K-FL: Kalman Filter-Based Clustering Federated Learning Method
IEEE Access(2023)
摘要
Federated learning is a distributed machine learning framework that enables a large number of devices to cooperatively train a model without data sharing. However, because federated learning trains a model using non-independent and identically distributed (non-IID) data stored at local devices, the weight divergence causes a performance loss. This paper focuses on solving the non-IID problems and proposes Kalman filter-based clustering federated learning method called K-FL to get performance gain by providing a specific model with low variance to the device. To the best of our knowledge, it is the first clustering federated learning method that can train a model requiring fewer communication rounds under the premise that non-IID environment without any prior knowledge and an initial value set by the user. From simulations, we demonstrate that the proposed K-FL can train a model much faster, requiring fewer communication rounds than FedAvg and LG-FedAvg when testing neural networks using the MNIST, FMNIST, and CIFAR10 datasets. As a numerical result, it is shown that the accuracy is improved in all datasets while the computational time cost is reduced by 1.43x, 1.67x, and 1.63x compared to FedAvg, respectively.
更多查看译文
关键词
Federated learning,distributed machine learning,clustering method,kalman filter
AI 理解论文
溯源树
样例
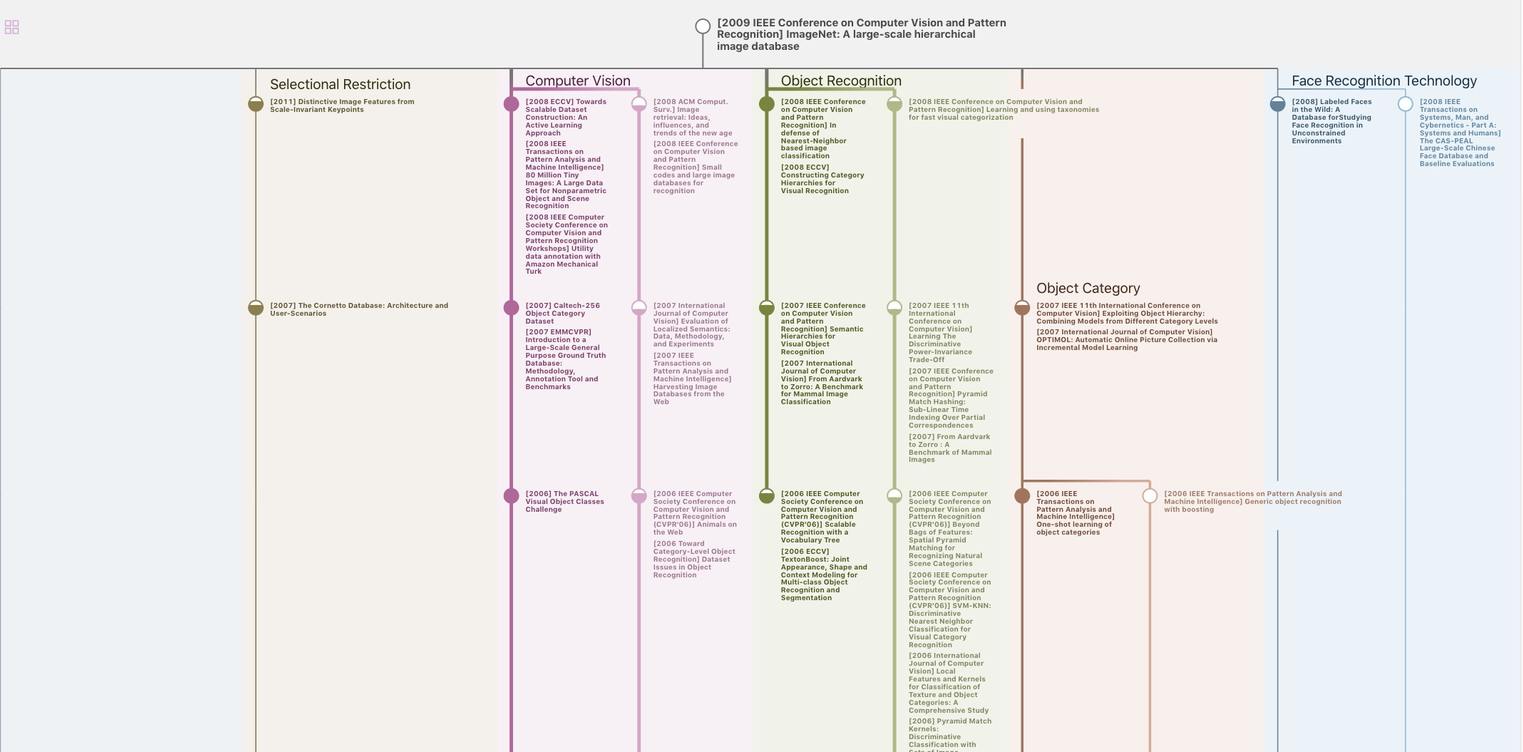
生成溯源树,研究论文发展脉络
Chat Paper
正在生成论文摘要