Energy-Efficient UAVs Coverage Path Planning Approach
CMES-COMPUTER MODELING IN ENGINEERING & SCIENCES(2023)
摘要
Unmanned aerial vehicles (UAVs), commonly known as drones, have drawn significant consideration thanks to their agility, mobility, and flexibility features. They play a crucial role in modern reconnaissance, inspection, intelligence, and surveillance missions. Coverage path planning (CPP) which is one of the crucial aspects that determines an intelligent system's quality seeks an optimal trajectory to fully cover the region of interest (ROI). However, the flight time of the UAV is limited due to a battery limitation and may not cover the whole region, especially in large region. Therefore, energy consumption is one of the most challenging issues that need to be optimized. In this paper, we propose an energy-efficient coverage path planning algorithm to solve the CPP problem. The objective is to generate a collision-free coverage path that minimizes the overall energy consumption and guarantees covering the whole region. To do so, the flight path is optimized and the number of turns is reduced to minimize the energy consumption. The proposed approach first decomposes the ROI into a set of cells depending on a UAV camera footprint. Then, the coverage path planning problem is formulated, where the exact solution is determined using the CPLEX solver. For small-scale problems, the CPLEX shows a better solution in a reasonable time. However, the CPLEX solver fails to generate the solution within a reasonable time for large-scale problems. Thus, to solve the model for large-scale problems, simulated annealing for CPP is developed. The results show that heuristic approaches yield a better solution for large-scale problems within a much shorter execution time than the CPLEX solver. Finally, we compare the simulated annealing against the greedy algorithm. The results show that simulated annealing outperforms the greedy algorithm in generating better solution quality.
更多查看译文
关键词
Coverage path planning,MILP,CPLEX solver,energy model,optimization,region of interest,area of interest
AI 理解论文
溯源树
样例
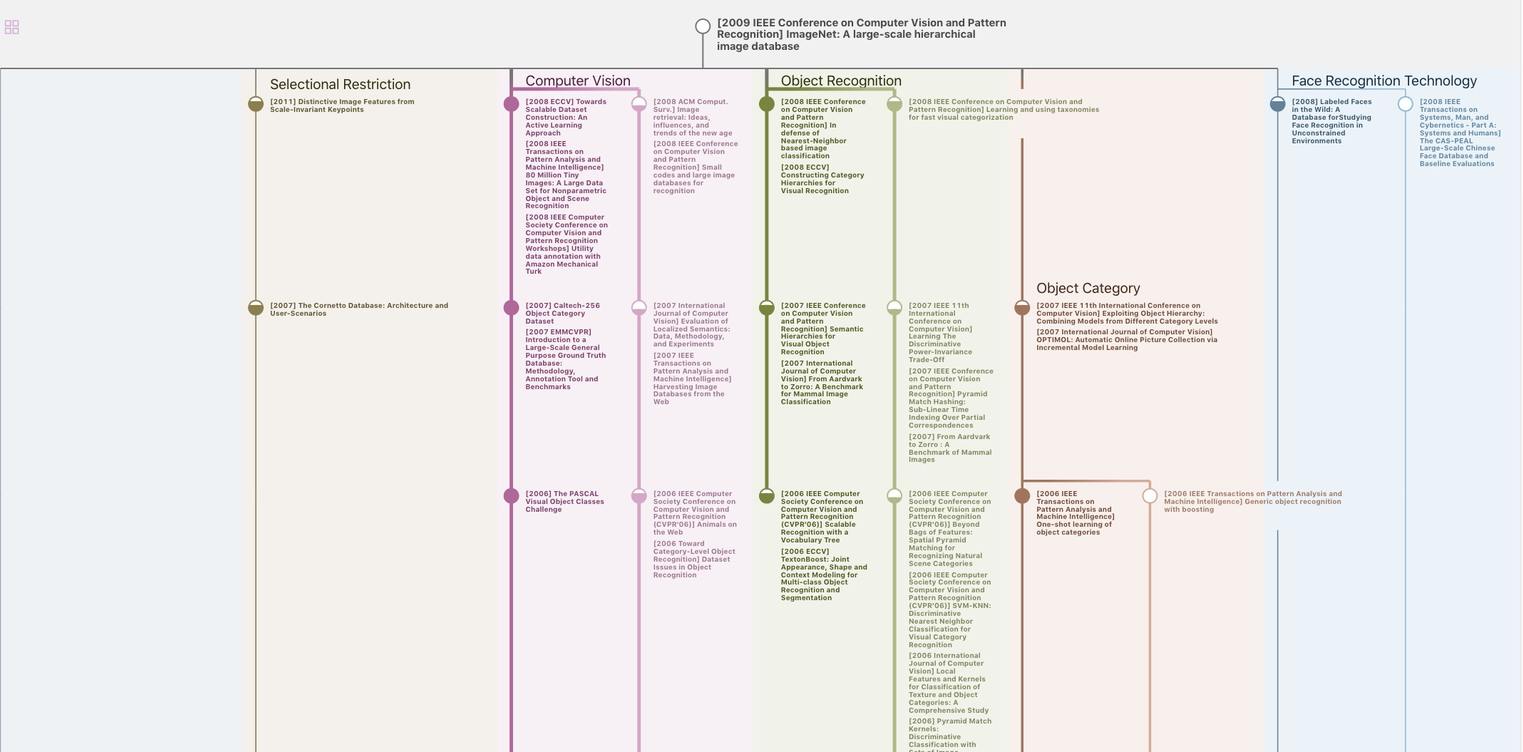
生成溯源树,研究论文发展脉络
Chat Paper
正在生成论文摘要