Latent Interactive A2C for Improved RL in Open Many-Agent Systems
CoRR(2023)
摘要
There is a prevalence of multiagent reinforcement learning (MARL) methods that engage in centralized training. But, these methods involve obtaining various types of information from the other agents, which may not be feasible in competitive or adversarial settings. A recent method, the interactive advantage actor critic (IA2C), engages in decentralized training coupled with decentralized execution, aiming to predict the other agents' actions from possibly noisy observations. In this paper, we present the latent IA2C that utilizes an encoder-decoder architecture to learn a latent representation of the hidden state and other agents' actions. Our experiments in two domains -- each populated by many agents -- reveal that the latent IA2C significantly improves sample efficiency by reducing variance and converging faster. Additionally, we introduce open versions of these domains where the agent population may change over time, and evaluate on these instances as well.
更多查看译文
关键词
improved rl,systems,latent,many-agent
AI 理解论文
溯源树
样例
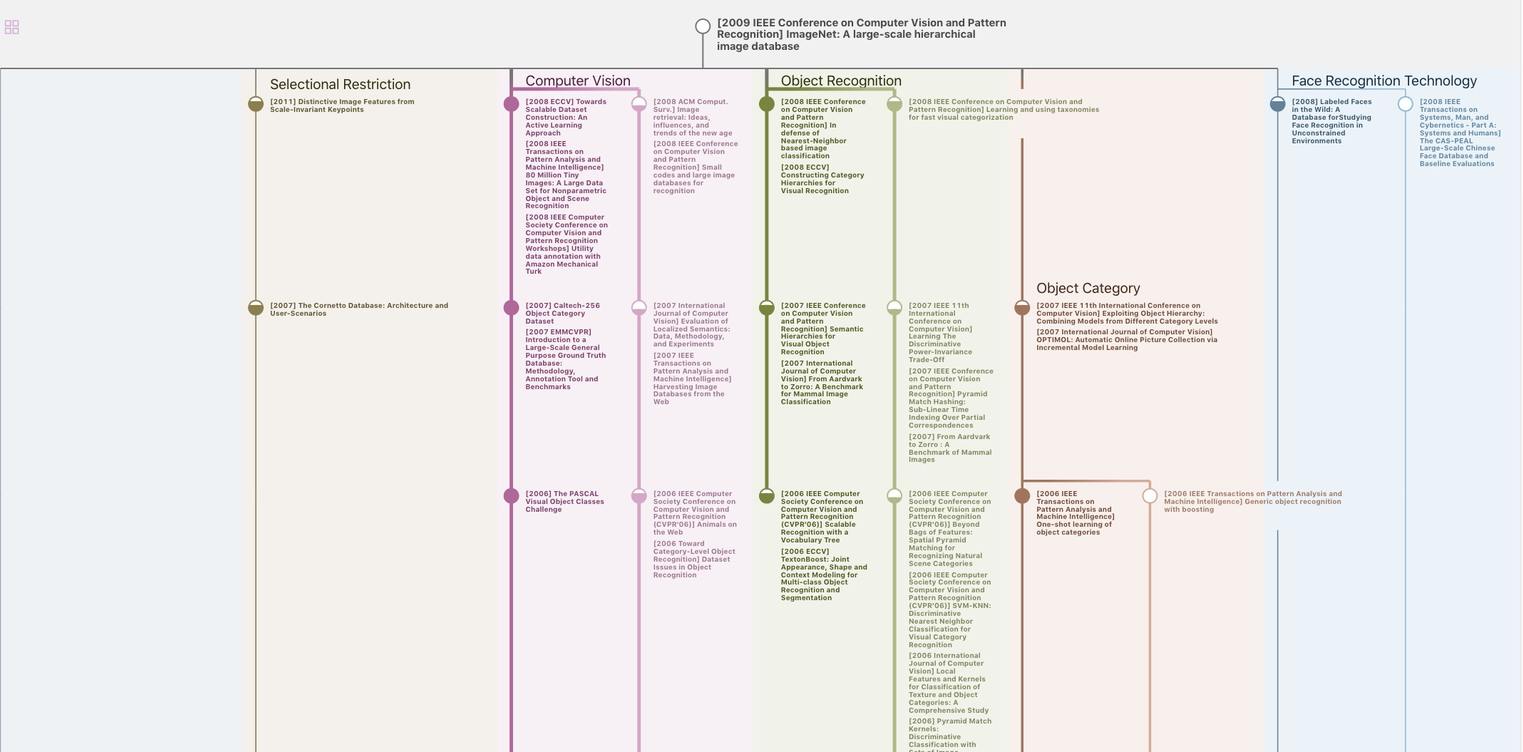
生成溯源树,研究论文发展脉络
Chat Paper
正在生成论文摘要