Robust decentralized stochastic gradient descent over unstable networks.
Comput. Commun.(2023)
摘要
Decentralized learning is essential for large-scale deep learning due to its great advantage in breaking the communication bottleneck. Most decentralized learning algorithms focus on reducing the communication overhead without taking into account the possibility of a shaky network connection, and existing analyses over unstable networks have various limitations such as centralized settings, strong unrealistic assumptions, etc. Hence, in this work, we study a non-convex optimization problem over unstable networks that fully consider unstable factors including unstable network connections, communication and artificially injected noise. Specifically, we focus on the most commonly used Stochastic Gradient Descent (SGD) algorithm in a mild decentralized setting and propose a robust algorithm to handle unstable networks. It is shown that our algorithm can attain a convergence rate which has the same order as decentralized algorithms over stable networks, and achieves linear speedup comparing with centralized ones. Moreover, the proposed algorithm also applies to the general case that the data are not independently and identically distributed. Extensive experiments on image classification demonstrate that the practical performance of our algorithm is comparable with the state-of-art decentralized algorithms in stable networks with only a little accuracy loss.
更多查看译文
关键词
Decentralized learning,Stochastic gradient descent,Unstable networks
AI 理解论文
溯源树
样例
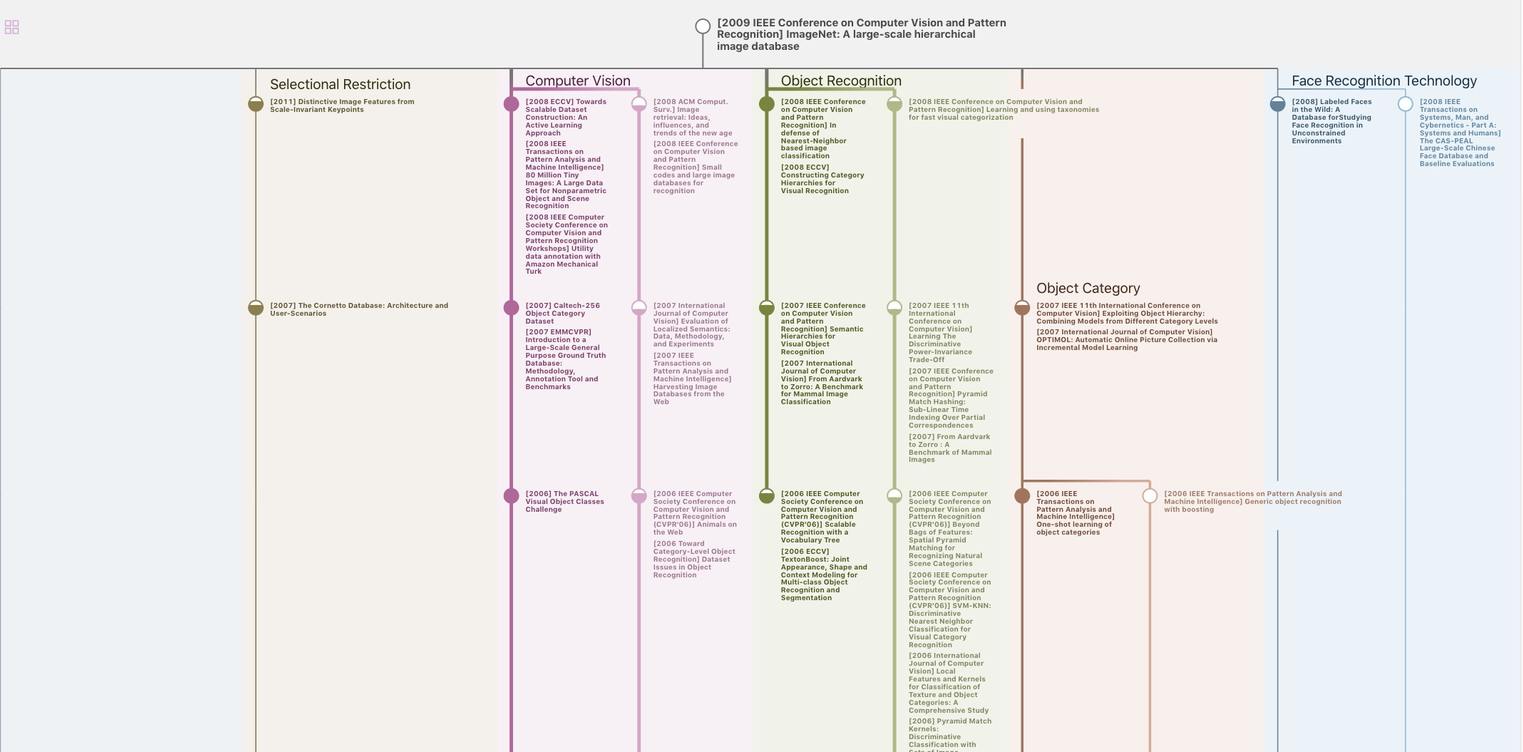
生成溯源树,研究论文发展脉络
Chat Paper
正在生成论文摘要