Democratizing Quality-Based Machine Learning Development through Extended Feature Models.
FASE(2023)
摘要
ML systems have become an essential tool for experts of many domains, data scientists and researchers, allowing them to find answers to many complex business questions starting from raw datasets. Nevertheless, the development of ML systems able to satisfy the stakeholders’ needs requires an appropriate amount of knowledge about the ML domain. Over the years, several solutions have been proposed to automate the development of ML systems. However, an approach taking into account the new quality concerns needed by ML systems (like fairness, interpretability, privacy, and others) is still missing. In this paper, we propose a new engineering approach for the quality-based development of ML systems by realizing a workflow formalized as a Software Product Line through Extended Feature Models to generate an ML System satisfying the required quality constraints. The proposed approach leverages an experimental environment that applies all the settings to enhance a given Quality Attribute, and selects the best one. The experimental environment is general and can be used for future quality methods’ evaluations. Finally, we demonstrate the usefulness of our approach in the context of multi-class classification problem and fairness quality attribute.
更多查看译文
关键词
machine learning development,machine learning,models,quality-based
AI 理解论文
溯源树
样例
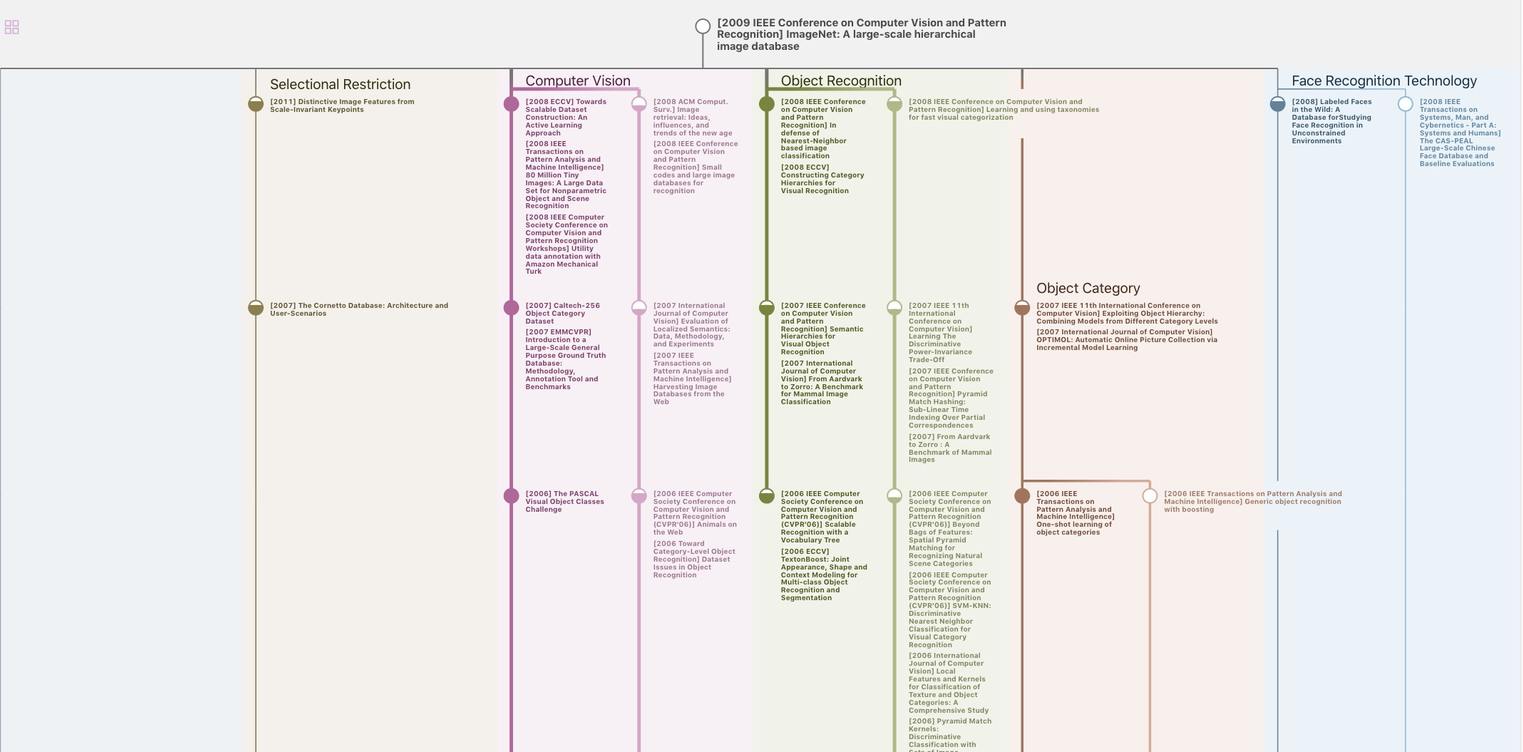
生成溯源树,研究论文发展脉络
Chat Paper
正在生成论文摘要