Data-Driven $H_{}$ Optimal Output Feedback Control for Linear Discrete-Time Systems Based on Off-Policy Q-Learning
IEEE TRANSACTIONS ON NEURAL NETWORKS AND LEARNING SYSTEMS(2023)
摘要
This article develops two novel output feedback (OPFB) Q-learning algorithms, on-policy Q-learning and off-policy Q-learning, to solve $H_{infinity}$ static OPFB control problem of linear discrete-time (DT) systems. The primary contribution of the proposed algorithms lies in a newly developed OPFB control algorithm form for completely unknown systems. Under the premise of satisfying disturbance attenuation conditions, the conditions for the existence of the optimal OPFB solution are given. The convergence of the proposed Q-learning methods, and the difference and equivalence of two algorithms are rigorously proven. Moreover, considering the effects brought by probing noise for the persistence of excitation (PE), the proposed off-policy Q-learning method has the advantage of being immune to probing noise and avoiding biasedness of solution. Simulation results are presented to verify the effectiveness of the proposed approaches.
更多查看译文
关键词
Heuristic algorithms,Optimal control,Transmission line matrix methods,Process control,Performance analysis,Output feedback,Games,H∞,control,off-policy Q-learning,Q-learning,static output feedback (OPFB),zero-sum game
AI 理解论文
溯源树
样例
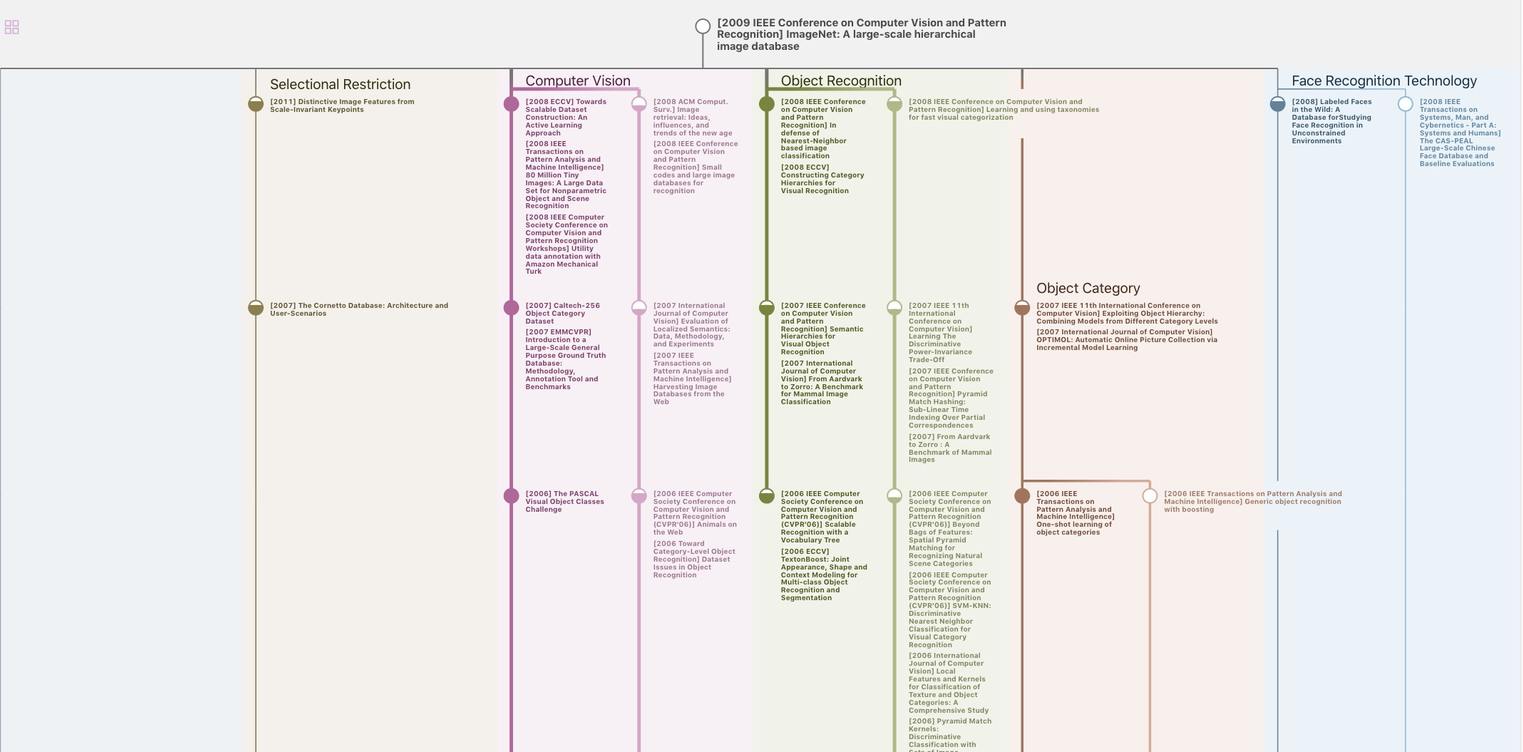
生成溯源树,研究论文发展脉络
Chat Paper
正在生成论文摘要