Optimized Client-side Detection of Model Poisoning Attacks in Federated learning.
HPCC/DSS/SmartCity/DependSys(2022)
摘要
Recent studies have shown that federated learning is vulnerable to a new type of poisoning attack, called model poisoning attack. One or more malicious clients send crafted local model updates to the server to poison the global model. The training data between federated learning clients is non-IID, which makes the updates submitted by the clients various. The poisoned update from the malicious client can be hidden between various benign updates. Many anomaly detection mechanisms are limited. Client detection is a flexible detection method suitable for non-IID data distribution in federated learning. The core idea of client-side detection is to evaluate the model using various client-side data, which is used for training and detecting model poisoning attacks. However, the effectiveness of this scheme depends on the authenticity of the report returned by the client. Malicious clients can return false reports to evade detection. In this paper, we adopt the idea of group testing and use the COMP algorithm to improve the detection process. We conduct experiments in settings with different proportions of malicious clients. Experimental results show that our scheme can tolerate a higher proportion of malicious clients. In the CIFAR-10 based semantic backdoor attack, our scheme is effective when the proportion of malicious clients is 20%. In MNIST-based semantic backdoor attacks, our scheme is effective when the proportion of malicious clients is 25%.
更多查看译文
关键词
Federated learning,Model poisoning attack,group testing
AI 理解论文
溯源树
样例
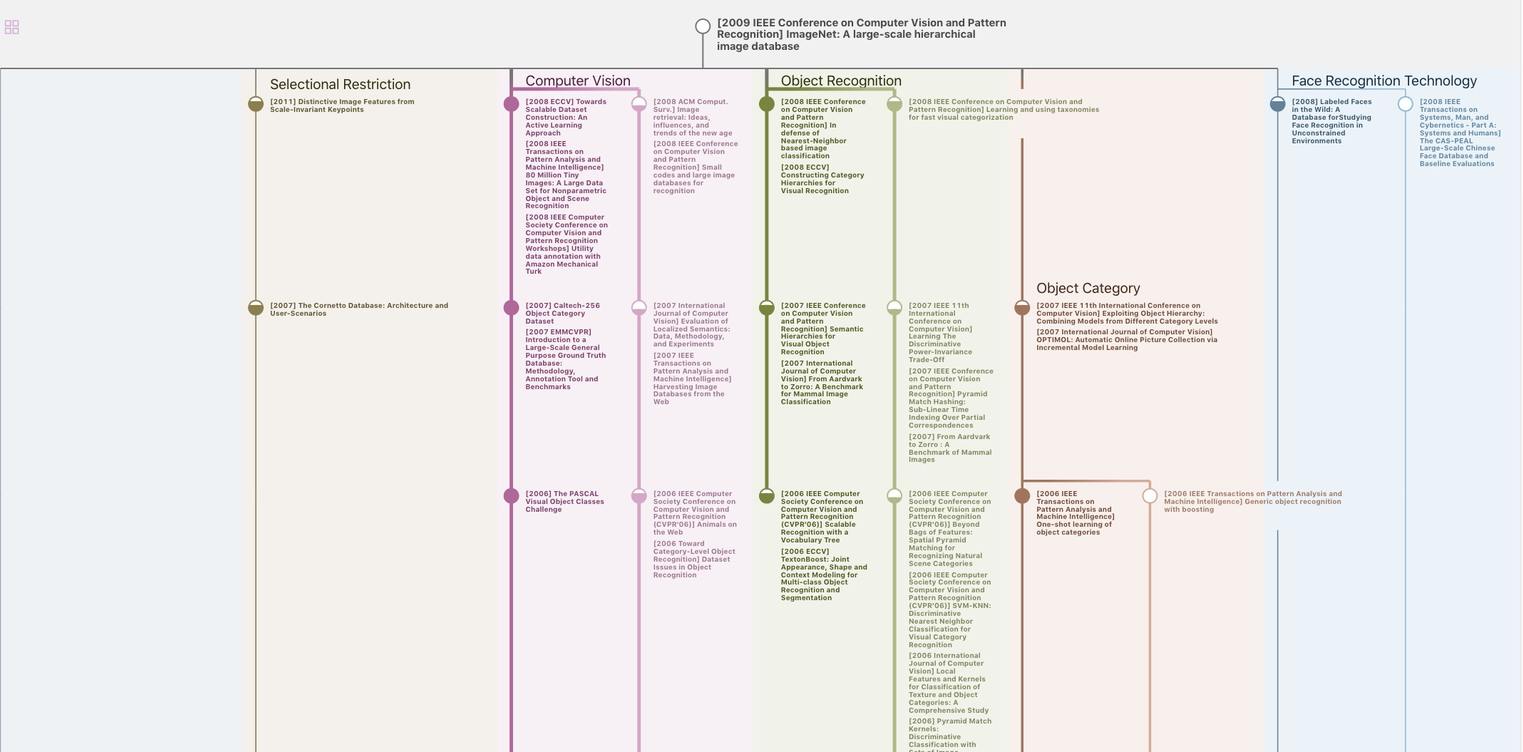
生成溯源树,研究论文发展脉络
Chat Paper
正在生成论文摘要