Improving Human Pose Estimation Based on Stacked Hourglass Network
NEURAL PROCESSING LETTERS(2023)
摘要
The performance of multi-person pose estimation has been greatly improved due to the rapid development of deep learning. However, the problems of self-occlusion, mutual occlusion and complex background not only have not been effectively solved. In order to further effectively solve these problems, in this paper, we design a novel Global and Local Content-aware Feature Boosting Network (GLCFBNet) that includes Intra-layer Feature Residual-like Module (IFRM), Input Feature Aggregation Module (IFAM), Spatial and Channel Feature Hourglass Attention Module (SCFHAM). We propose a novel IFRM that can expand receptive field of each convolution layer through aggregation feature. The IRAM can fully extract the edge information of the input image,and effectively solve the problem of negative background impact. The SCFHAM can accurately determine the location of the occluded keypoints, judge the global information of the reasonable keypoints, and extract the effective features for joint node positioning from the redundant feature information. We evaluate the effectiveness of our proposed method on the MSCOCO keypoint detection dataset, the MPII Human Pose dataset and the CrowdPose dataset.
更多查看译文
关键词
Human pose estimation,Keypoints occlusion,Complex background,Attention mechanisms
AI 理解论文
溯源树
样例
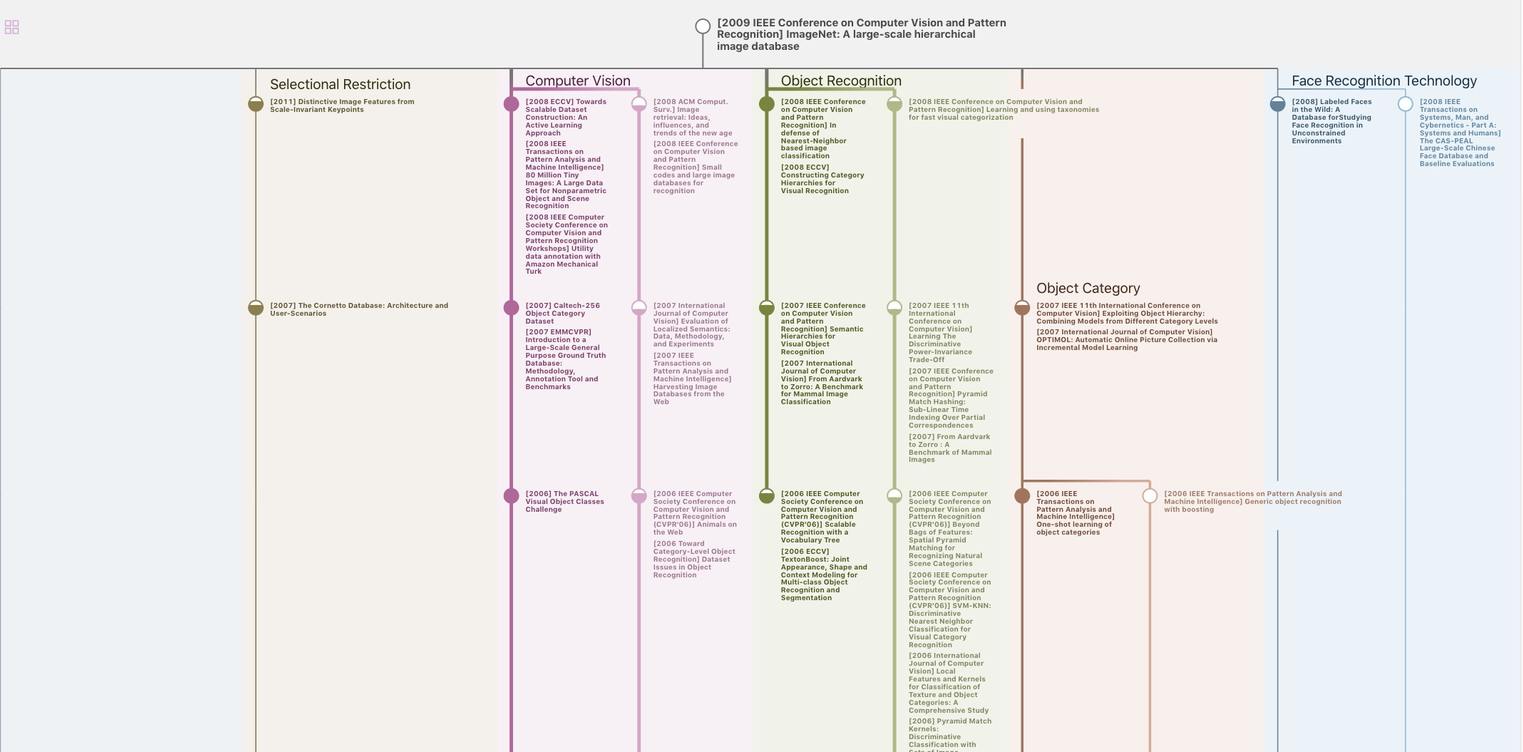
生成溯源树,研究论文发展脉络
Chat Paper
正在生成论文摘要