CURE-Net: A Cascaded Deep Network for Underwater Image Enhancement
IEEE JOURNAL OF OCEANIC ENGINEERING(2024)
摘要
Underwater imaging is usually degraded by low contrast or color cast due to light absorption and scattering. This fact limits the accuracy of object recognition in underwater and marine environments. In this article, to address this issue, we propose a CURE-Net that progressively improves degraded underwater images in a coarse-to-fine way. To be specific, our CURE-Net consists of three cascaded subnetworks. The first two subnetworks use attention and gate fusion to learn multiscale contextual information, whereas the third subnetwork preserves fine spatial details. A detail enhancement block and a supervised recovery block are deployed between subnetworks to further improve the recovery of colors and details. Our proposed CURE-Net is extensively evaluated on popular data sets and achieves state-of-the-art performance. Compared with its peers, our method is also more friendly to object recognition-underwater objects are more easily recognized after being processed by our method.
更多查看译文
关键词
Image color analysis,Task analysis,Logic gates,Colored noise,Visualization,Image restoration,Image enhancement,Deep learning (DL),image denoising,image processing,underwater image enhancement (UIE)
AI 理解论文
溯源树
样例
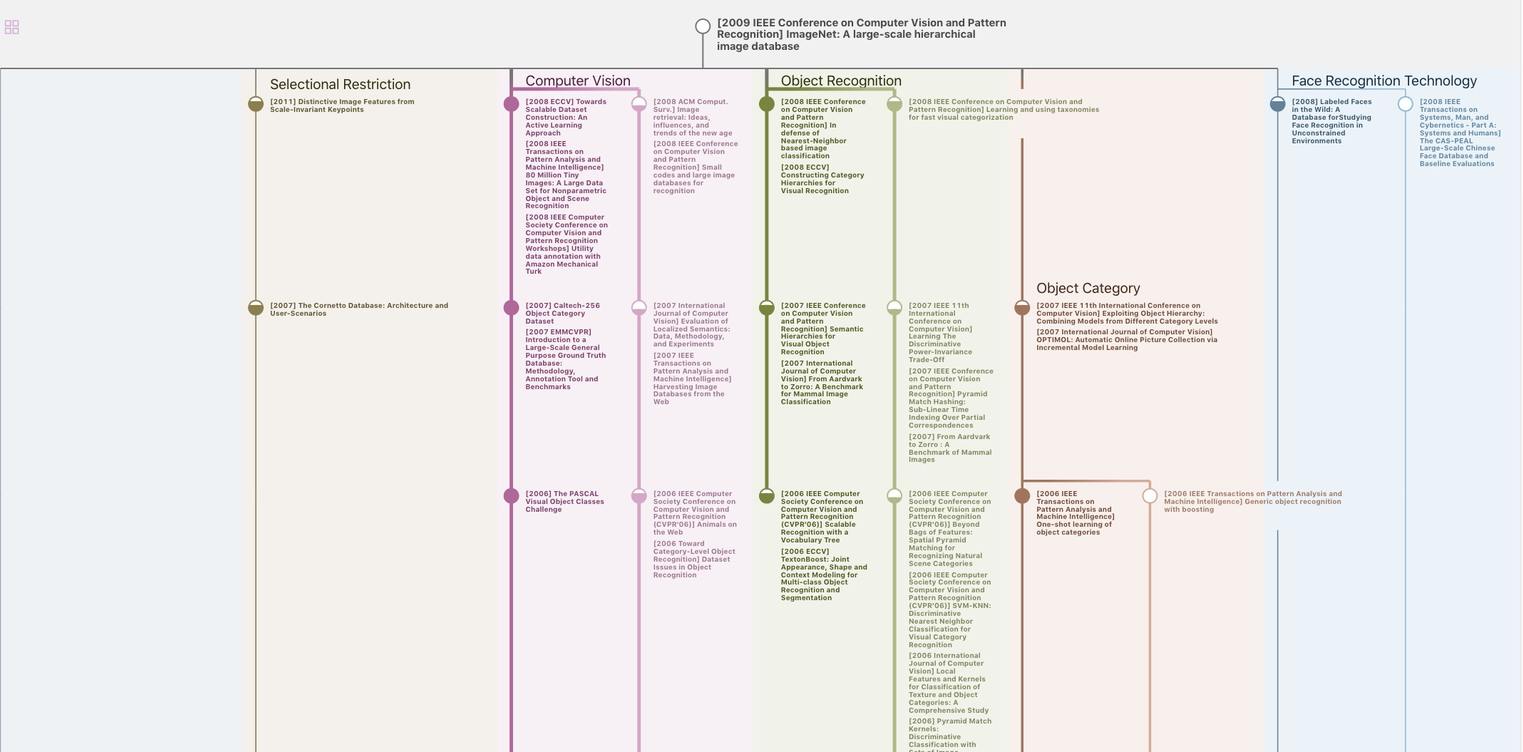
生成溯源树,研究论文发展脉络
Chat Paper
正在生成论文摘要