Leveraging Existing Literature on the Web and Deep Neural Models to Build a Knowledge Graph Focused on Water Quality and Health Risks.
WWW(2023)
摘要
A knowledge graph focusing on water quality in relation to health risks posed by water activities (such as diving or swimming) is not currently available. To address this limitation, we first use existing resources to construct a knowledge graph relevant to water quality and health risks using KNowledge Acquisition and Representation Methodology (KNARM). Subsequently, we explore knowledge graph completion approaches for maintaining and updating the graph. Specifically, we manually identify a set of domain-specific UMLS concepts and use them to extract a graph of approximately 75,000 semantic triples from the Semantic MEDLINE database (which contains head-relation-tail triples extracted from PubMed). Using the resulting knowledge graph, we experiment with the KG-BERT approach for graph completion by employing pre-trained BERT/RoBERTa models and also models fine-tuned on a collection of water quality and health risks abstracts retrieved from the Web of Science. Experimental results show that KG-BERT with BERT/RoBERTa models fine-tuned on a domain-specific corpus improves the performance of KG-BERT with pre-trained models. Furthermore, KG-BERT gives better results than several translational distance or semantic matching baseline models.
更多查看译文
AI 理解论文
溯源树
样例
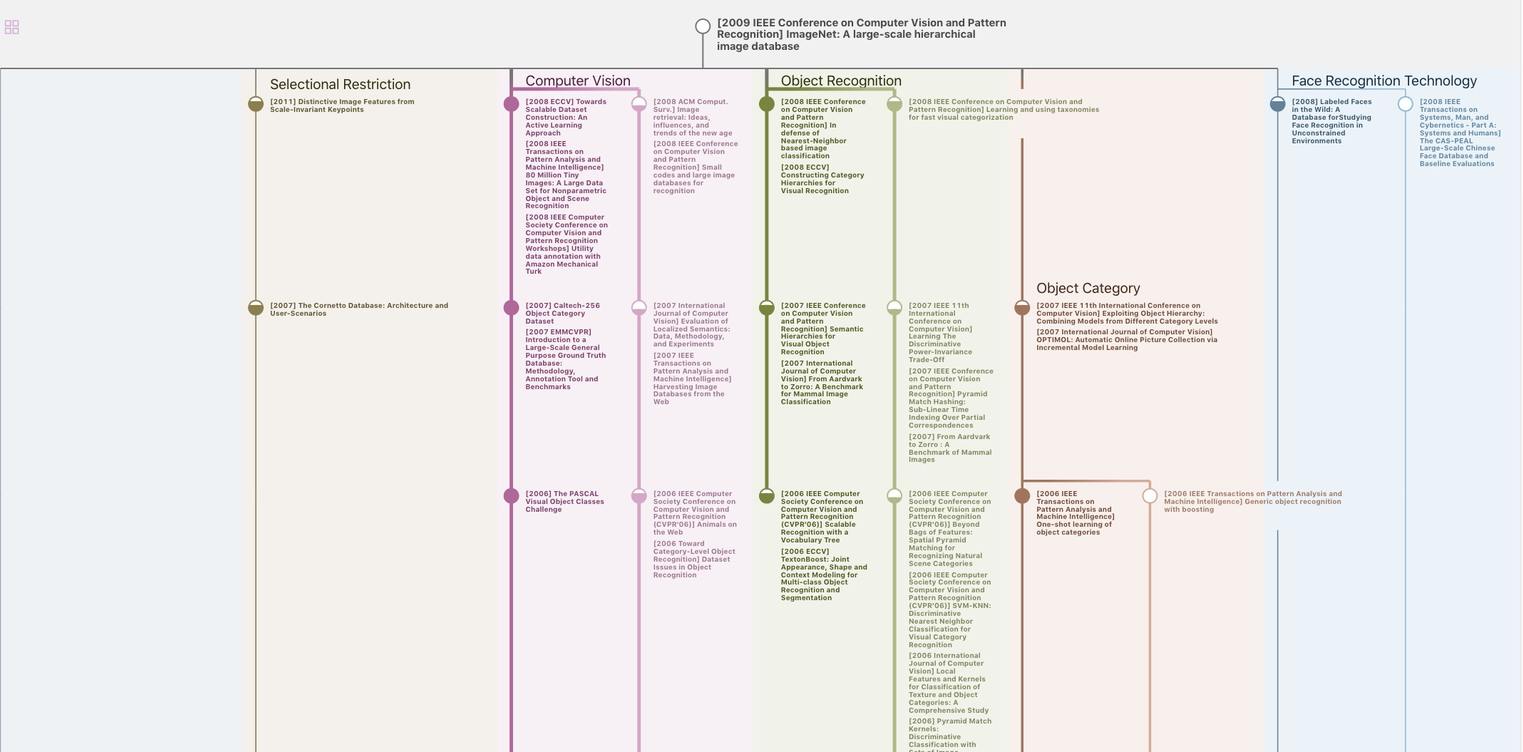
生成溯源树,研究论文发展脉络
Chat Paper
正在生成论文摘要