Multi-View Attention Networks with Contrastive Predictive Coding for Sequential Recommendation.
HPCC/DSS/SmartCity/DependSys(2022)
摘要
Attention-based models have achieved outstanding performance in sequential recommendation due to their ability to obtain better sequential representations. However, these models still suffer from over-fitting and over-parameterization issues. Regularization techniques such as dropout can alleviate the over-fitting problem, but an excessively high dropout rate means a greater probability of missing sequential critical relationships. This will lead to incomplete user representations and sub-optimal model performance. In addition, the single embedding of timestamps also leads to incomplete user representation. Therefore, we introduce a Multi-view Attention Network with Contrastive Predictive Coding (MVACPC) to solve the above problems in this paper. Specifically, we design a multi-view attention network to alleviate the problem of dropouts losing critical information in the sequence, which can obtain more comprehensive user representations. The network is based on the idea of parallel computing and parameter sharing without adding too much training time and parameters. In order to make the model learn more temporal information, We use different temporal encoding methods for multiple positive sample sequences obtained through data augmentation. Finally, we devise an extended contrastive predictive coding loss to optimize the model. We validate the effectiveness of the MVACPC on public datasets, and the experimental results show that MVACPC outperforms various state-of-the-art sequential recommendation models on different evaluation metrics.
更多查看译文
关键词
sequential recommendation,multi-view attention network,muti-time,contrastive predictive coding
AI 理解论文
溯源树
样例
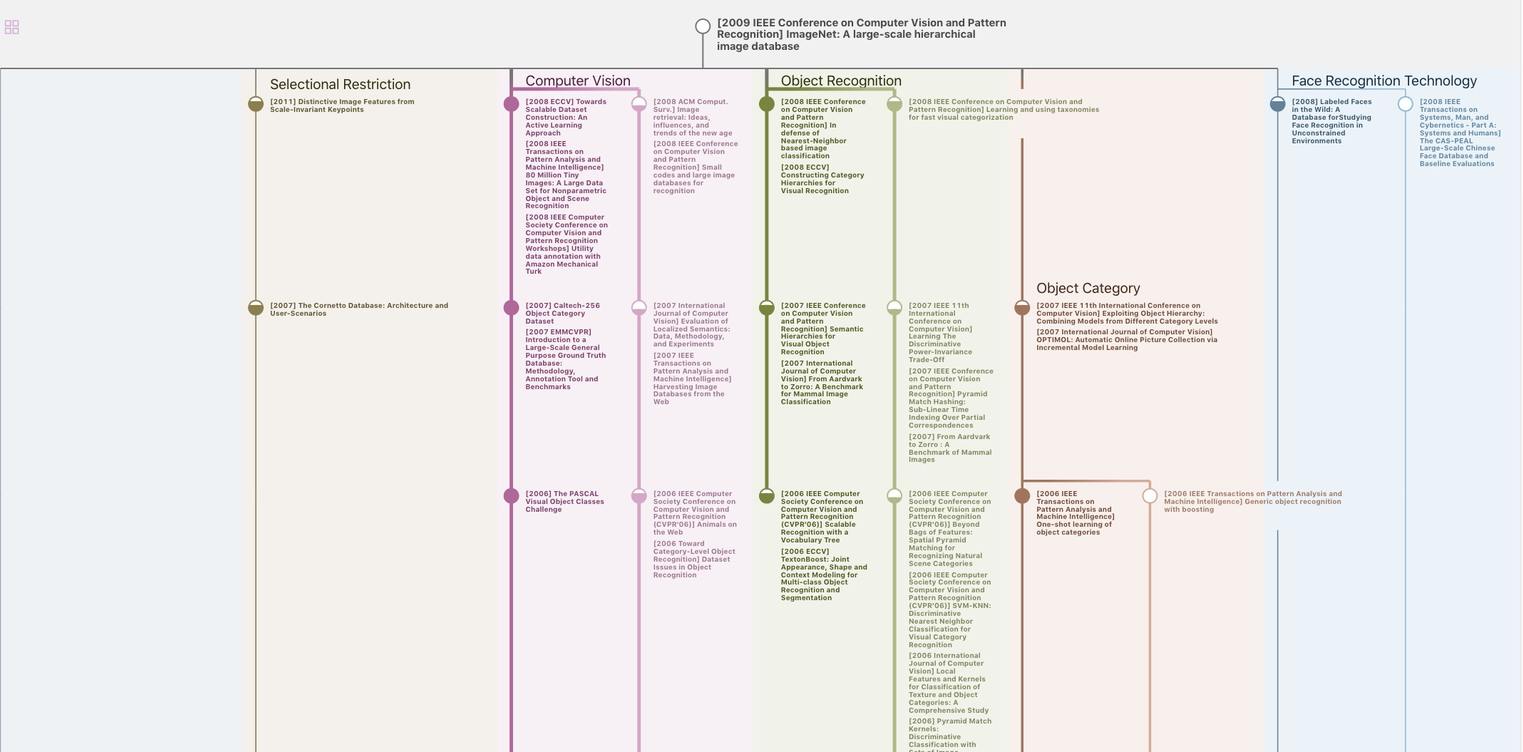
生成溯源树,研究论文发展脉络
Chat Paper
正在生成论文摘要