Projecting live fuel moisture content via deep learning
INTERNATIONAL JOURNAL OF WILDLAND FIRE(2023)
摘要
Background. Live fuel moisture content (LFMC) is a key environmental indicator used to monitor for high wildfire risk conditions. Many statistical models have been proposed to predict LFMC from remotely sensed data; however, almost all these estimate current LFMC (nowcasting models). Accurate modelling of LFMC in advance (projection models) would provide wildfire managers with more timely information for assessing and preparing for wildfire risk. Aims. The aim of this study was to investigate the potential for deep learning models to predict LFMC across the continental United States 3 months in advance. Method. Temporal convolutional networks were trained and evaluated using a large database of field measured samples, as well as year-long time series of MODerate resolution Imaging Spectroradiometer (MODIS) reflectance data and Parameter-elevation Relationships on Independent Slopes Model (PRISM) meteorological data. Key results. The proposed 3-month projection model achieved an accuracy (root mean squared error (RMSE) 27.52%; R-2 0.47) close to that of the nowcasting model (RMSE 26.52%; R-2 0.51). Conclusions. The study is the first to predict LFMC with a 3-month lead-time, demonstrating the potential for deep learning models to make reliable LFMC projections. Implications. These findings are beneficial for wildfire management and risk assessment, showing proof-of-concept for providing advance information useful to help mitigate the effect of catastrophic wildfires.
更多查看译文
关键词
convolutional neural network,deep learning ensembles,fire danger,live fuel moisture content,meteorological data,MODIS,remote sensing,time series analysis
AI 理解论文
溯源树
样例
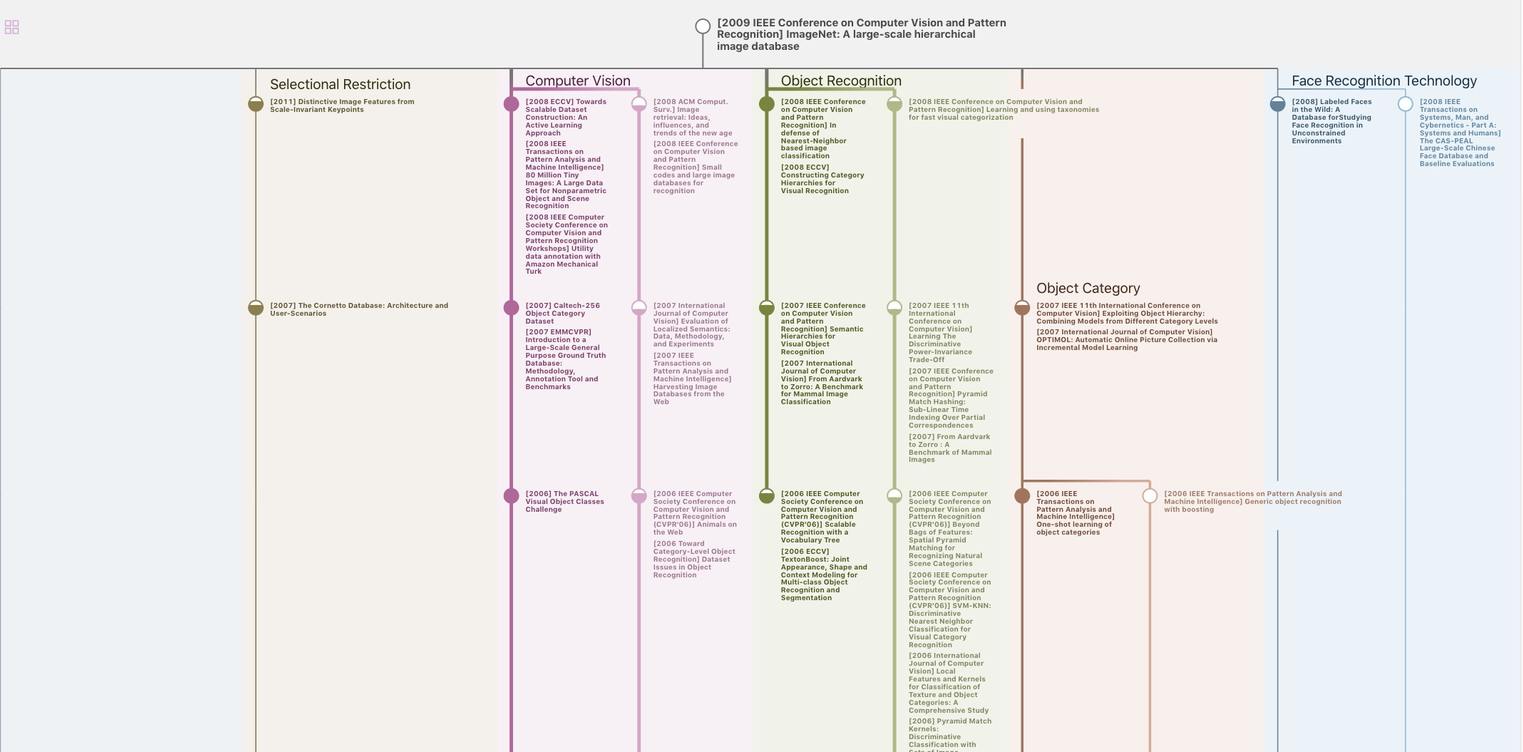
生成溯源树,研究论文发展脉络
Chat Paper
正在生成论文摘要