Thermal fault prognosis of lithium-ion batteries in real-world electric vehicles using self-attention mechanism networks
APPLIED THERMAL ENGINEERING(2023)
摘要
Battery temperature is directly related to various thermal faults and thermal runaway of a battery system in electric vehicles. Its prediction is critical to the safety and durability of the battery system. This paper presents a novel clustering-based data partitioning method and a partitioned all-season coverage model established by the spiral self-attention neural network to perform real-time multi-forward-step temperature prediction for battery systems. A yearlong real-world operational dataset of an electric taxi is deployed. The proposed series -temperature method can generate a generic and non-interfering model for all temperature probes. The results demonstrate that the partitioned training model has excellent online prediction performance for non -differentiated real-world vehicle operation in all climates. The prognosis feasibility and reliability of the model for various temperature anomalies are also validated, providing a reliable tool for battery safety by determining the advent of thermal faults and mitigating the risk of thermal runaway. Furthermore, the flexible and adjustable predicted horizons could provide sufficient processing time for predicting and preventing battery anomaly/fault. This method's superiority, stability, flexibility, and robustness are verified under different hyperparameters and operating patterns.
更多查看译文
关键词
Electric vehicles,Battery systems,Self -attention mechanism,Temperature prediction,Fault prognosis
AI 理解论文
溯源树
样例
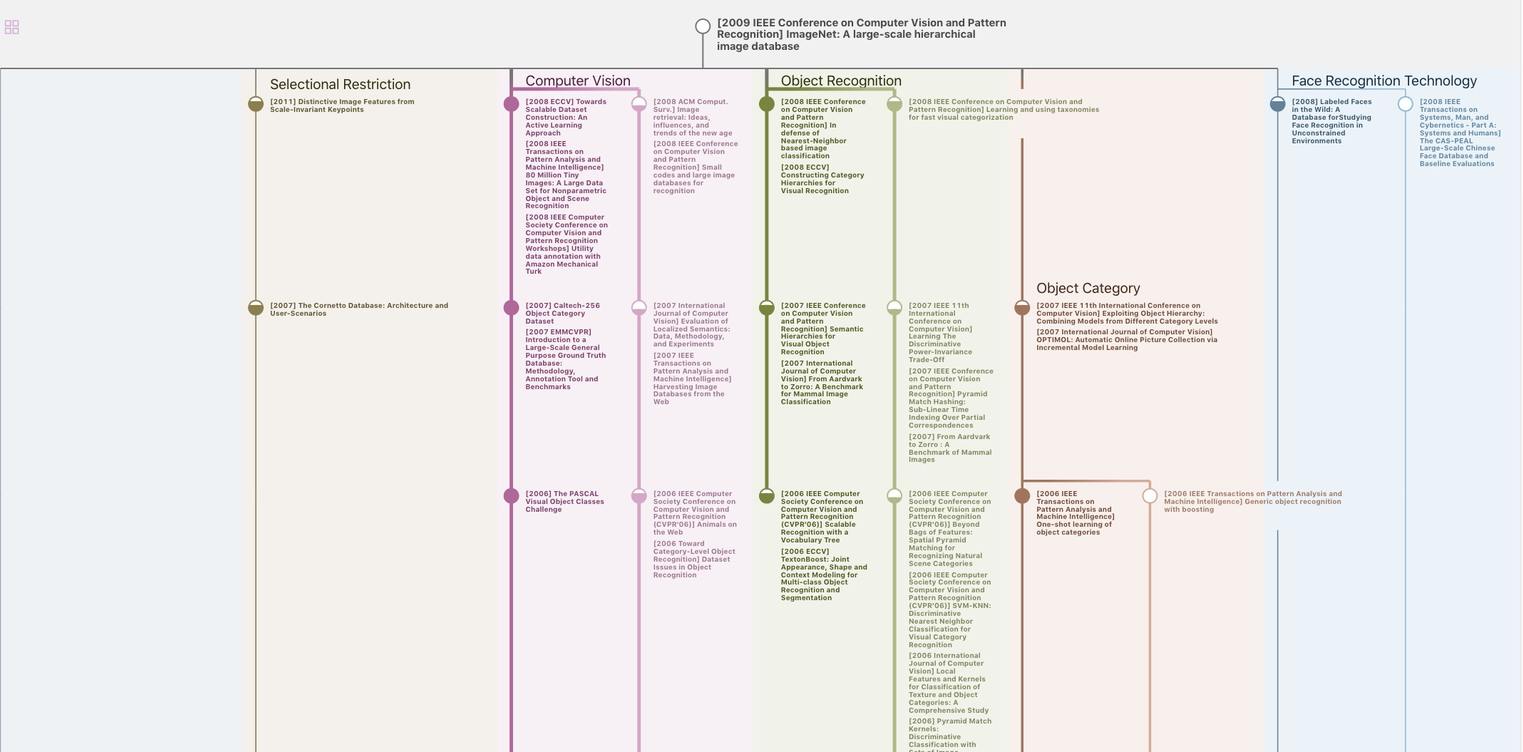
生成溯源树,研究论文发展脉络
Chat Paper
正在生成论文摘要