Factual Error Correction in Summarization with Retriever-Reader Pipeline.
ICONIP (1)(2022)
摘要
Summarization models compress the source text without sacrificing the primary information. However, about 30% of summaries produced by state-of-the-art summarization models suffer from the factual inconsistencies between source text and summary, also known as hallucinations, making them less trustworthy. It has been challenging to reduce hallucinations, especially entity hallucinations. Most prior works use the entire source text for factual error correction, while input length is often limited. It prevents models from checking facts precisely and limits its applications on long document summarization. To address this issue, we propose a post-editing factual error correction method based on a Retriever-Reader pipeline. After the summarization model generates the summary, entities in the summary are examined and corrected iteratively by retrieving and reading only the most relevant parts of the source document. We validate the proposed approach on the CNN/DM and Gigaword datasets. Experiments show that the proposed approach outperforms all the baseline models in the consistency metric. Better results are also achieved on human evaluations.
更多查看译文
关键词
Summarization, Entity hallucination, Post-editing factual error correction
AI 理解论文
溯源树
样例
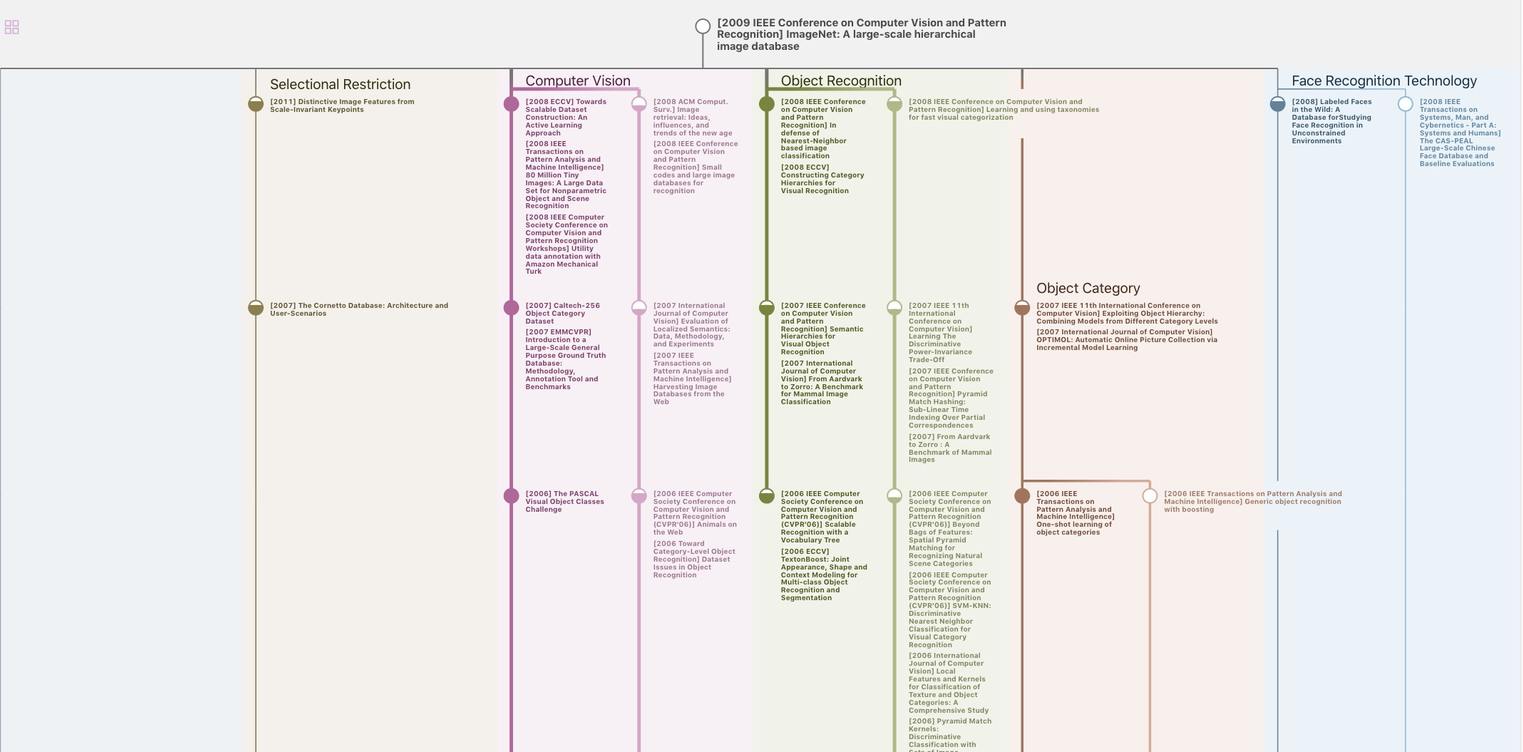
生成溯源树,研究论文发展脉络
Chat Paper
正在生成论文摘要