The Impact of Dynamic Adjustment of Swarm Behavior on Particle Swarm Optimization Performance using Benchmark Functions.
CCWC(2023)
摘要
Solving Optimization problems require finding the best solution while considering a large set of possible feasible answers. One of the important factors in tuning the search optimization methods that can significantly impact their performances is to determine what parameter settings to use. Real world problems are often consist of search spaces that are quite dynamic with varying degrees of complexities. In such search spaces, the conventional search optimization methods in which the parameters and behaviors of the optimization methods are pre-set and unchanging in response to the changes in the complexity of search space from one iteration to the next are known to be less adequate and unsuitable. Particle Swarm Optimization (PSO) is known as one of the most well-known meta-heuristics with known search characteristics in response to benchmark functions and is considered as methodological basis for multiple dynamic search behavior innovations this study proposes to adapt to search dynamics of a problem in each iteration. This study introduces three dynamic behavior-based PSO methods (DPPSO, DACPSO, and C-AEPSO). These methods are inspired from heterogeneous and sub-swarm principles. Thirteen benchmark functions are considered to evaluate the performance of the methods proposed. DPPSO and C-AEPSO are found to outperform a collection of 8 well-known evolutionary optimization methods across the benchmark functions considered. The results indicated that DACPSO is ranked as one of the top four best performing algorithms in this study, further indicating the superiority of the methods proposed.
更多查看译文
关键词
Particle Swarm Optimization, Dynamic Behaviour, Dynamic Parameter tuning, Dynamic Acceleration Coefficients
AI 理解论文
溯源树
样例
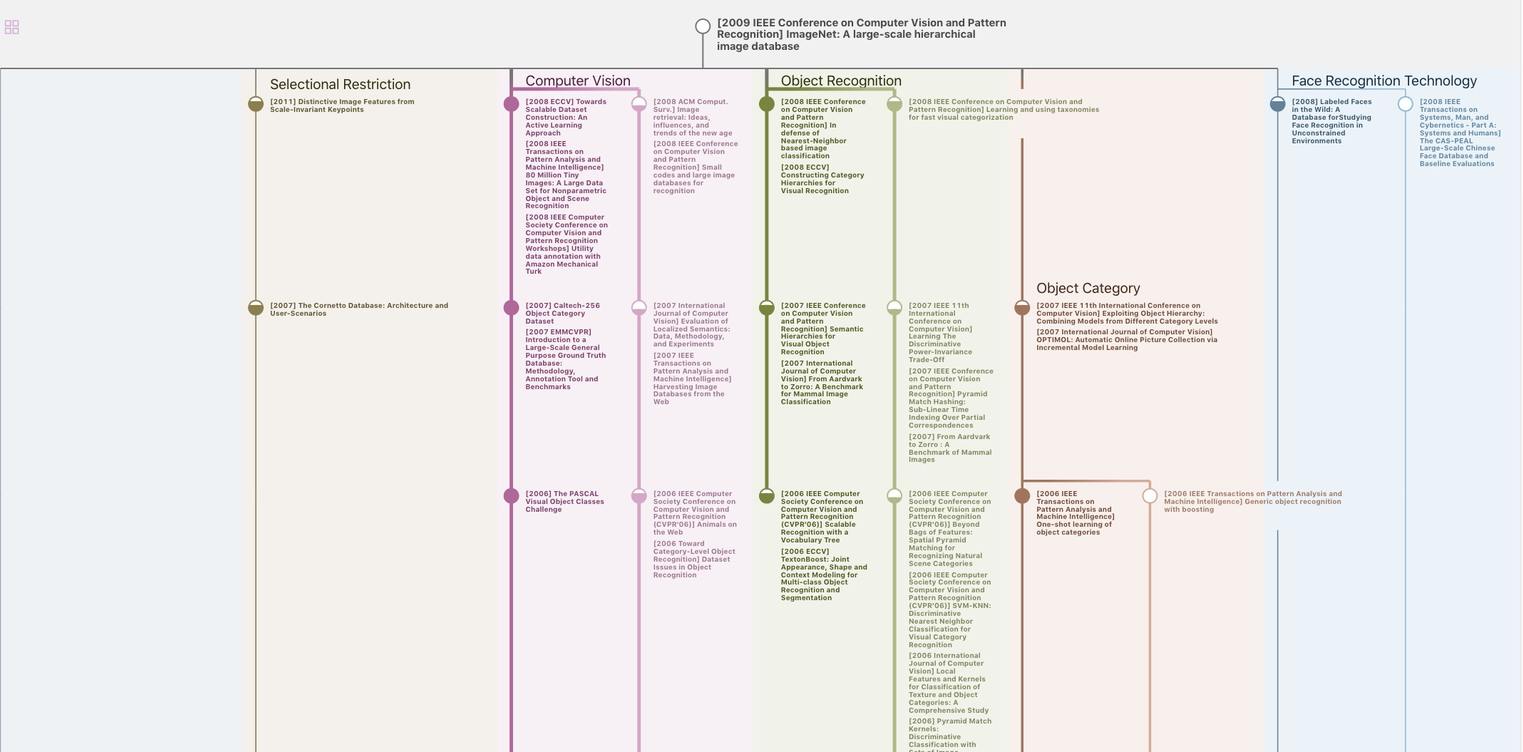
生成溯源树,研究论文发展脉络
Chat Paper
正在生成论文摘要