Improved Techniques for Maximum Likelihood Estimation for Diffusion ODEs
ICML 2023(2023)
摘要
Diffusion models have exhibited excellent performance in various domains. The probability flow ordinary differential equation (ODE) of diffusion models (i.e., diffusion ODEs) is a particular case of continuous normalizing flows (CNFs), which enables deterministic inference and exact likelihood evaluation. However, the likelihood estimation results by diffusion ODEs are still far from those of the state-of-the-art likelihood-based generative models. In this work, we propose several improved techniques for maximum likelihood estimation for diffusion ODEs, including both training and evaluation perspectives. For training, we propose velocity parameterization and explore variance reduction techniques for faster convergence. We also derive an error-bounded high-order flow matching objective for finetuning, which improves the ODE likelihood and smooths its trajectory. For evaluation, we propose a novel training-free truncated-normal dequantization to fill the training-evaluation gap commonly existing in diffusion ODEs. Building upon these techniques, we achieve state-of-the-art likelihood estimation results on image datasets (2.56 on CIFAR-10, 3.43 on ImageNet-32) without variational dequantization or data augmentation.
更多查看译文
关键词
diffusion odes,maximum likelihood estimation
AI 理解论文
溯源树
样例
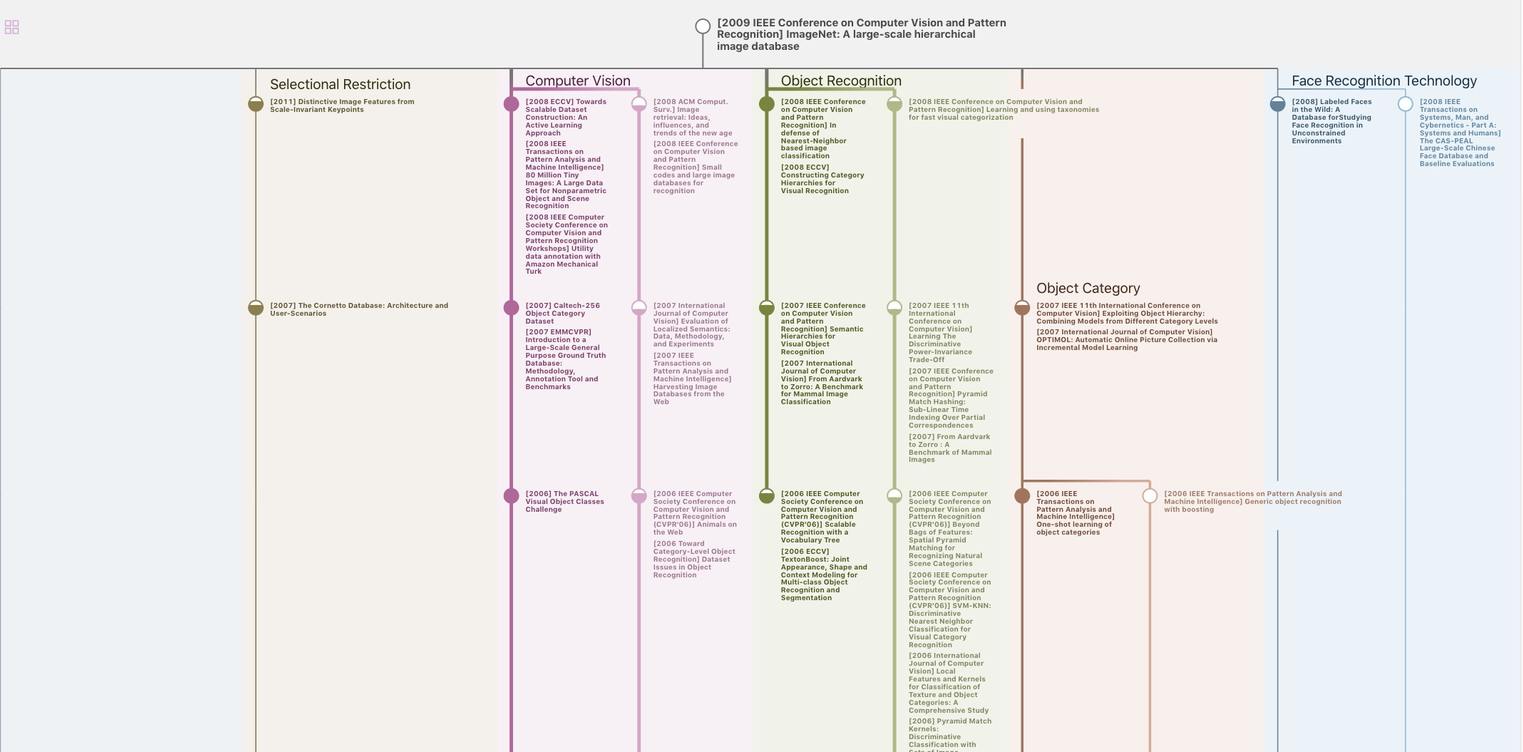
生成溯源树,研究论文发展脉络
Chat Paper
正在生成论文摘要