Heterogeneous Graph Representation for Knowledge Tracing.
ICONIP (1)(2022)
摘要
Knowledge tracing (KT) is a fundamental task of intelligent education, which traces students’ knowledge states by their historical interactions. In KT, students, questions, concepts, and answers are four main types of entities, and they contain various relations, including student-question interactive relations, question-concept relations, and question-answer relations. Such rich knowledge in these heterogeneous relations could potentially improve the prediction of KT. However, it has not been sufficiently utilized in existing KT methods. In this paper, we propose a novel method, called Heterogeneous Graph Representation for Knowledge Tracing, to leverage these useful relations. Our method first models all the complex entities and relations in KT as a heterogeneous graph, and then uses a heterogeneous graph neural network to obtain entities’ feature representations. After that, we feed the feature embeddings to a KT model in an end-to-end training manner. Due to the heterogeneous graph’s high representational capacity, our method exploits the relations among students, questions, concepts, and answers in a concise and unified way. Experiments on four KT datasets show that our method achieves state-of-the-art performance.
更多查看译文
关键词
heterogeneous graph representation,knowledge
AI 理解论文
溯源树
样例
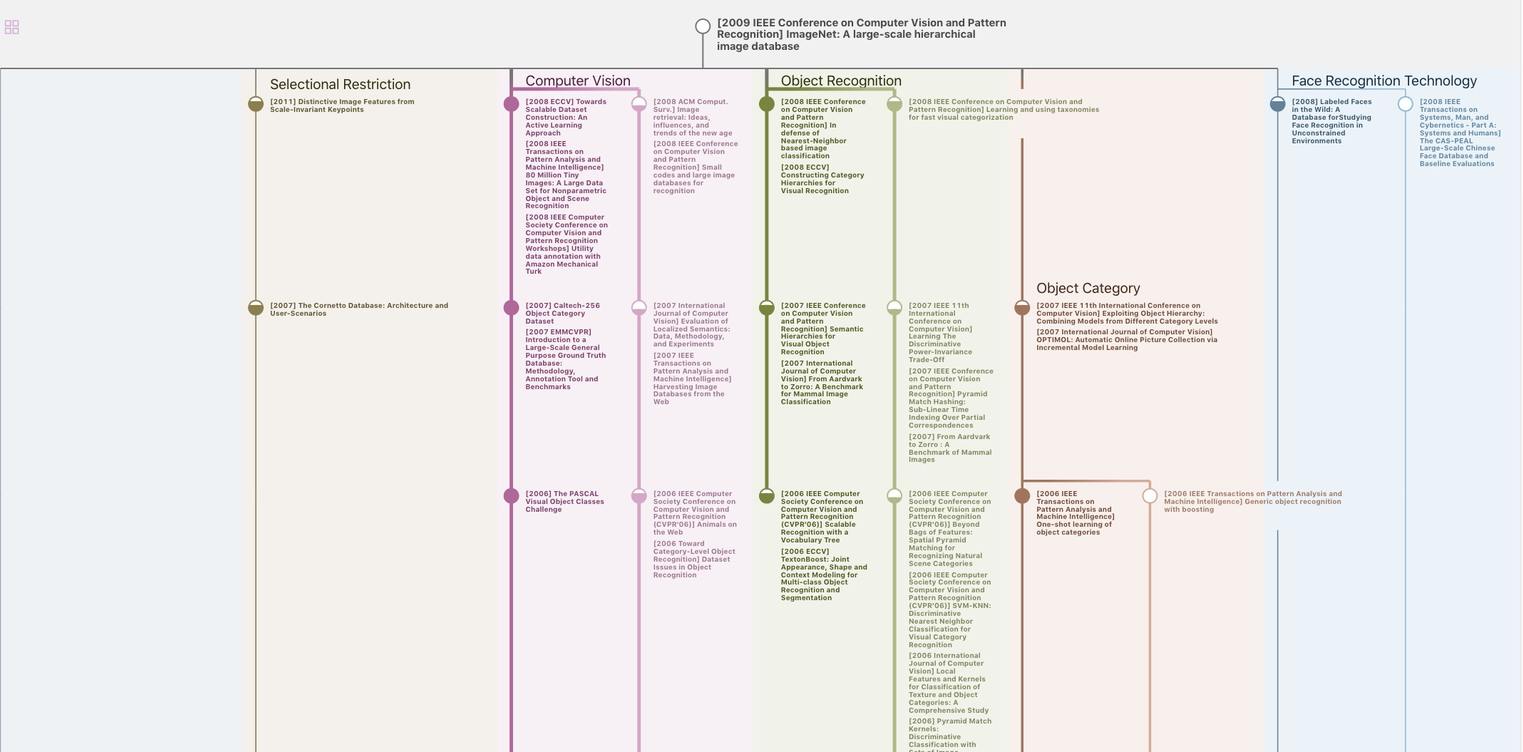
生成溯源树,研究论文发展脉络
Chat Paper
正在生成论文摘要