Next-Generation Seismic Monitoring with Neural Operators
arxiv(2023)
摘要
Seismic phase picking is the task of annotating seismograms with seismic wave arrival times and underpins earthquake monitoring operations globally. State-of-the-art approaches for phase picking use deep neural networks to annotate seismograms at each station independently; this is in stark contrast to the way that human experts annotate seismic data, in which waveforms from the whole network are examined simultaneously. With the performance gains of single-station algorithms approaching saturation, it is clear that meaningful future advances will require algorithms that can naturally examine data for entire networks at once. Here, we introduce a general-purpose network-wide phase picking algorithm, PhaseNO, that is based on a recently developed machine learning paradigm called Neural Operator. PhaseNO can use data from any number of stations arranged in any arbitrary geometry to pick phases across the entire seismic network simultaneously. By leveraging the natural spatial and temporal contextual information, PhaseNO achieves superior performance over leading baseline algorithms by detecting many more earthquakes, picking many more phase arrivals, yet also greatly improving measurement accuracy. Following similar trends being seen across the domains of artificial intelligence, our approach provides but a glimpse of the potential gains from fully-utilizing the massive seismic datasets being collected around the world.
更多查看译文
AI 理解论文
溯源树
样例
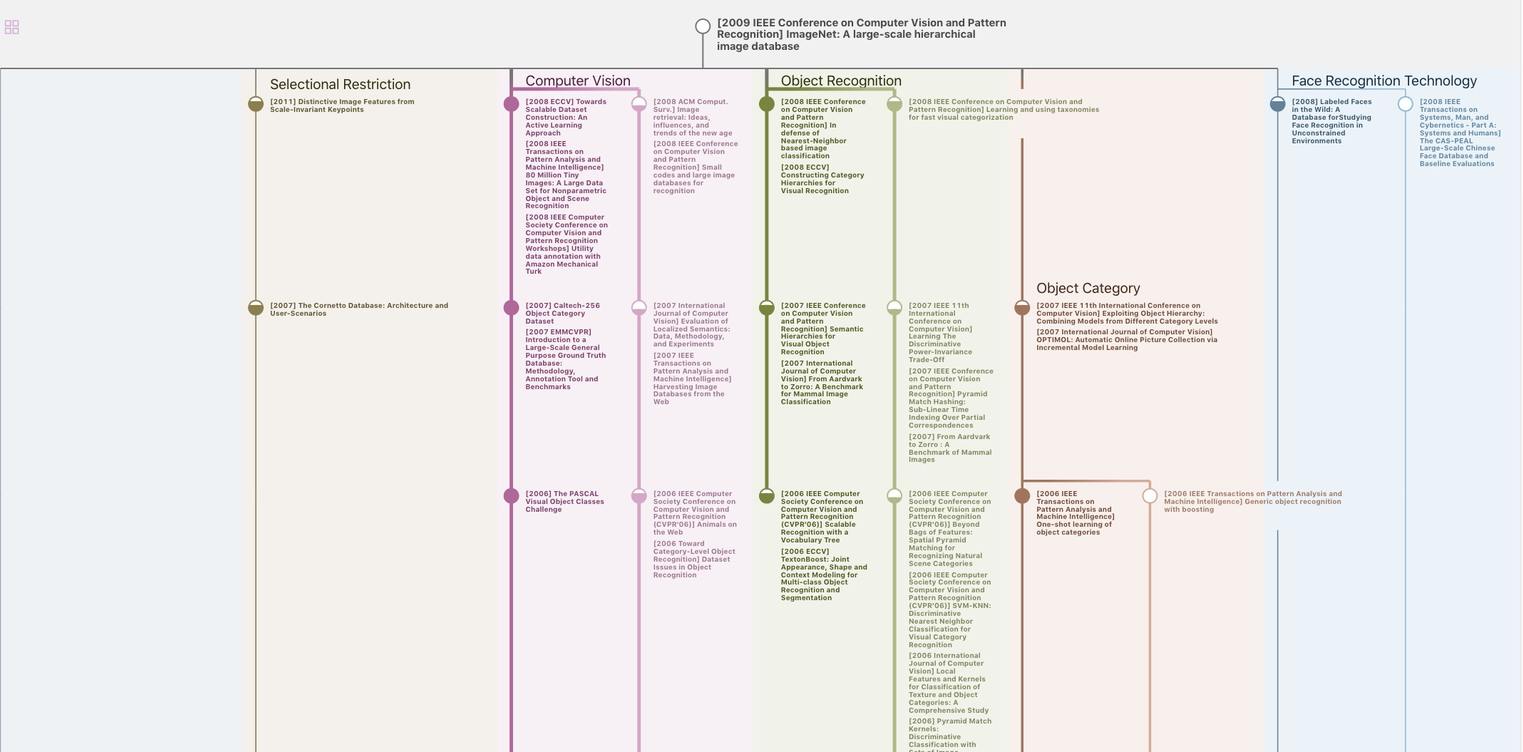
生成溯源树,研究论文发展脉络
Chat Paper
正在生成论文摘要