Inference of population genetics parameters using discriminator neural networks: an adversarial Monte Carlo approach
bioRxiv (Cold Spring Harbor Laboratory)(2023)
摘要
Accurately estimating biological variables of interest, such as parameters of demographic models, is a key problem in evolutionary genetics. Likelihood-based and likelihood-free methods both typically use only limited genetic information, such as carefully chosen summary statistics. Deep convolutional neural networks (CNNs) trained on genotype matrices can incorporate a great deal more information, and have been shown to have high accuracy for inferring parameters such as recombination rates and population sizes, when evaluated using simulations. However these methods are typically framed as regression or classification problems, and it is not straightforward to ensure that the training data adequately model the empirical data on which they are subsequently applied. It has recently been shown that generative adversarial networks (GANs) can be used to iteratively tune parameter values until simulations match a given target dataset. Here, we investigate an adversarial architecture for discriminator-based inference, which iteratively improves the sampling distribution for training the discriminator CNN via Monte Carlo density estimation. We show that this method produces parameter estimates with excellent agreement to simulated data. We developed dinf, a modular Python package for discriminator-based inference that incorporates this method, and is available from .
### Competing Interest Statement
The authors have declared no competing interest.
更多查看译文
关键词
population genetics parameters,discriminator,inference
AI 理解论文
溯源树
样例
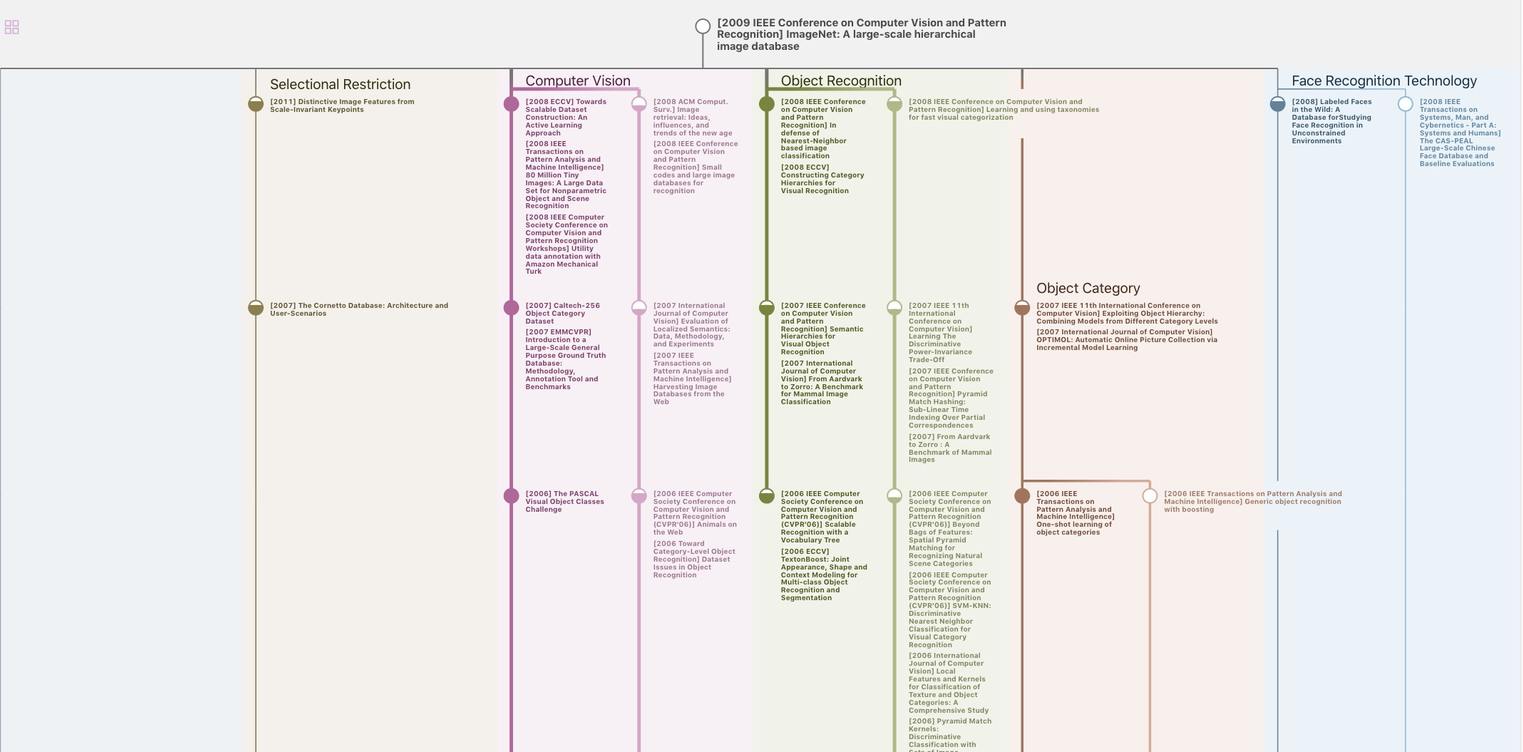
生成溯源树,研究论文发展脉络
Chat Paper
正在生成论文摘要