Flock: Accurate network fault localization at scale
CoRR(2023)
摘要
Inferring the root cause of failures among thousands of components in a data center network is challenging, especially for "gray" failures that are not reported directly by switches. Faults can be localized through end-to-end measurements, but past localization schemes are either too slow for large-scale networks or sacrifice accuracy. We describe Flock, a network fault localization algorithm and system that achieves both high accuracy and speed at datacenter scale. Flock uses a probabilistic graphical model (PGM) to achieve high accuracy, coupled with new techniques to dramatically accelerate inference in discrete-valued Bayesian PGMs. Large-scale simulations and experiments in a hardware testbed show Flock speeds up inference by >10000x compared to past PGM methods, and improves accuracy over the best previous datacenter fault localization approaches, reducing inference error by 1.19-11x on the same input telemetry, and by 1.2-55x after incorporating passive telemetry. We also prove Flock's inference is optimal in restricted settings
更多查看译文
关键词
datacenter networks,fault localization,gray failures
AI 理解论文
溯源树
样例
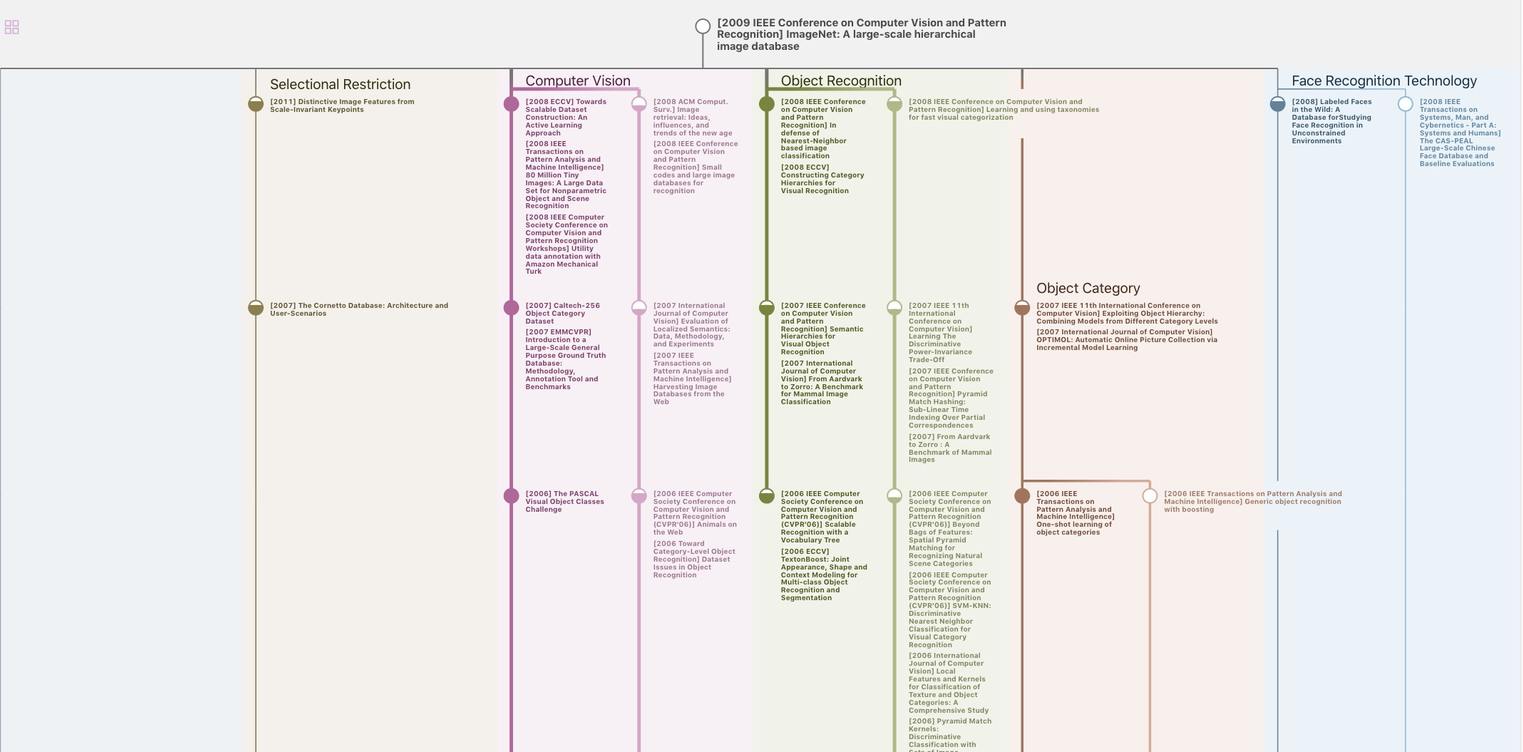
生成溯源树,研究论文发展脉络
Chat Paper
正在生成论文摘要