A Chaotic Local Search-Based Particle Swarm Optimizer for Large-Scale Complex Wind Farm Layout Optimization
IEEE/CAA Journal of Automatica Sinica(2023)
摘要
Wind energy has been widely applied in power generation to alleviate climate problems. The wind turbine layout of a wind farm is a primary factor of impacting power conversion efficiency due to the wake effect that reduces the power outputs of wind turbines located in downstream. Wind farm layout optimization (WFLO) aims to reduce the wake effect for maximizing the power outputs of the wind farm. Nevertheless, the wake effect among wind turbines increases significantly as the number of wind turbines increases in the wind farm, which severely affect power conversion efficiency. Conventional heuristic algorithms suffer from issues of low solution quality and local optimum for large-scale WFLO under complex wind scenarios. Thus, a chaotic local search-based genetic learning particle swarm optimizer (CGPSO) is proposed to optimize large-scale WFLO problems. CGPSO is tested on four larger-scale wind farms under four complex wind scenarios and compares with eight state-of-the-art algorithms. The experiment results indicate that CGPSO significantly outperforms its competitors in terms of performance, stability, and robustness. To be specific, a success and failure memories-based selection is proposed to choose a chaotic map for chaotic search local. It improves the solution quality. The parameter and search pattern of chaotic local search are also analyzed for WFLO problems.
更多查看译文
关键词
Chaotic local search (CLS),evolutionary computation,genetic learning,particle swarm optimization (PSO),wake effect,wind farm layout optimization (WFLO)
AI 理解论文
溯源树
样例
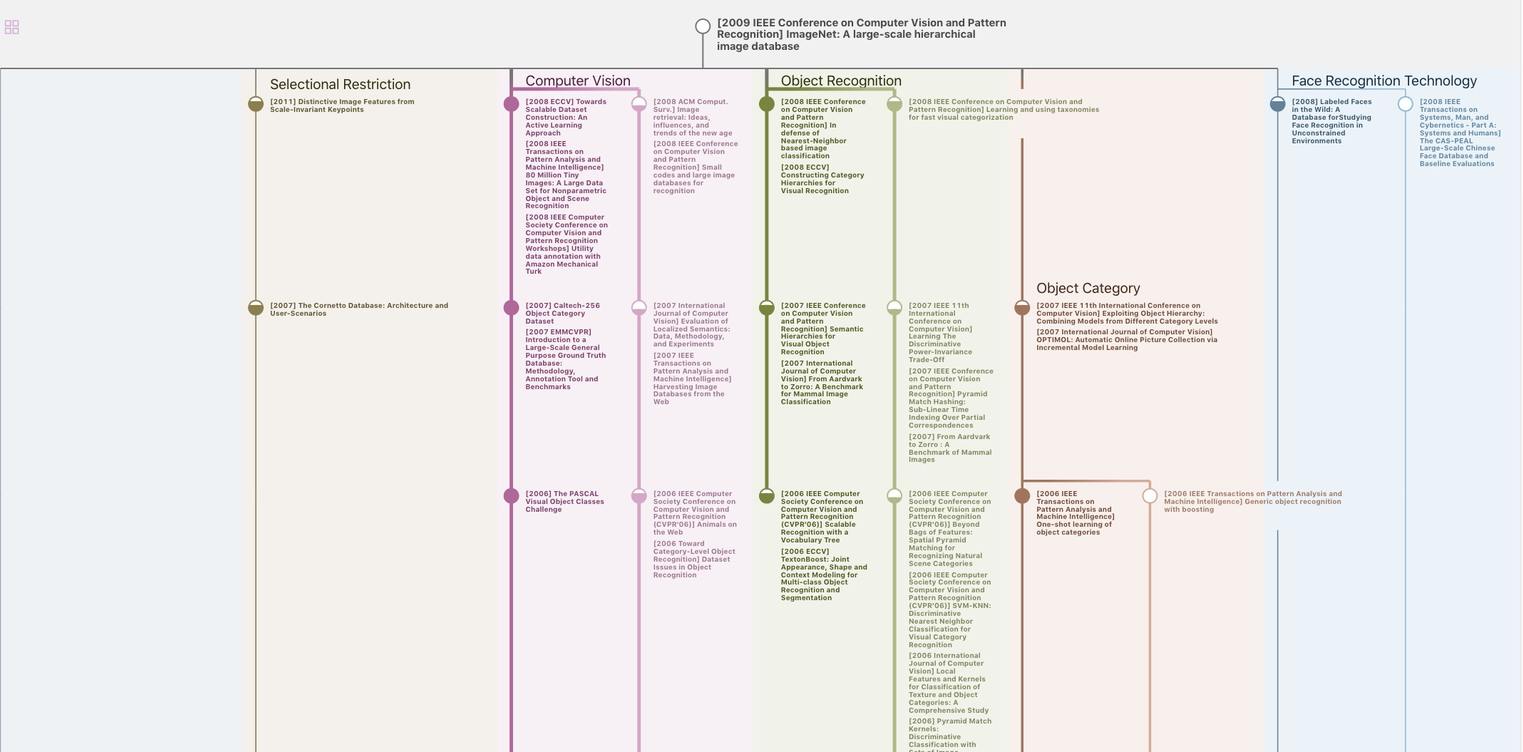
生成溯源树,研究论文发展脉络
Chat Paper
正在生成论文摘要