Safe Deep RL for Intraoperative Planning of Pedicle Screw Placement
CoRR(2023)
摘要
Spinal fusion surgery requires highly accurate implantation of pedicle screw implants, which must be conducted in critical proximity to vital structures with a limited view of anatomy. Robotic surgery systems have been proposed to improve placement accuracy, however, state-of-the-art systems suffer from the limitations of open-loop approaches, as they follow traditional concepts of preoperative planning and intraoperative registration, without real-time recalculation of the surgical plan. In this paper, we propose an intraoperative planning approach for robotic spine surgery that leverages real-time observation for drill path planning based on Safe Deep Reinforcement Learning (DRL). The main contributions of our method are (1) the capability to guarantee safe actions by introducing an uncertainty-aware distance-based safety filter; and (2) the ability to compensate for incomplete intraoperative anatomical information, by encoding a-priori knowledge about anatomical structures with a network pre-trained on high-fidelity anatomical models. Planning quality was assessed by quantitative comparison with the gold standard (GS) drill planning. In experiments with 5 models derived from real magnetic resonance imaging (MRI) data, our approach was capable of achieving 90% bone penetration with respect to the GS while satisfying safety requirements, even under observation and motion uncertainty. To the best of our knowledge, our approach is the first safe DRL approach focusing on orthopedic surgeries.
更多查看译文
关键词
intraoperative planning
AI 理解论文
溯源树
样例
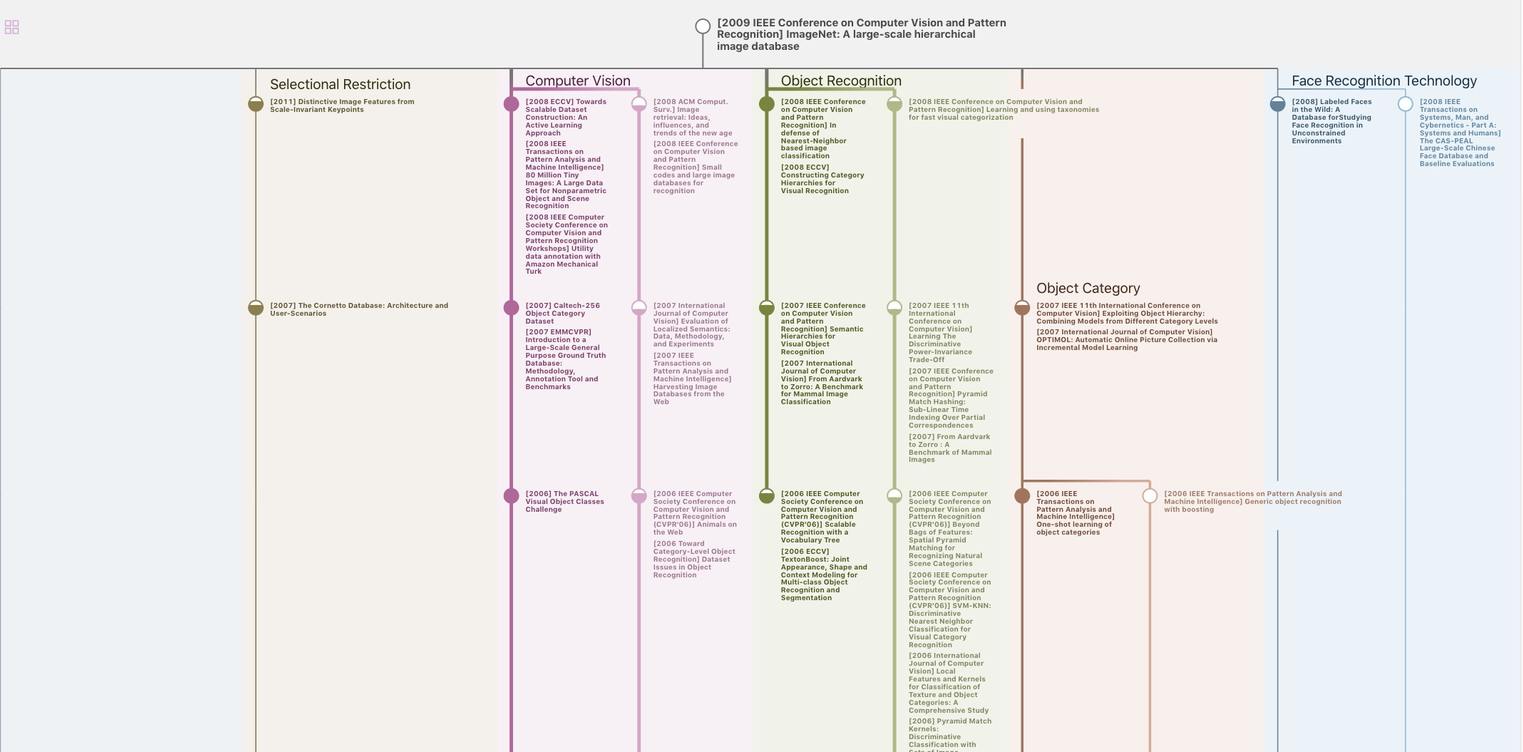
生成溯源树,研究论文发展脉络
Chat Paper
正在生成论文摘要