Target-Side Augmentation for Document-Level Machine Translation
conf_acl(2023)
摘要
Document-level machine translation faces the challenge of data sparsity due to its long input length and a small amount of training data, increasing the risk of learning spurious patterns. To address this challenge, we propose a target-side augmentation method, introducing a data augmentation (DA) model to generate many potential translations for each source document. Learning on these wider range translations, an MT model can learn a smoothed distribution, thereby reducing the risk of data sparsity. We demonstrate that the DA model, which estimates the posterior distribution, largely improves the MT performance, outperforming the previous best system by 2.30 s-BLEU on News and achieving new state-of-the-art on News and Europarl benchmarks. Our code is available at \url{https://github.com/baoguangsheng/target-side-augmentation}.
更多查看译文
关键词
translation,target-side,document-level
AI 理解论文
溯源树
样例
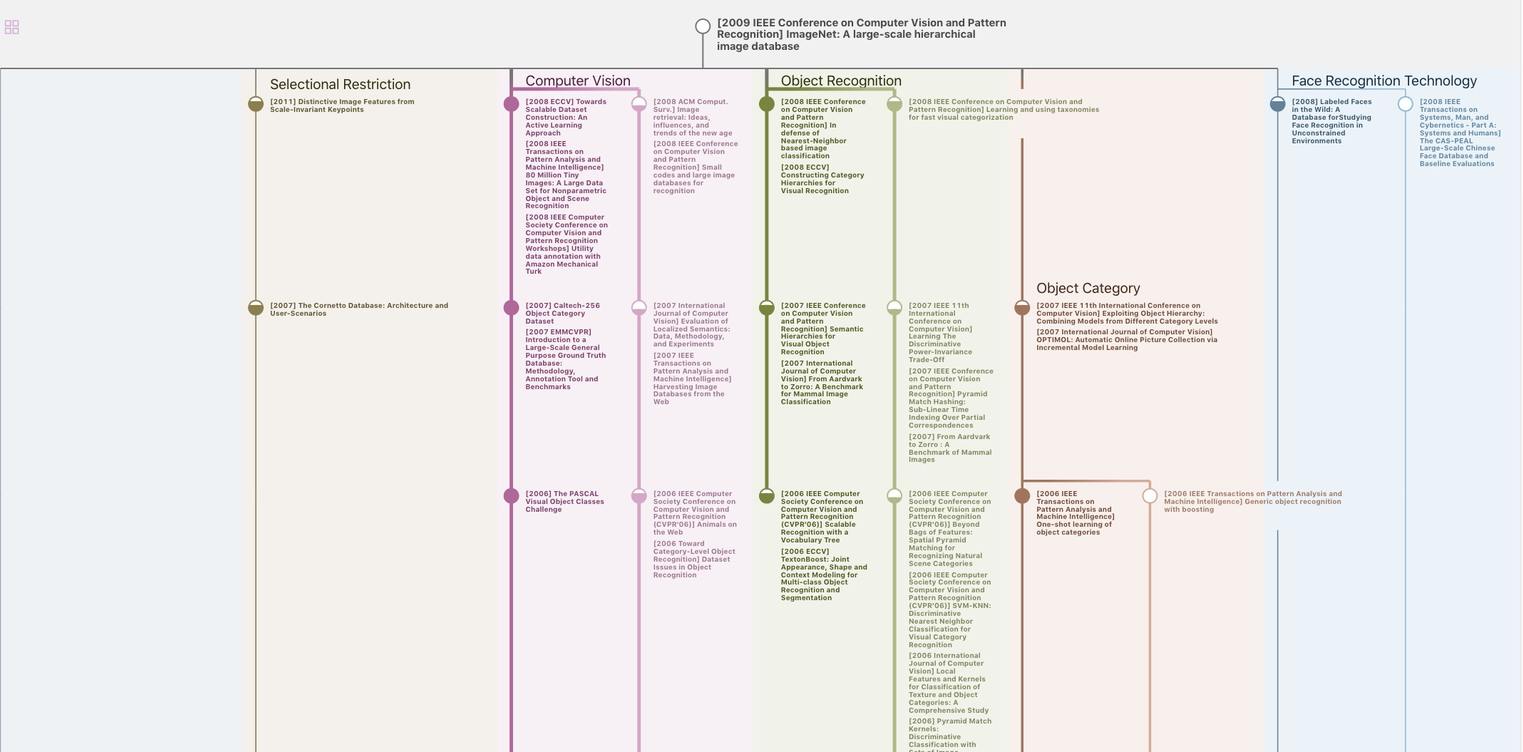
生成溯源树,研究论文发展脉络
Chat Paper
正在生成论文摘要