Physics-informed neural networks for building thermal modeling and demand response control
BUILDING AND ENVIRONMENT(2023)
摘要
Buildings energy consumption constitutes over 40% of the total primary energy consumption, and buildings can provide potential energy flexibility for the grid. In the grid with high penetration of renewable energy, a controloriented model with accurate prediction and prompt response is crucial for better load management. However, developing such a model is still a big challenge for grid-integrated buildings due to the limitations of current modeling approaches. Physics-based models commonly represent a specific building while customization for each building remains a daunting task; meanwhile, data-driven models, though easier to develop, require a large amount of data and are not well generalized for other buildings. Thus, we propose a novel model based on a physics-informed neural network dubbed as PhysCon, which combines the interpretable ability of physical laws and the expressive power of neural networks for control-oriented demand response of grid-integrated buildings. Specifically, a Resistance-Capacitance (2R2C) thermal model is adopted to express the knowledge of building physics, and a full-connected neural network is developed to learn the information from actual data. We demonstrate the prediction performance of PhysCon using actual building physics parameters and on-site measured data from an experimental platform that contains two detachable rooms. Two different internal thermal mass scenarios, namely light and heavy mass, were designed to demonstrate the power of PhysCon for buildings with different levels of demand response potentials. The results show that PhysCon outperforms the purely data-driven model in predicting the room temperature and thermal load demand for both light and heavy buildings. Moreover, building thermal mass is an efficient and free demand response resource for grid-integrated buildings. The proposed PhysCon model can be conveniently and efficiently deployed in the energy management system of buildings, owing to its simplicity and generalization.
更多查看译文
关键词
Physics -informed neural networks,Control -oriented modeling,Grid -integrated buildings,Demand response
AI 理解论文
溯源树
样例
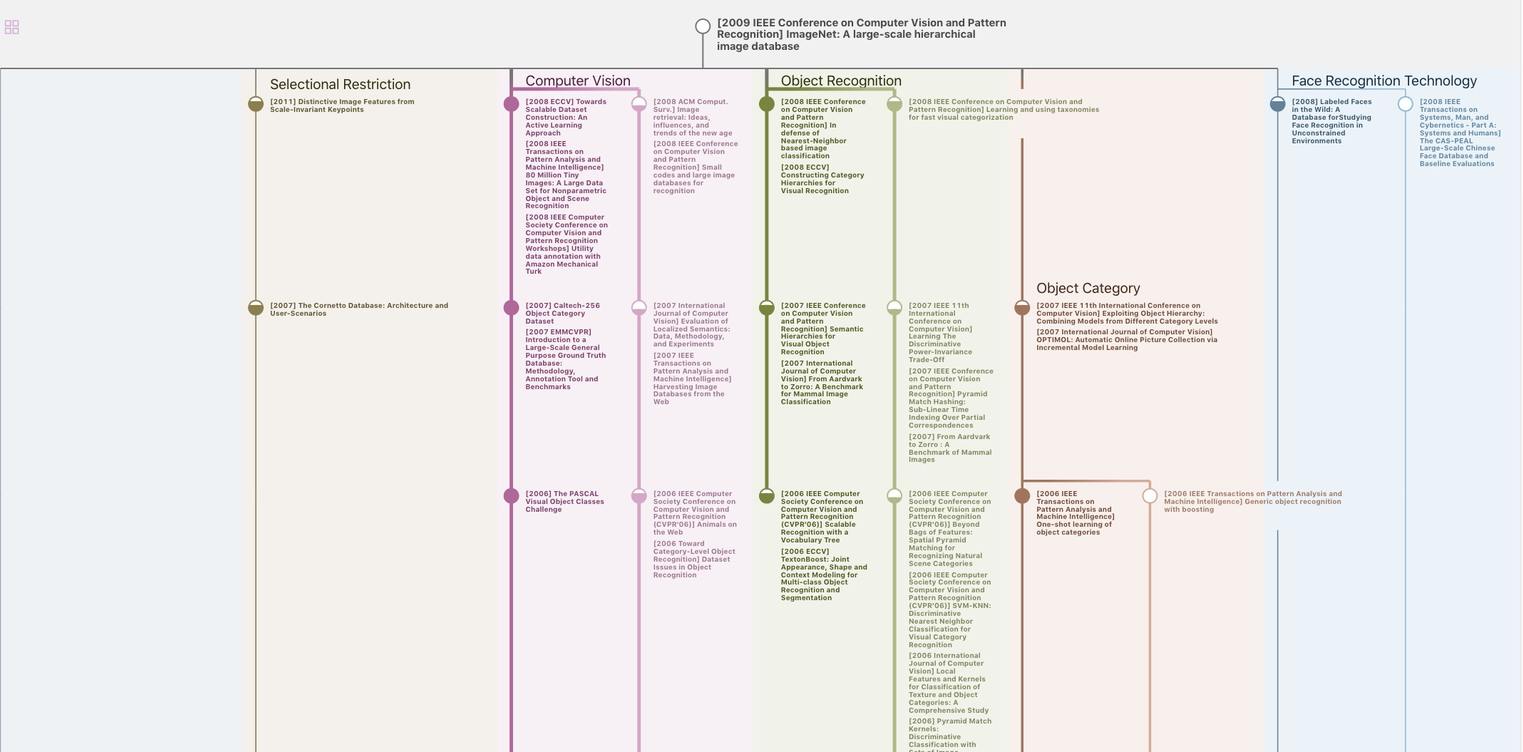
生成溯源树,研究论文发展脉络
Chat Paper
正在生成论文摘要