Optimal strategy selection for attack graph games using deep reinforcement learning.
HPCC/DSS/SmartCity/DependSys(2022)
摘要
While various defense mechanisms have been proposed in cybersecurity, it is still unclear how these defense mechanisms should be deployed in practice to mitigate the damage of cyber attacks. In this work, we propose a Stackelberg game model to simulate the interaction between cyber attackers and defenders. We develop a reinforcement learning (RL) based approach to seek the optimal defense strategy. We further design a policy iteration method to accelerate the convergence speed of training. We conduct experiments with real network data and various game settings to evaluate the performance of our approach. Experiment results show that our RL-based approach outperform baselines, and the approach is robust to the uncertainty security environment.
更多查看译文
关键词
cyber security,attack graph,game theory,deep reinforcement learning,policy iteration
AI 理解论文
溯源树
样例
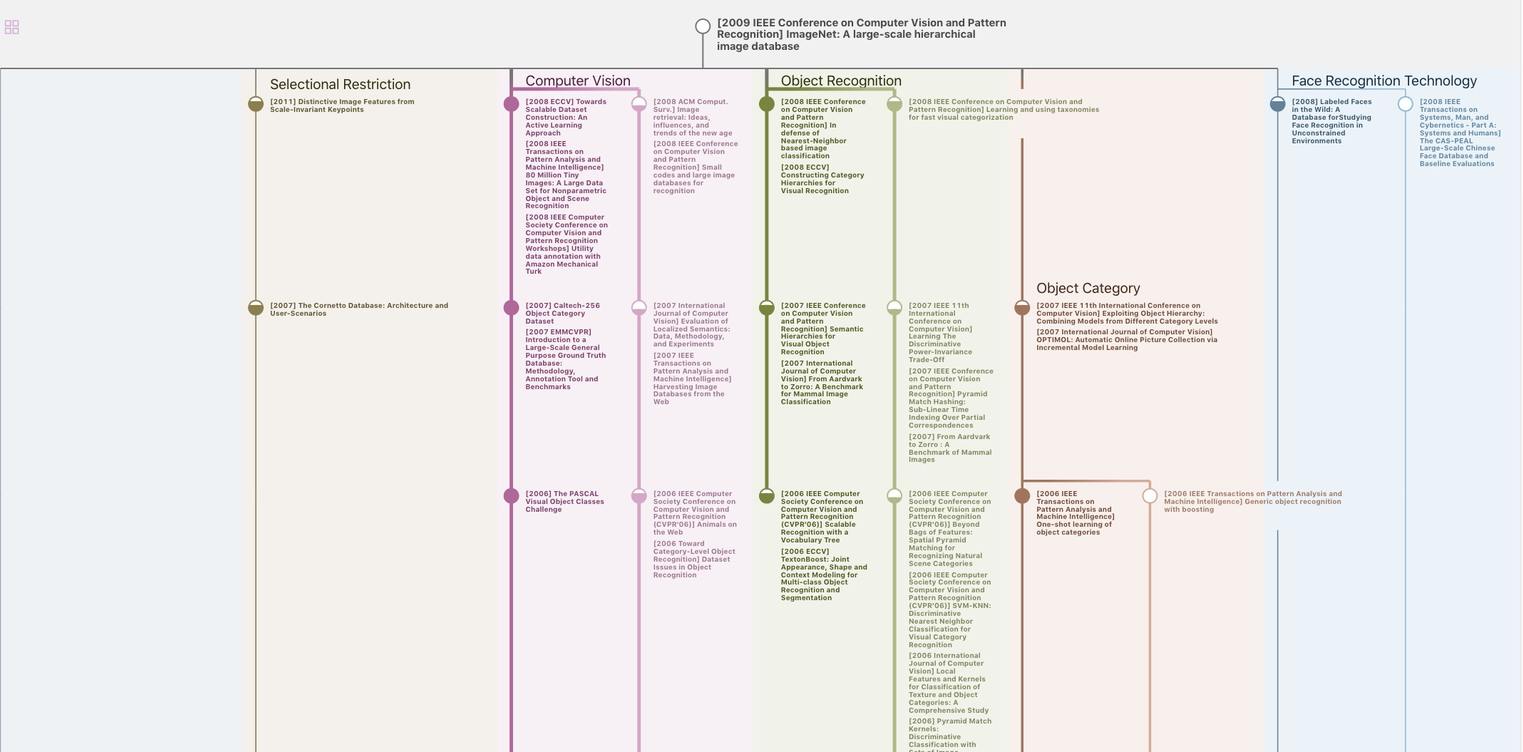
生成溯源树,研究论文发展脉络
Chat Paper
正在生成论文摘要