Deep reinforcement learning based scheduling strategy for federated learning in sensor-cloud systems
FUTURE GENERATION COMPUTER SYSTEMS-THE INTERNATIONAL JOURNAL OF ESCIENCE(2023)
摘要
Sensor-cloud systems (SCSs) aim to provide flexible configurable platforms for monitoring and control-ling the IoT-enabled applications. By integrating sensors, wireless networks and cloud for managing sensors, collecting data, and automating decision-making, the collected sensing data are typically used for machine learning purposes. With increasing emphasis in privacy protection, Federated Learning (FL) is widely adopted for enhancing privacy preservation. FL enables sharing of data for machine learning while preserving the privacy of the data owners. In SCSs, FL involves a large number of edge nodes in order to ensure a sufficient amount of data for model training. However, FL inevitably incurs prohibitive overheads if it simply gathers data from all the nodes, hence making it desirable to adopt some scheduling strategy so that data are collected only from a selected subset of nodes. This paper proposes a scheduling strategy based on deep reinforcement learning (DRL) for improving the performance and efficiency of FL in SCSs. The DRL environment, such as state space, action space, and reward function, is carefully designed. Proximal policy optimization is employed to train the DRL agent. Experimental results demonstrated that the proposed method outperforms other baselines on both independent and identically distributed (IID) and non-IID datasets.(c) 2023 Elsevier B.V. All rights reserved.
更多查看译文
关键词
Federated learning,Sensor-cloud systems,Scheduling strategy,Deep reinforcement learning,Wireless networks,Communication
AI 理解论文
溯源树
样例
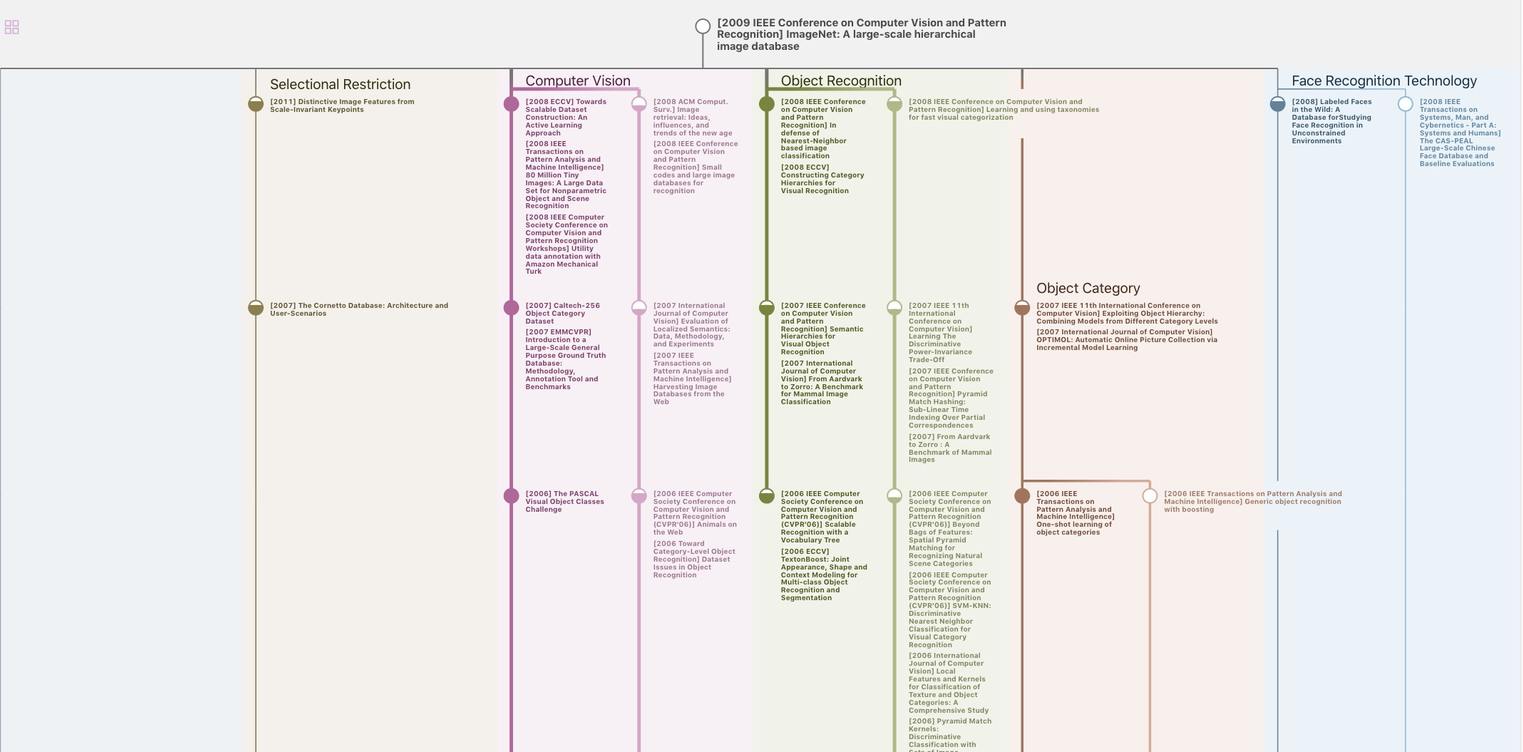
生成溯源树,研究论文发展脉络
Chat Paper
正在生成论文摘要