A Systematic Review of Individual Tree Crown Detection and Delineation with Convolutional Neural Networks (CNN)
CURRENT FORESTRY REPORTS(2023)
摘要
Purpose of Review Crown detection and measurement at the individual tree level provide detailed information for accurate forest management. To efficiently acquire such information, approaches to conduct individual tree detection and crown delineation (ITDCD) using remotely sensed data have been proposed. In recent years, deep learning, specifically convolutional neural networks (CNN), has shown potential in this field. This article provides a systematic review of the studies that used CNN for ITDCD and identifies major trends and research gaps across six perspectives: accuracy assessment methods, data types, platforms and resolutions, forest environments, CNN models, and training strategies and techniques. Recent Findings CNN models were mostly applied to high-resolution red–green–blue (RGB) images. When compared with other state-of-the-art approaches, CNN models showed significant improvements in accuracy. One study reported an increase in detection accuracy of over 11%, while two studies reported increases in F1-score of over 16%. However, model performance varied across different forest environments and data types. Several factors including data scarcity, model selection, and training approaches affected ITDCD results. Summary Future studies could (1) explore data fusion approaches to take advantage of the characteristics of different types of remote sensing data, (2) further improve data efficiency with customised sample approaches and synthetic samples, (3) explore the potential of smaller CNN models and compare their learning efficiency with commonly used models, and (4) evaluate impacts of pre-training and parameter tunings.
更多查看译文
关键词
Deep learning,Tree detection,Crown delineation,Forestry,Remote sensing,Object detection,Instance segmentation
AI 理解论文
溯源树
样例
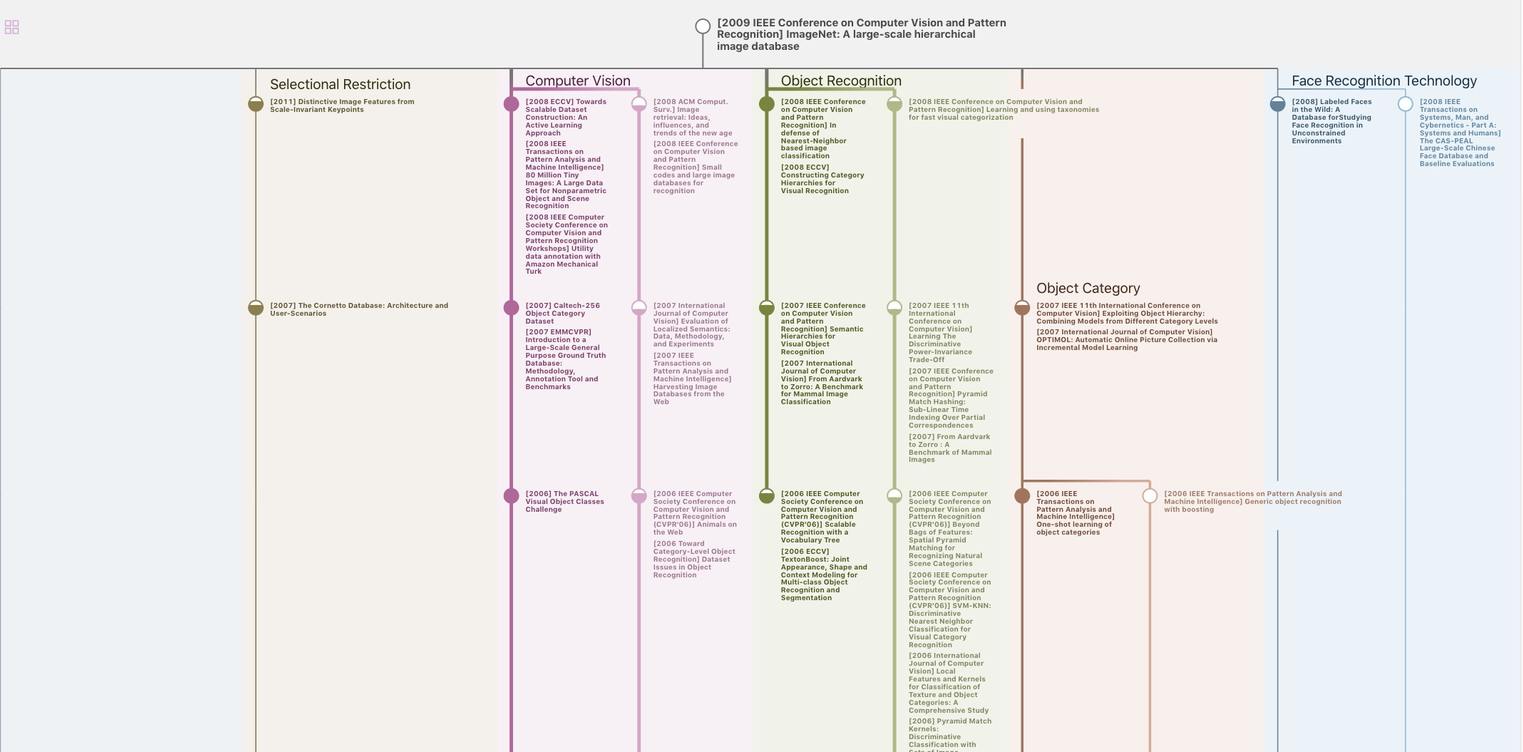
生成溯源树,研究论文发展脉络
Chat Paper
正在生成论文摘要