Data-Free Learning of Reduced-Order Kinematics
PROCEEDINGS OF SIGGRAPH 2023 CONFERENCE PAPERS, SIGGRAPH 2023(2023)
摘要
Physical systems ranging from elastic bodies to kinematic linkages are defined on high-dimensional configuration spaces, yet their typical low-energy configurations are concentrated on much lower-dimensional subspaces. This work addresses the challenge of identifying such subspaces automatically: given as input an energy function for a high-dimensional system, we produce a low-dimensional map whose image parameterizes a diverse yet low-energy submanifold of configurations. The only additional input needed is a single seed configuration for the system to initialize our procedure; no dataset of trajectories is required. We represent subspaces as neural networks that map a low-dimensional latent vector to the full configuration space, and propose a training scheme to fit network parameters to any system of interest. This formulation is effective across a very general range of physical systems; our experiments demonstrate not only nonlinear and very low-dimensional elastic body and cloth subspaces, but also more general systems like colliding rigid bodies and linkages. We briefly explore applications built on this formulation, including manipulation, latent interpolation, and sampling.
更多查看译文
关键词
simulation,neural network,subspaces
AI 理解论文
溯源树
样例
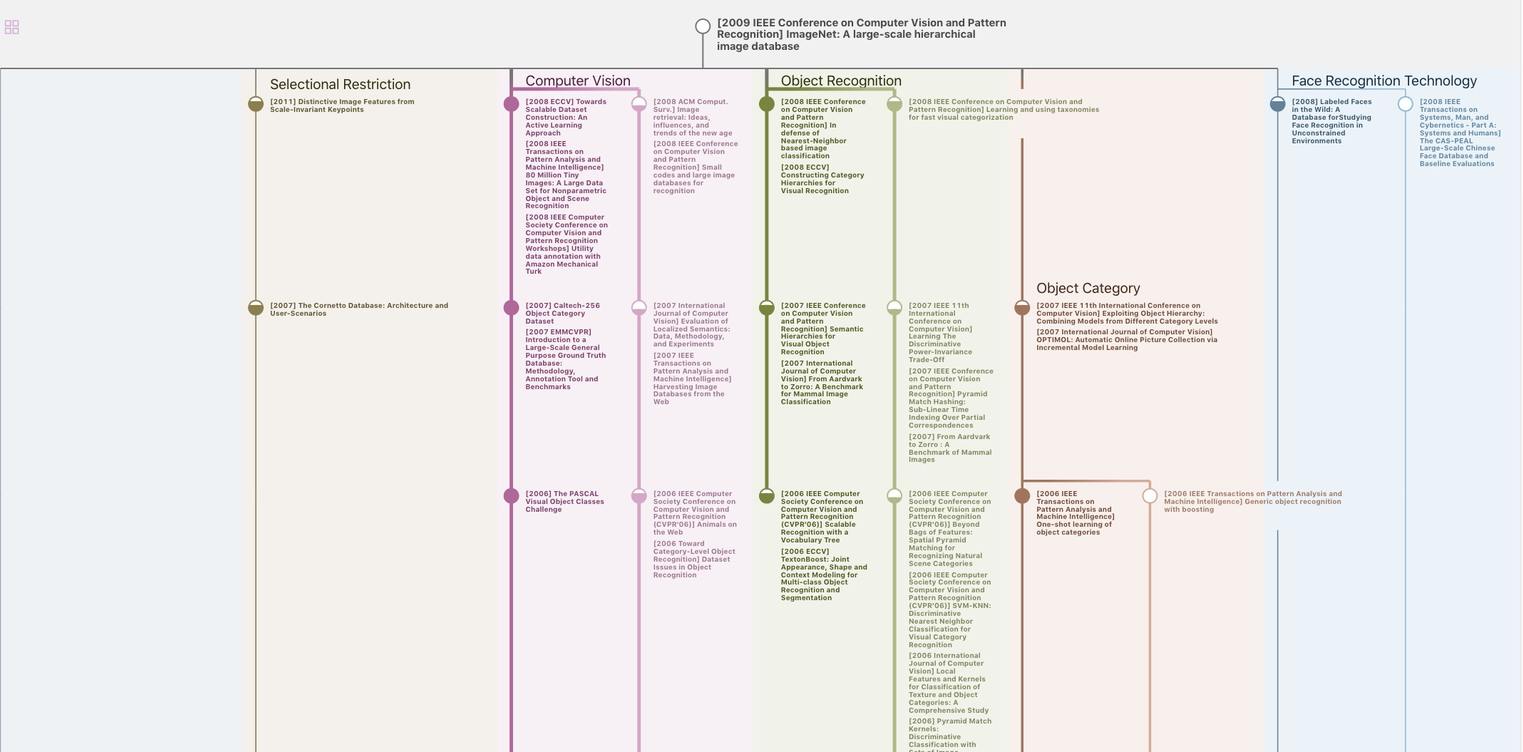
生成溯源树,研究论文发展脉络
Chat Paper
正在生成论文摘要