A new interest extraction method based on multi-head attention mechanism for CTR prediction
KNOWLEDGE AND INFORMATION SYSTEMS(2023)
摘要
Click-through rate (CTR) prediction plays a vital role in recommendation systems. Most models pay little attention to the relationship between target items in the user behavior sequence. The attention units used in these models cannot fully capture the context information, which can be used to reflect the variations of user interests. To address these problems, we propose a new model named interest extraction method based on multi-head attention mechanism (IEN) for CTR prediction. Specifically, we design an interest extraction module, which consists of two sub-modules: the item representation module (IRM) and the context–item interaction module (CIM). In IRM, we learn the relationship between target items in the user behavior sequence by a multi-head attention mechanism. Then, the user representation is gained by integrating the refined item representation and position information. At last, the correlation between the user and the target item is used to reflect user interests. In CIM, the context information has valuable temporal features which can reflect the variations of user interests. Therefore, user interests can be further acquired through the feature interaction between the context and the target item. After that, the learned relevance and the feature interaction are fed to the multi-layer perceptron (MLP) for prediction. Besides, experiments on four Amazon datasets were conducted to evaluate the effectiveness of our method in capturing user interests. The experimental results show that our proposed method outperforms state-of-the-art methods in terms of AUC and RI in the CTR prediction task.
更多查看译文
关键词
Recommendation system,Multi-head attention,Feature interaction,Click-through rate prediction
AI 理解论文
溯源树
样例
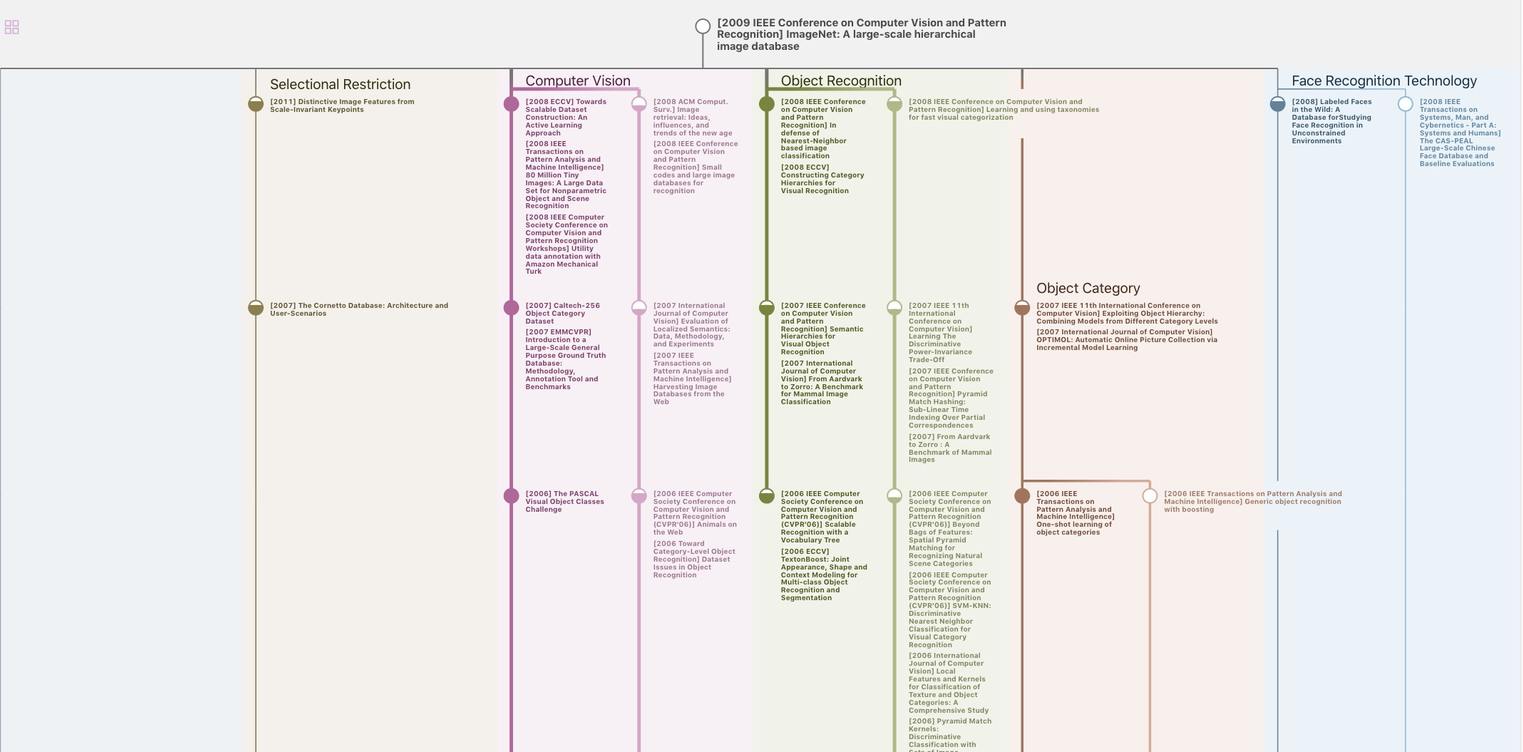
生成溯源树,研究论文发展脉络
Chat Paper
正在生成论文摘要