Task offloading in Multiple-Services Mobile Edge Computing: A deep reinforcement learning algorithm.
Comput. Commun.(2023)
摘要
Multiple-Services Mobile Edge Computing enables task-relate services cached in edge server to be dynamically updated, and thus provides great opportunities to offload tasks to edge server for execution. However, the requirements and popularity of services, the computing requirement and the amount of data transferred from users to edge server are dynamic with time. How to adaptively adjust the subset of total service types in the resource-limited edge server and determine the task offloading destination and resource allocation decisions to improve the overall system performance is a challenge problem. To solve this challenge, we firstly convert it into a Markov decision process, then propose a soft actor-critic deep reinforcement learning-based algorithm, called DSOR, to jointly determine not only the discrete decisions of service caching and task offloading but also the continuous allocation of bandwidth and computing resource. To improve the accuracy of our algorithm, we employ an efficient trick of converting the discrete action selection into a continuous space to deal with the key design challenge that arises from continuous-discrete hybrid action space. Additionally, to improve resource utilization, a novel reward function is integrated to our algorithm to speed up the convergence of training while making full use of system resources. Extensive numerical results show that compared with other baseline algorithms, our algorithm can effectively reduce the long-term average completion delay of tasks while accessing excellent performance in terms of stability.
更多查看译文
关键词
Mobile edge computing,Service caching,Task offloading,Resource allocation,Deep reinforcement learning
AI 理解论文
溯源树
样例
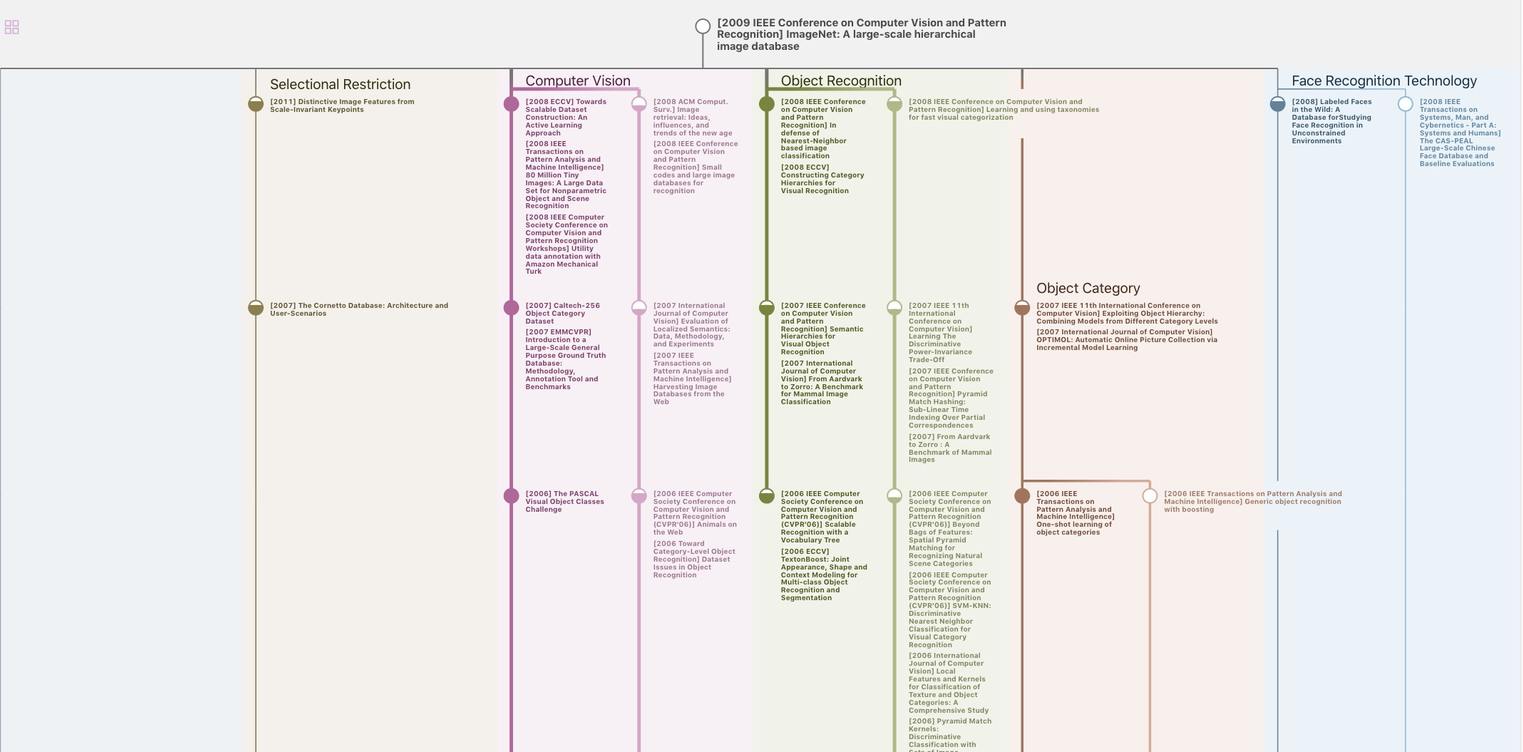
生成溯源树,研究论文发展脉络
Chat Paper
正在生成论文摘要