Salient Co-Speech Gesture Synthesizing with Discrete Motion Representation
ICASSP 2023 - 2023 IEEE International Conference on Acoustics, Speech and Signal Processing (ICASSP)(2023)
摘要
Synthesizing co-speech gestures is challenging because the mapping from speech to gesticulation is inherently non-deterministic. When giving talks, people conduct not only gentle and rhythmic motions but also abrupt and salient gesticulations. Most previous research efforts, however, ignore this nature of co-speech gestures and synthesize deterministic results, producing over-smoothed movements with limited expressiveness. To address this issue, we propose a new co-speech gesture generation approach that produces high-quality salient gesticulations. Specifically, we build a discrete motion representation (DMR) space to bridge the speech-gesture mapping and the gesture generation stages. The incorporation of DMR enables random sampling in motion space and avoids the over-smooth problem in speech-gesture mapping. Based on DMR, we devise a novel multi-modal co-speech gesture synthesis model with temporal attention (MCGT). MCGT explicitly models DMR’s categorical distribution conditioned on the speech context, which captures complex context patterns and produces more salient gesticulations in sync with the context. In addition, we construct a new benchmark for evaluating salient motion quality in co-speech gestures, containing a large-scale co-speech gesture dataset with salient gesticulations. We also introduce a new metric, referred to as salient motion similarity, to evaluate the salient motion quality. Experiments demonstrate superior results from our approach over several competing baselines.
更多查看译文
关键词
Co-speech gesture,Discrete motion representation,Temporal attention
AI 理解论文
溯源树
样例
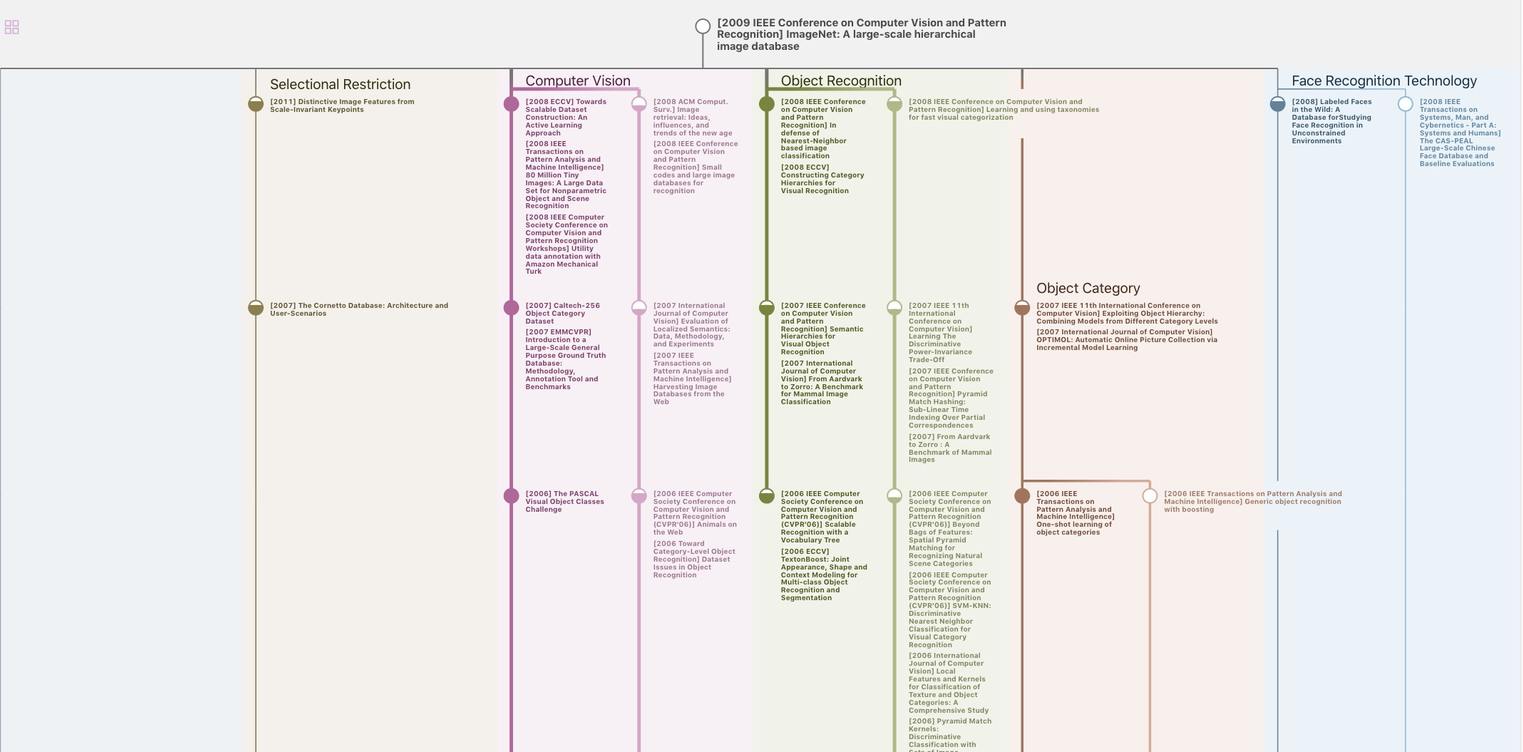
生成溯源树,研究论文发展脉络
Chat Paper
正在生成论文摘要