Learning for Feature Matching via Graph Context Attention.
IEEE Trans. Geosci. Remote. Sens.(2023)
摘要
Establishing reliable correspondences via a deep learning network is an important task in remote sensing, photogrammetry, and other computer vision fields. It usually requires mining the relationship among correspondences to aggregate both local and global contexts. However, current methods are insufficient to effectively acquire context information with high reliability. In this article, we propose a graph context attention-based network (GCA-Net) to capture and leverage abundant contextual information for feature matching. Specifically, we design a graph context attention block, which generates multipath graph contexts and softly fuses them to combine respective advantages. In addition, for building the graph context containing stronger representation ability and outlier resistance ability, we further design a local-global channel mining block to gather context information by focusing on the significant part as well as to mine dependencies among channels of correspondences in both local and global aspects. The proposed GCA-Net is able to effectively infer the probability of correspondences being inliers or outliers and estimate the essential matrix meanwhile. Extensive experimental results for outlier removal and relative pose estimation demonstrate that GCA-Net outperforms the state-of-the-art methods on both outdoor and indoor datasets (i.e., YFCC100M and SUN3D). In addition, experiments extended to remote sensing and point cloud scenes also demonstrate the powerful generalization capability of our network.
更多查看译文
关键词
feature matching,attention,graph
AI 理解论文
溯源树
样例
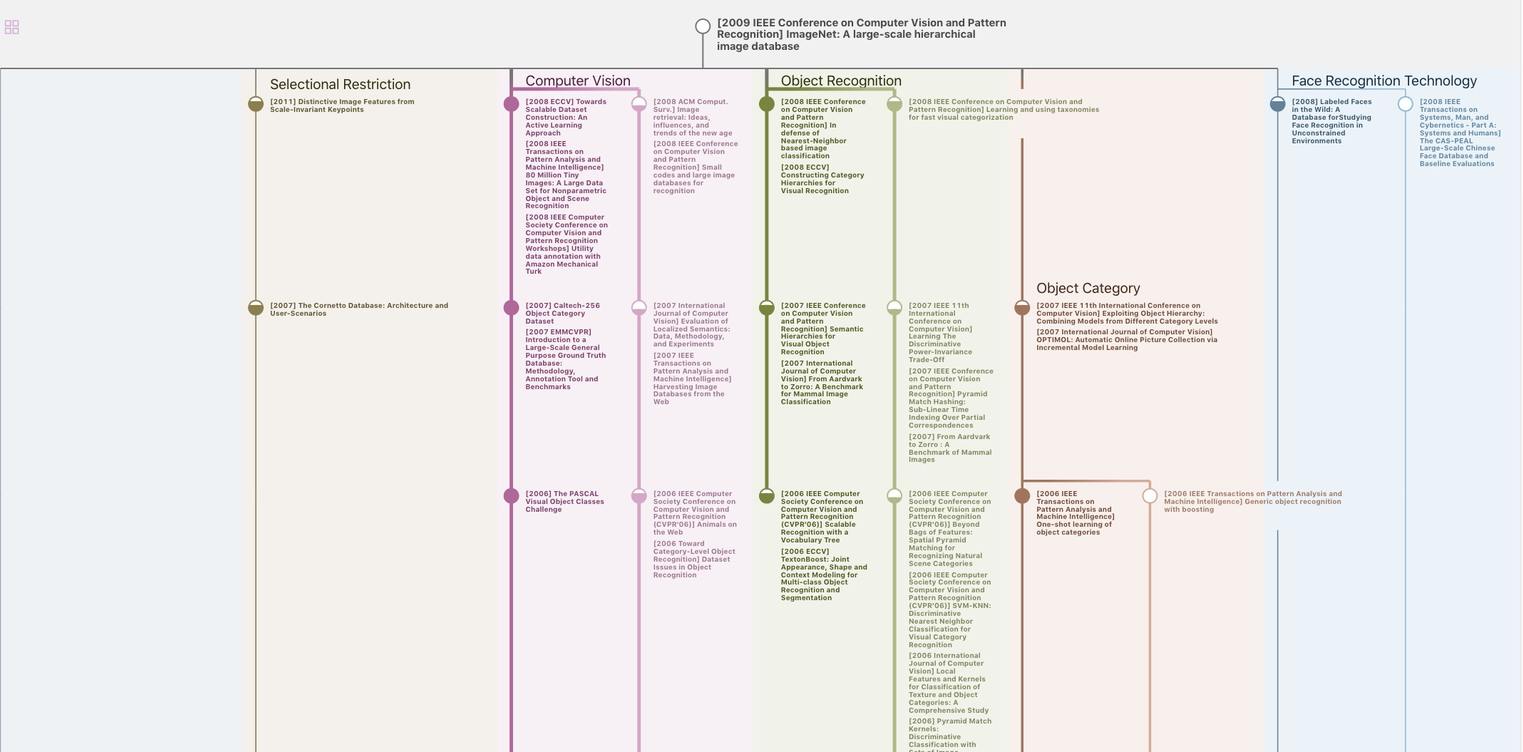
生成溯源树,研究论文发展脉络
Chat Paper
正在生成论文摘要