Aero-Engine Modeling and Control Method with Model-Based Deep Reinforcement Learning
AEROSPACE(2023)
摘要
Due to the strong representation ability and capability of learning from data measurements, deep reinforcement learning has emerged as a powerful control method, especially for nonlinear systems, such as the aero-engine control system. In this paper, a novel application of deep reinforcement learning (DRL) is presented for aero-engine control. In addition, transition dynamic characteristic information of the aero-engine is extracted from the replay buffer of deep reinforcement learning to train a neural-network dynamic prediction model for the aero-engine. In turn, the dynamic prediction model is used to improve the learning efficiency of reinforcement learning. The practical applicability of the proposed control system is demonstrated by the numerical simulations. Compared with the traditional control system, this novel aero-engine control system has faster response speed, stronger self-learning ability, and avoids the complicated manual parameter adjustment without sacrificing the control performance. Moreover, the dynamic prediction model has satisfactory prediction accuracy, and the model-based method can achieve higher learning efficiency than the model-free method.
更多查看译文
关键词
aero-engine control,reinforcement learning,neural network,nonlinear system
AI 理解论文
溯源树
样例
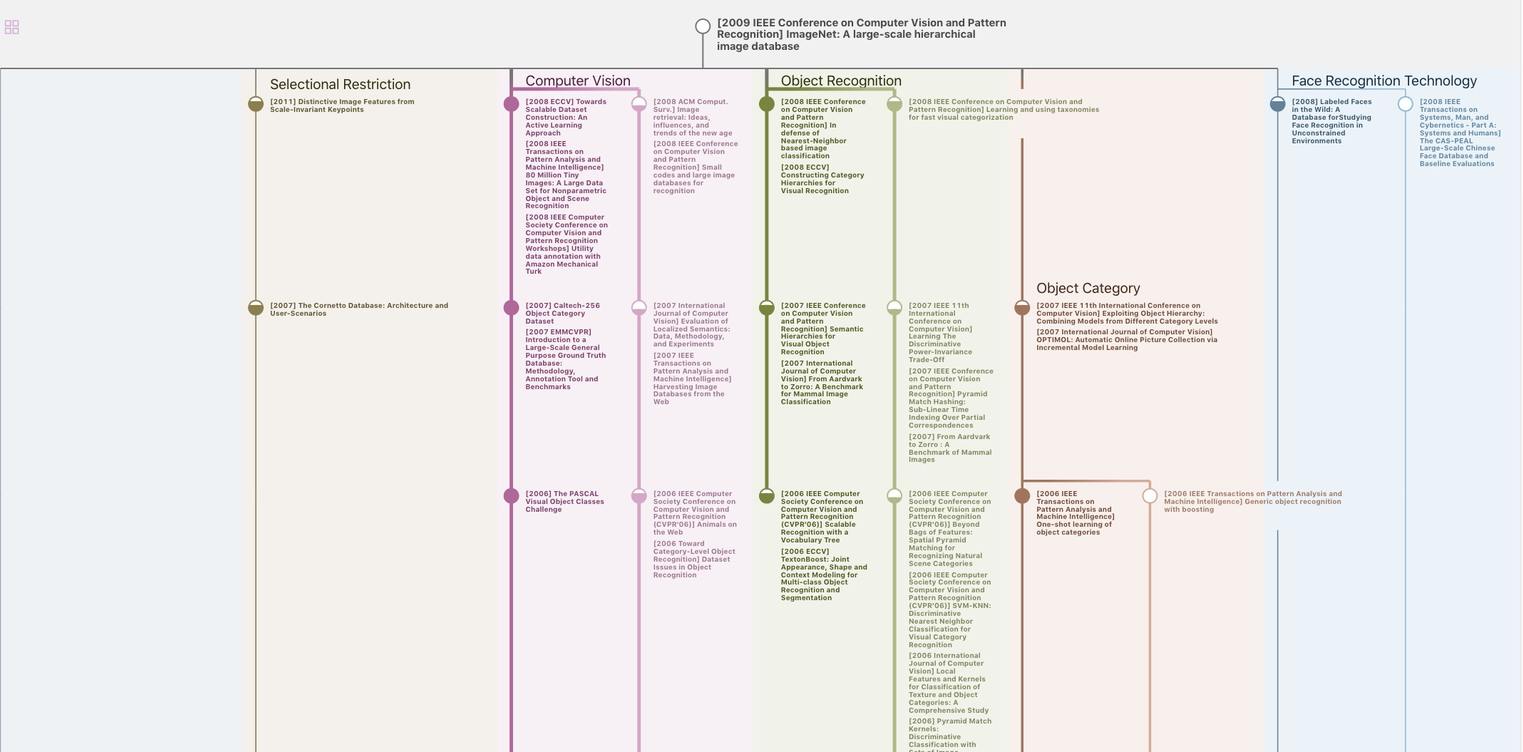
生成溯源树,研究论文发展脉络
Chat Paper
正在生成论文摘要