TE-DyGE: Temporal Evolution-Enhanced Dynamic Graph Embedding Network.
DASFAA (3)(2023)
摘要
Dynamic graph representation has gradually attached more attention in the research area since many real-world graphs evolve over time. Recent works typically represent the dynamic graph as a sequence of discrete static subgraphs and apply a recurrent neural network (RNN) to capture graph evolution over multiple discrete-time subgraphs. However, these approaches fail to capture the fine-grained temporal evolution leading to introducing temporal invalid information into the structure representation. In this paper, we propose a novel temporal evolution-enhanced dynamic graph embedding method (TE-DyGE) to address this limitation. To capture the fine-grained temporal evolution, TE-DyGE first applies a temporal random walk to filter out temporally valid information. Additionally, we introduce temporal-dependent weight to enhance the structure attention network. We evaluate the TE-DyGE in link prediction tasks. The experimental results demonstrate a generally higher performance of TE-DyGE compared with several state-of-the-art related baselines.
更多查看译文
关键词
dynamic graph,te-dyge,evolution-enhanced
AI 理解论文
溯源树
样例
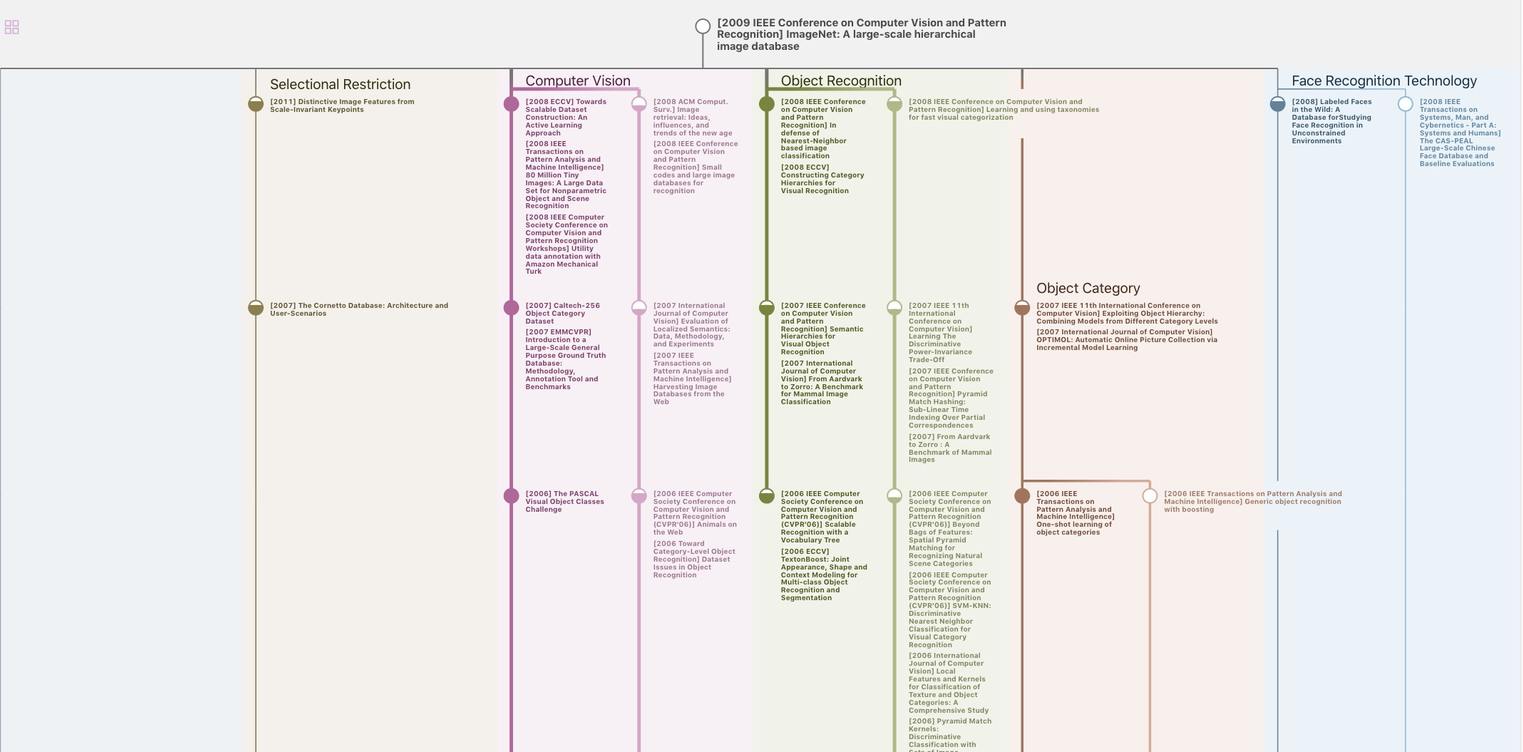
生成溯源树,研究论文发展脉络
Chat Paper
正在生成论文摘要