A unified speech enhancement approach to mitigate both background noises and adversarial perturbations.
Inf. Fusion(2023)
摘要
Deep learning has been vastly applied in classification systems of speech signals. However, adversarial examples crafted by adding small perturbations to input speech signals can make those classification systems produce incorrect results. Beyond detecting and discarding adversarial examples when securing the classification sys-tems, we consider to purify the input adversarial examples to normal ones in this paper. Speech enhancement is a technique known as removing background noises to enhance voice quality. By treating adversarial per-turbations as noises, we hereby propose a unified speech enhancement approach to mitigate both background noises and adversarial perturbations. The approach is named by Speech Enhancement and Adversarial Defense Network (SEADNet), which performs model-level fusion of conventional speech enhancement and adversarial defenses. In SEADNet, a learnable noise-adding module is firstly created to adaptively putting noises to the input speech to destroy the functions of adversarial perturbations. Subsequently, a speech enhancement network is deployed to reconstruct clean speech from noisy inputs. Finally, an adversarial example detection module is designed as a discriminator to guide the reconstructed speech in the previous speech enhancement network to mitigate adversarial perturbations. The overall network is optimized by minimizing a joint loss function. Experiments are conducted on the tasks of speech enhancement and automatic speaker verification with benchmark datasets. The proposed SEADNet performs well on both tasks of conventional noise mitigation and adversarial defenses compared to the related competitive baselines.
更多查看译文
关键词
Speech enhancement,Noise mitigation,Adversarial defense,Model fusion,Automatic speaker verification
AI 理解论文
溯源树
样例
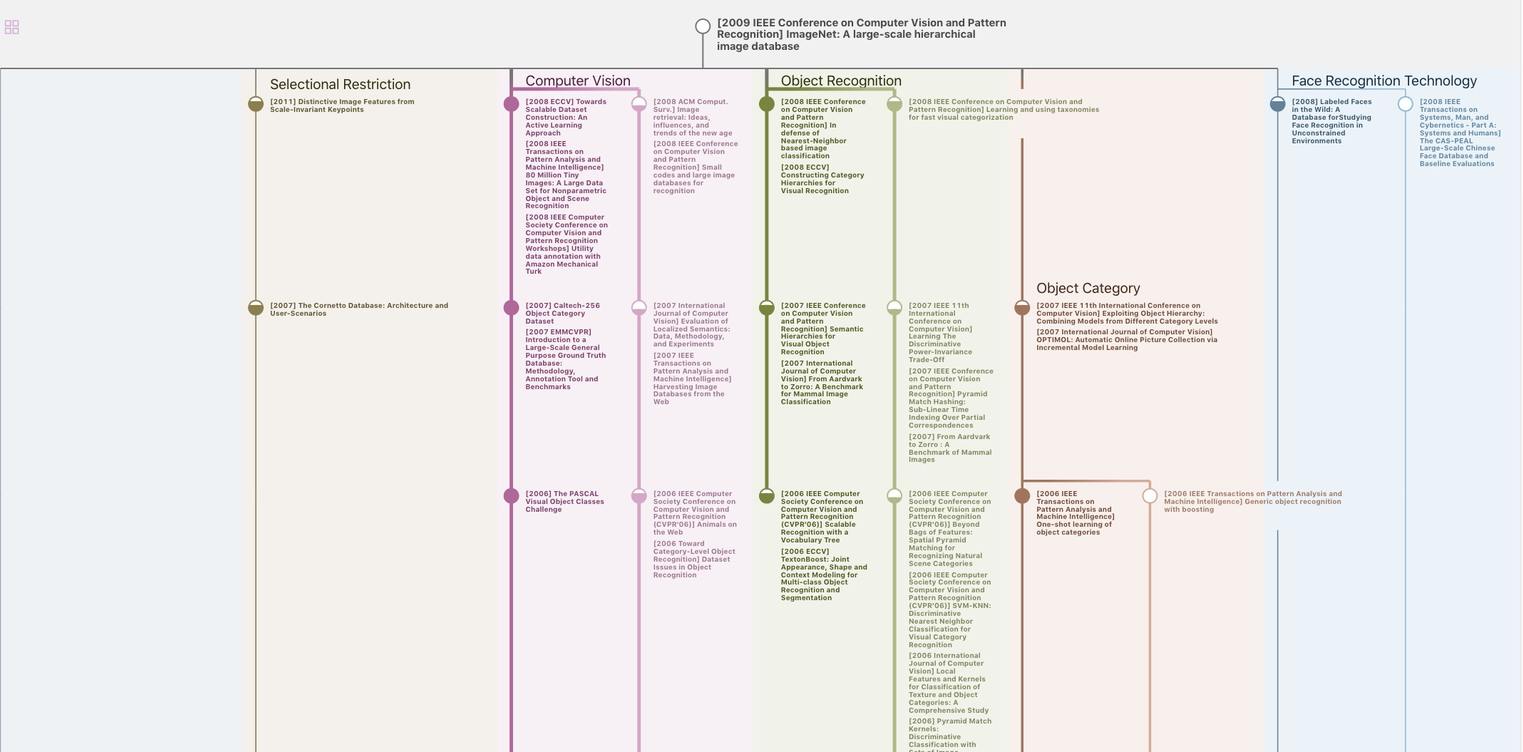
生成溯源树,研究论文发展脉络
Chat Paper
正在生成论文摘要