FDPBoost: Federated differential privacy gradient boosting decision trees.
J. Inf. Secur. Appl.(2023)
摘要
The big data era has led to an exponential increase in data usage, resulting in significantly advancements in data-driven domains and data mining. However, due to privacy and regulatory requirements, sharing data among various institutions is not always possible. Federated learning can help address this problem, but existing studies that combine differential privacy with tree models have shown significant accuracy loss. In this study, we propose a Federated Differential Privacy Gradient Boosting Decision Tree (FDPBoost) that protects the private datasets of different owners while improving model accuracy. We select sensitive features according to the secure feature set indicator, and use an exponential mechanism to protect sensitive features and assign significant weight to the Laplace mechanism to protect leaf node values. Additionally, a distributed two -level boosting framework is designed to allocate the privacy budget between intra-iteration and inter-iteration decision trees while protecting model communication. The FDPBoost is tested on five datasets sourced from the materials and medical domains. Our experiments reveal that FDPBoost achieves competitive accuracy with traditional federated gradient boosting decision trees while also exhibiting a significant reduction in error rate as compared to PPGBDT (Zhao et al.) and FV-tree (Gao et al.). Notably, FDPBoost's error rate on the tumor-diagnosis dataset is 30% lower than that of FV-tree.
更多查看译文
关键词
Federated learning,Differential privacy,Gradient boosting decision tree,Distributed two-level boosting framework
AI 理解论文
溯源树
样例
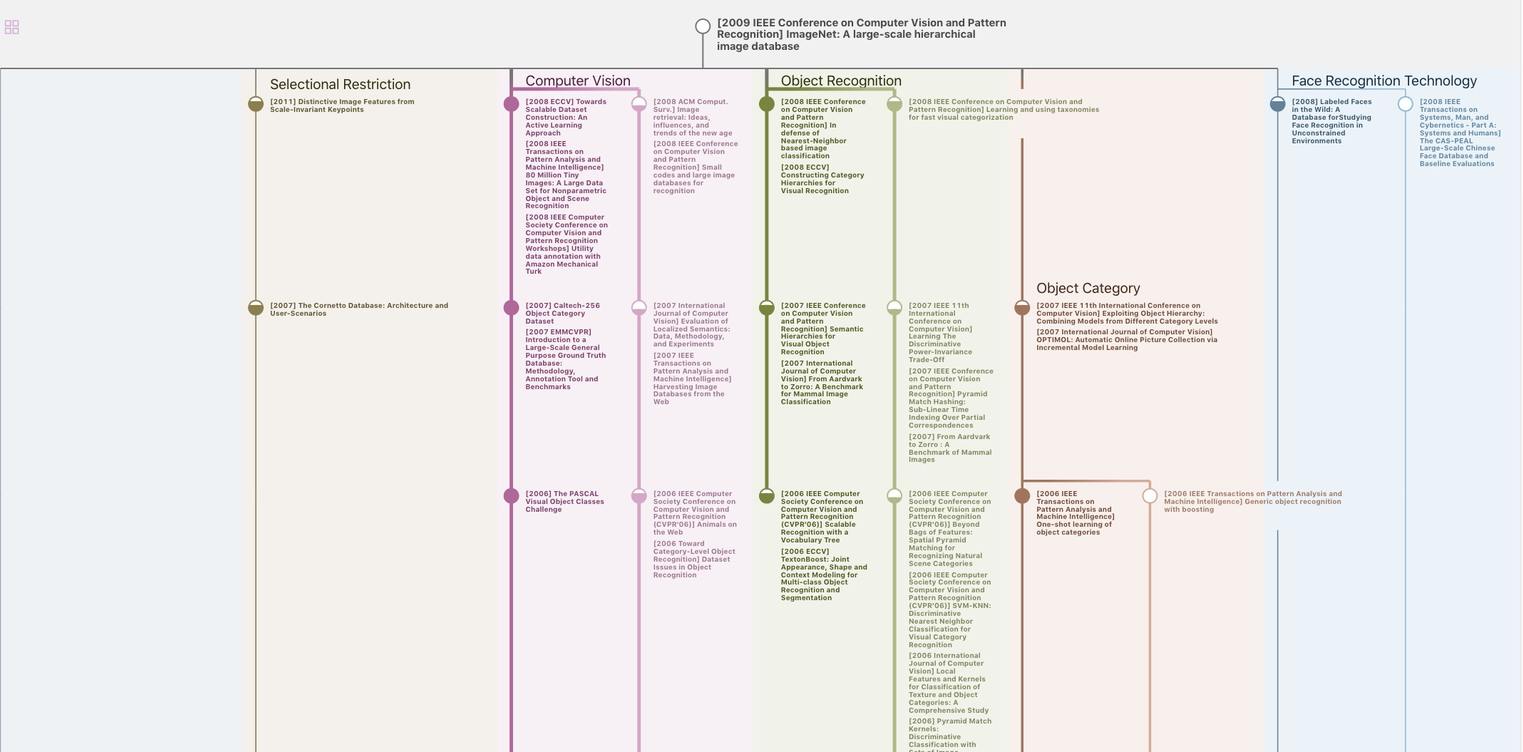
生成溯源树,研究论文发展脉络
Chat Paper
正在生成论文摘要