Reinforcement Learning Based Incentive Mechanism for Federated Meta Learning: A Game-Theoretic Perspective.
ICTAI(2022)
摘要
Federated learning (FL) is a novel decentralized machine learning mechanism, which bridges data silos to train a global model by utilizing data and computation power of local clients in a privacy-preserving way. Moreover, to handle heterogeneous data across domains, tasks and partities, federated meta-learning (FML) has been proposed, which leverages the fast adaptation of meta-learning for transferable and customizable models. Nevertheless, most of the existing studies focus on providing personalized models for different users, leaving other important issues not well solved, especially, incentive mechanisms, which are used as a common foundation for FML to attrack and maintain high-quality and reputable clients. To fill the gap, this paper proposes a learning-based incentive mechanism for FML to motivate local clients to participate in the data federation. First, we propose to reward clients according to the amount of data they contribute to the model training. Then, to analyze the behaviors of model owner and local clients, we formulate the incentivized training task as a Stackelberg game, and design a method based on reinforcement learning (RL) to learn optimal pricing and participating strategies for the task publisher and the local clients, respectively. Lastly, extensive experiments are conducted to demonstrate the efficiency and effectiveness of the proposed RL-based incentive mechanism, which assists multiple parties to reach the equilibrium of the game designed for FML.
更多查看译文
关键词
Reinforcement Learning, Stackelberg Game, Federated Meta-Learning, Incentive Mechanism
AI 理解论文
溯源树
样例
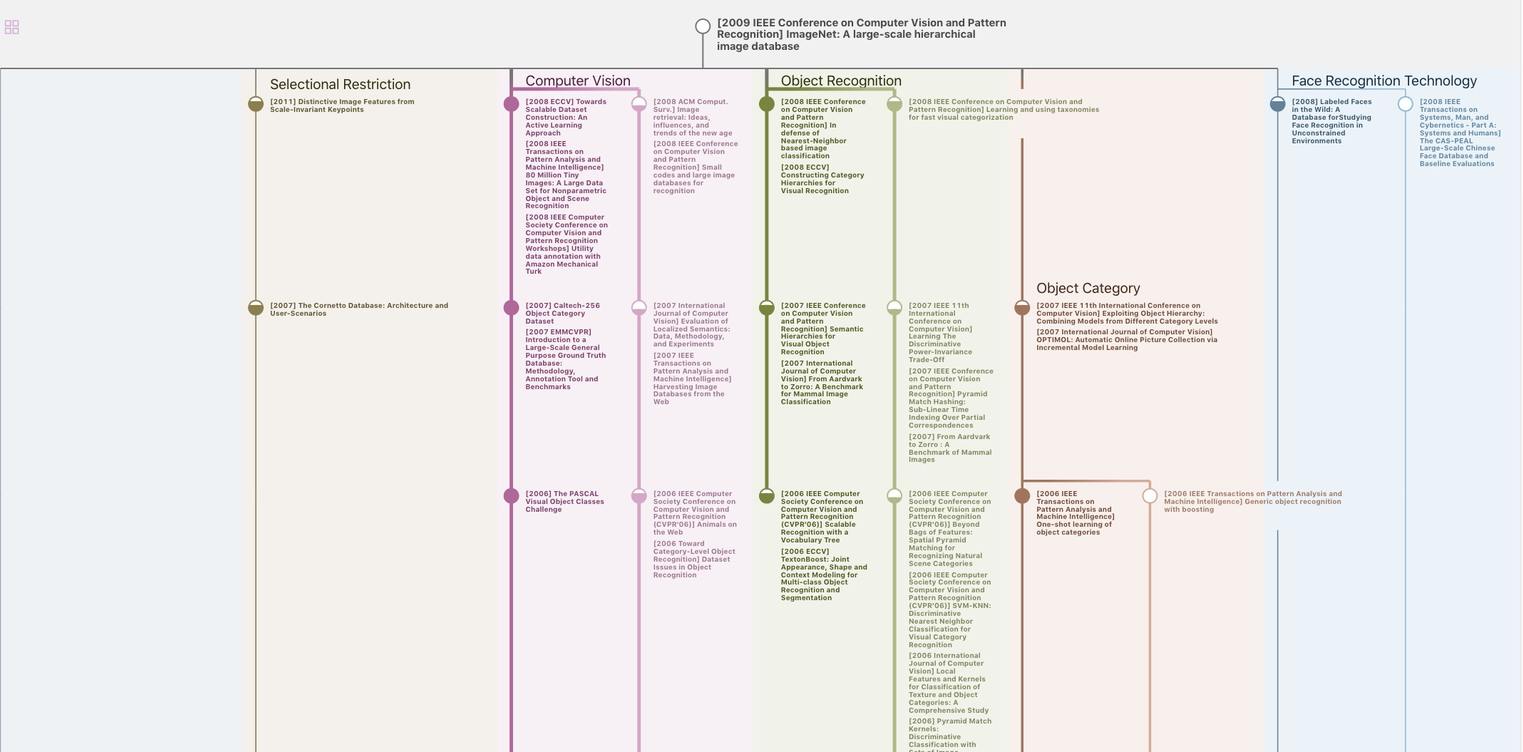
生成溯源树,研究论文发展脉络
Chat Paper
正在生成论文摘要