An Empirical Evaluation of Deep Neural Networks in Federated Learning.
HPCC/DSS/SmartCity/DependSys(2022)
摘要
With the fast development of artificial intelligence (e.g., deep learning), it brings the new opportunities and challenges for business management and service optimization of the business process management. However, there are few works to present the comprehensive analysis of neural network models in the new intelligent paradigm, especially of federated learning. In this paper, we attempt to give an empirical study of deep neural networks in federated learning, which is used for analyzing the possibility of applying federated learning to BPM. At first, we present an overview of the theoretical analysis of deep learning and its distributed extension, i.e., federated learning. Then, We introduce three classic deep neural network models, including multi-layer perception, convolutional neural network, and residual network, and the corresponding structure and the key components. Next, we give the detailed experimental setting for the common test dataset called MNIST, including the structure of neural networks and the setting of the hyperparameters. Finally, extensive experiments are performed to evaluate the performance of the neural networks in federated learning.
更多查看译文
关键词
image classification,empirical analysis,neural network,federated learning
AI 理解论文
溯源树
样例
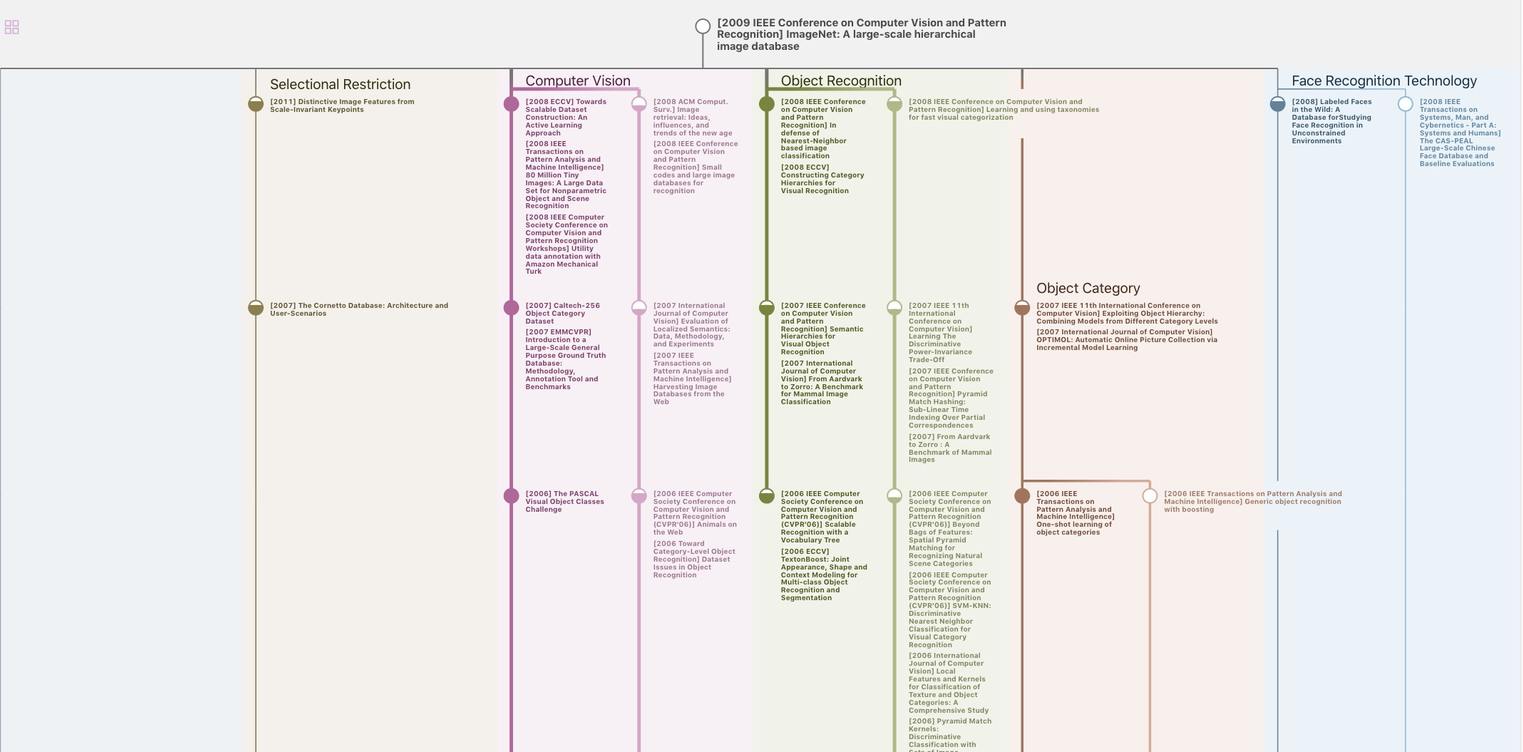
生成溯源树,研究论文发展脉络
Chat Paper
正在生成论文摘要