Enhancing Paraphrase Question Generation With Prior Knowledge
IEEE ACM Trans. Audio Speech Lang. Process.(2023)
摘要
Paraphrase question generation (PQG) aims to rewrite a given original question to a new paraphrase question, where the paraphrase question needs to have the same expressed meaning as the original question, but have a difference in expression form. Existing methods on PQG mainly focus on synonym substitution or word order adjustment based on the original question. However, rewriting based on the word-level may not guarantee the difference between paraphrase questions and original questions. In this paper, we propose a knowledge-aware paraphrase question generation model. Our model first employs a knowledge extractor to extract the prior knowledge related to the original question from the knowledge base. Then an attention mechanism and a gate mechanism are introduced in our model to selectively utilize the extracted prior knowledge for rewriting, which helps to expand the content of the generated question to maximize the difference. Additionally, we use a discriminator module to promote the generated paraphrase to be semantically close to the original question and the ground truth. Specifically, the loss function of the discriminator penalizes the excessive distance between the representation of the paraphrase question and the ground truth. Extensive experiments on the Quora dataset show that the proposed model outperforms the baselines. Further, our model is applied to the SQuAD dataset, which proves the generalization ability of our model in the existing QA dataset.
更多查看译文
关键词
Task analysis,Decoding,Speech processing,Logic gates,Knowledge based systems,Deep learning,Internet,Paraphrase question generation,prior knowledge,discriminator module
AI 理解论文
溯源树
样例
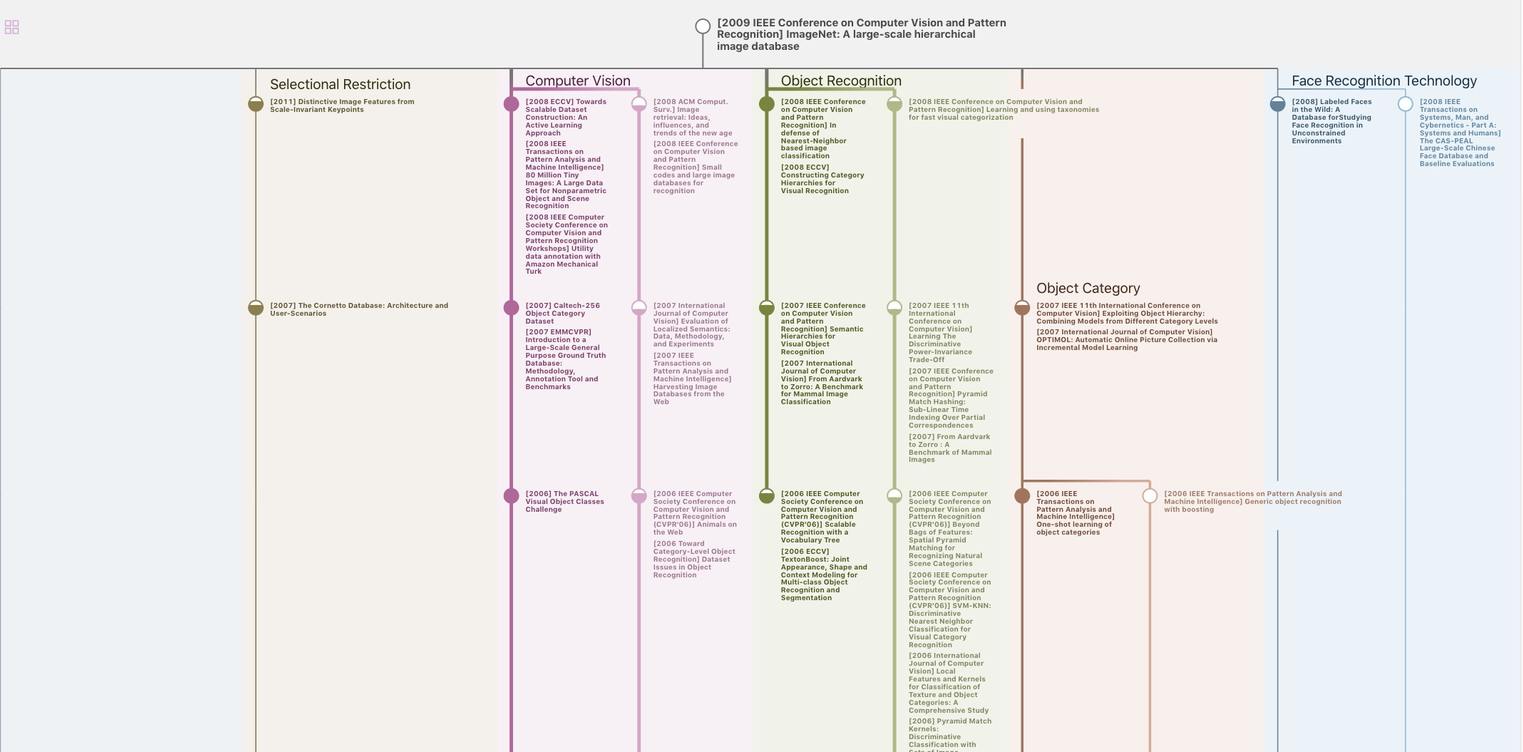
生成溯源树,研究论文发展脉络
Chat Paper
正在生成论文摘要