Understanding Residents' Behavior for Smart City Management by Sequential and Periodic Pattern Mining
IEEE TRANSACTIONS ON COMPUTATIONAL SOCIAL SYSTEMS(2024)
摘要
Understanding the residents' routine and repetitive behavior patterns is important for city planners and strategic partners to enact appropriate city management policies. However, the existing approaches reported in smart city management areas often rely on clustering or machine learning, which are ineffective in capturing such behavioral patterns. Aiming to address this research gap, this article proposes an analytical framework, adopting sequential and periodic pattern mining techniques, to effectively discover residents' routine behavior patterns. The effectiveness of the proposed framework is demonstrated in a case study of American public behavior based on a large-scale venue check-in dataset. The dataset was collected in 2020 (during the global pandemic due to COVID-19) and contains 257 561 check-in data of 3995 residents. The findings uncovered interesting behavioral patterns and venue visit information of residents in the United States during the pandemic, which could help the public and crisis management in cities.
更多查看译文
关键词
Behavioral sciences,Data mining,Smart cities,Data analysis,Soft sensors,Pandemics,Data collection,Periodic pattern mining,sequential pattern mining,smart city,social media analytics
AI 理解论文
溯源树
样例
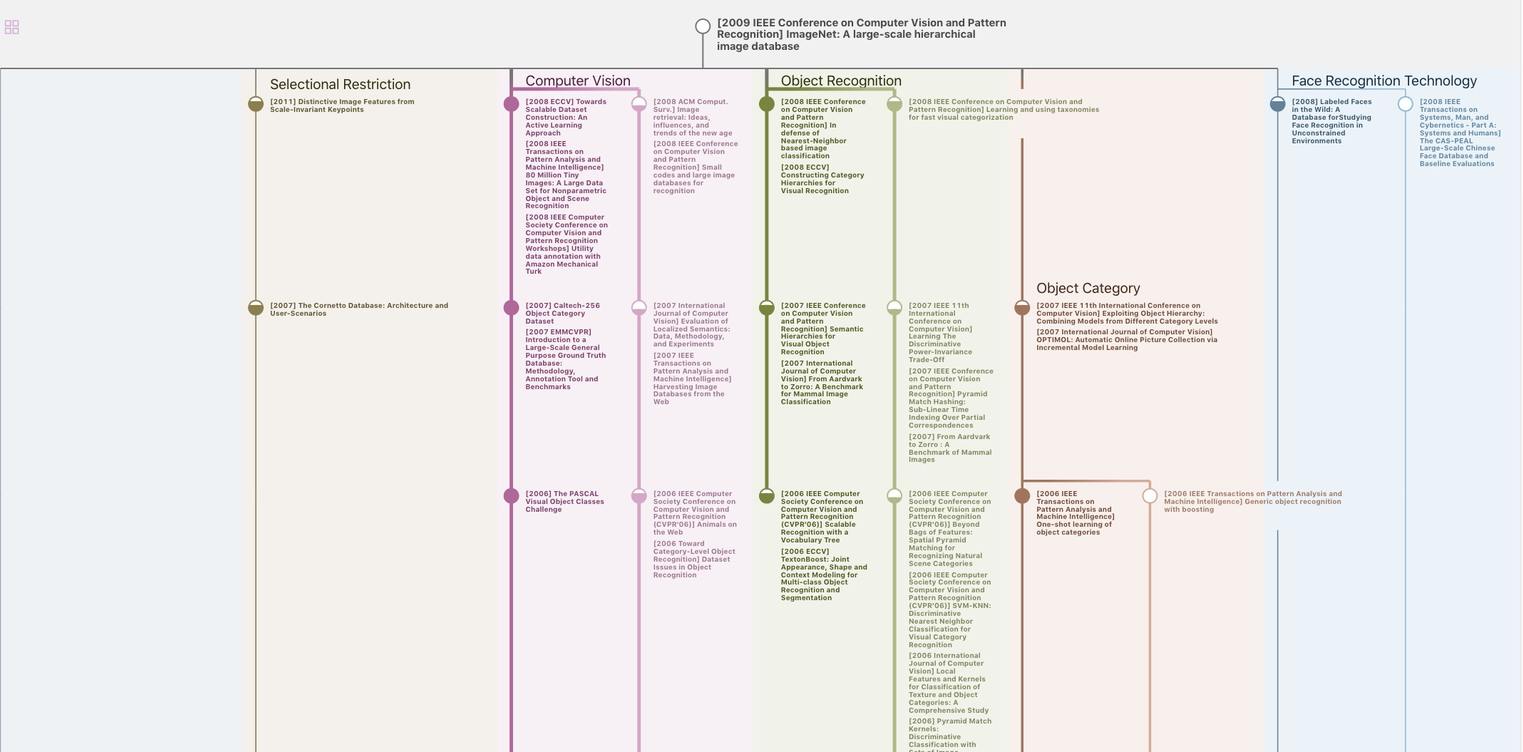
生成溯源树,研究论文发展脉络
Chat Paper
正在生成论文摘要