neural network, QNN model and EMD-QNN model in stock market prediction.
更多查看译文
关键词
Quantum neural network,primary ensemble empirical mode decomposition,intrinsic mode functions (IMFs),financial time series prediction
AI 理解论文
溯源树
样例
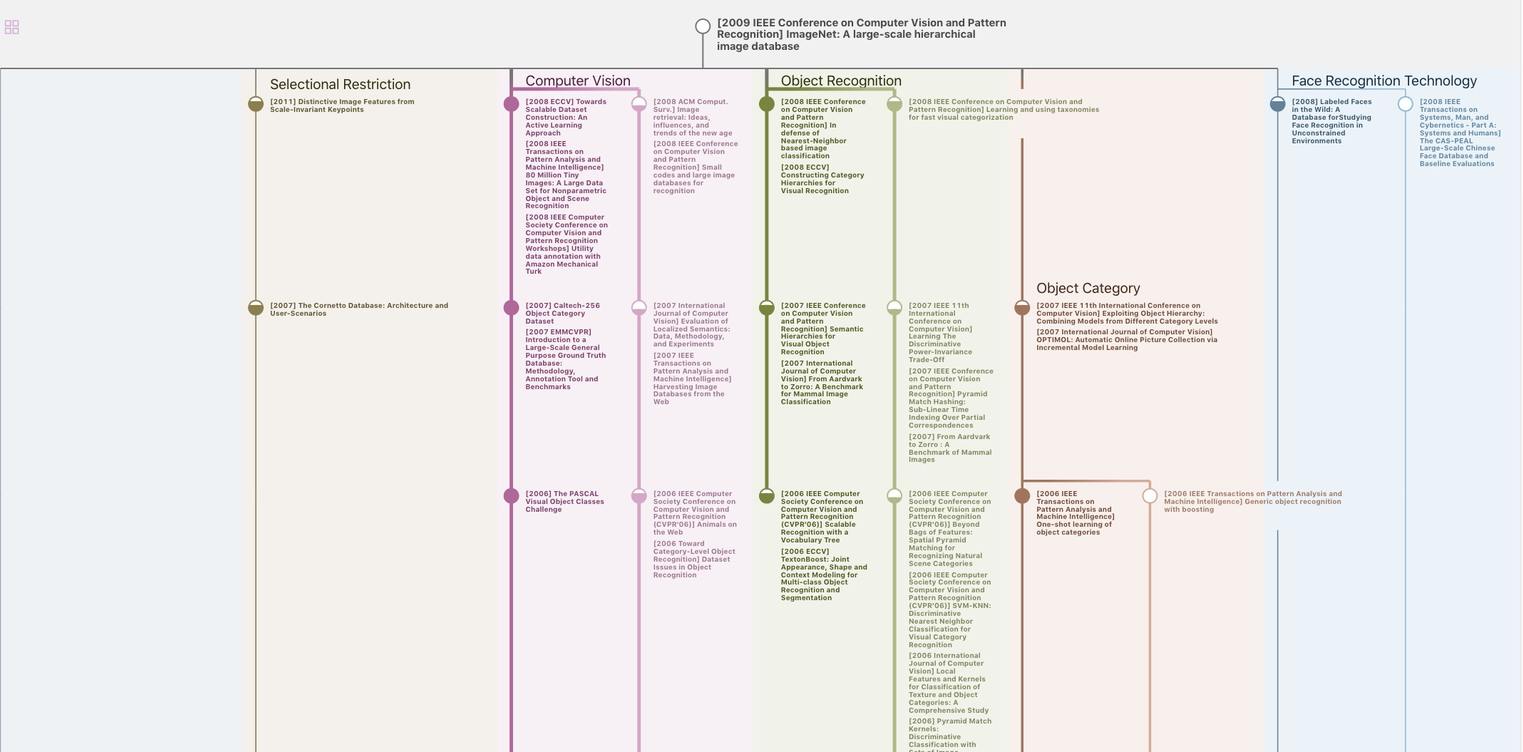
生成溯源树,研究论文发展脉络
Chat Paper
正在生成论文摘要