Enhancing Clinical Predictive Modeling through Model Complexity-Driven Class Proportion Tuning for Class Imbalanced Data: An Empirical Study on Opioid Overdose Prediction
CoRR(2023)
摘要
Class imbalance problems widely exist in the medical field and heavily deteriorates performance of clinical predictive models. Most techniques to alleviate the problem rebalance class proportions and they predominantly assume the rebalanced proportions should be a function of the original data and oblivious to the model one uses. This work challenges this prevailing assumption and proposes that links the optimal class proportions to the model complexity, thereby tuning the class proportions per model. Our experiments on the opioid overdose prediction problem highlight the performance gain of tuning class proportions. Rigorous regression analysis also confirms the advantages of the theoretical framework proposed and the statistically significant correlation between the hyperparameters controlling the model complexity and the optimal class proportions.
更多查看译文
AI 理解论文
溯源树
样例
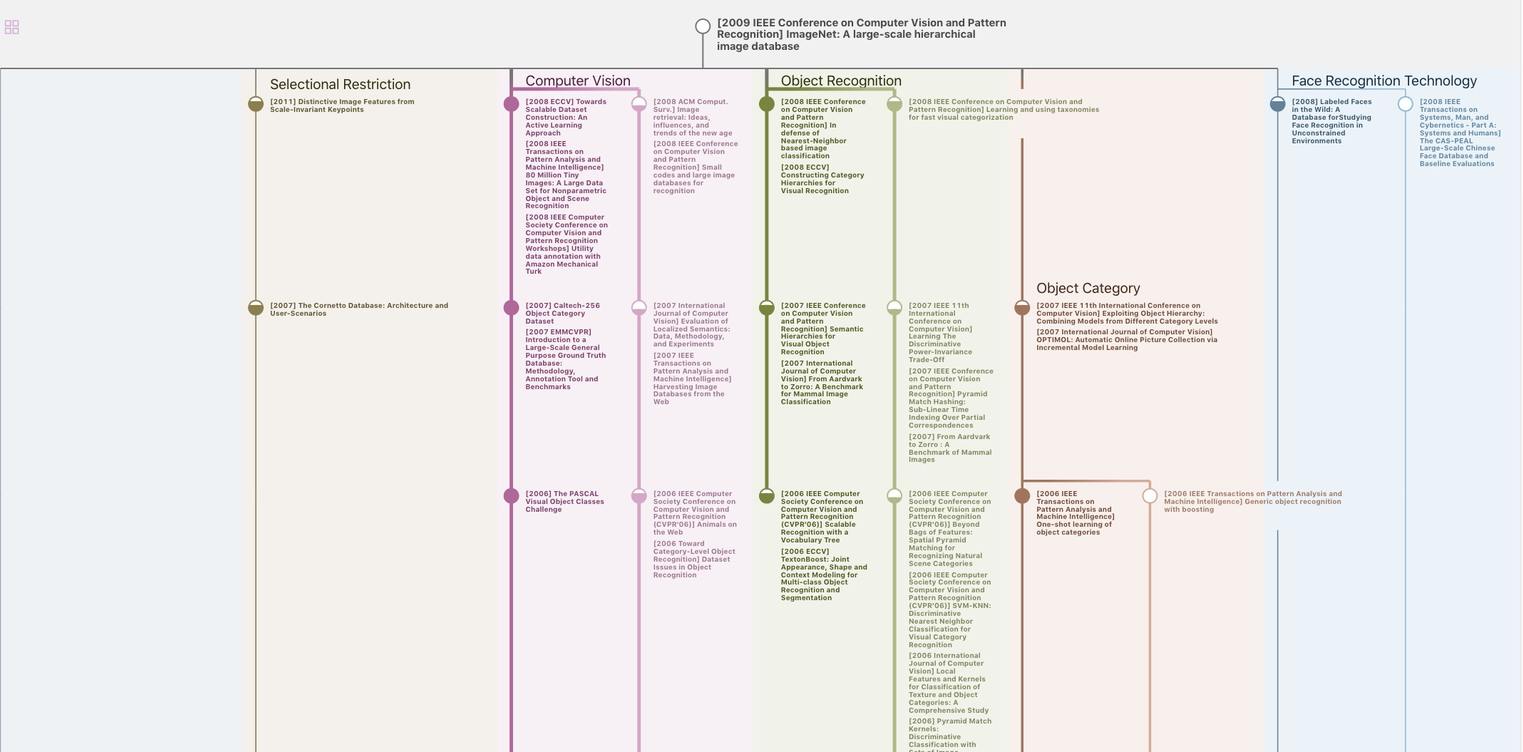
生成溯源树,研究论文发展脉络
Chat Paper
正在生成论文摘要