Load balancing inside programmable data planes based on network modeling prediction using a GNN with network behaviors.
Comput. Networks(2023)
摘要
In data center networks, existing control plane-and end host-based load-balancing methods are encumbered by excessively large decision delays during rapid reactions to microbursts. However, existing programmable data plane-based load balancing methods require large overheads involved in active probing. Accurate network modeling can optimize load balancing. However, existing modeling methods suffer from low generalization and high overhead. In this study, we propose a network modeling method based on a graph neural network (GNN) with basic network behavior (hereinafter called GNN-Behavior). This is derived from two inherent correlations observed: the correlation between global network behavior and basic network behavior and the correlation among basic network behaviors. We employed the GNN with an improved message-passing neural network to learn such two inherent correlations. Particularly, we considered modeling end-to-end delay as a use case to validate GNN-Behavior. Furthermore, we propose a packet-level load-balancing scheme inside programmable data planes (PDPs) based on the accurate prediction of end-to-end delay from the GNN-Behavior model(LBPP). LBPP is a control plane-PDP collaborative method that integrates a global view from a controller and quick response from switches. Experimental results demonstrate the feasibility and effectiveness of the GNN-Behavior and LBPP. Compared with queuing theory (QT), RouteNet, and GNN-based scheme, GNN-Behavior increases goodness of fit (R-2) by 73.1%, 11.1%, and 3.74%, respectively. Under an unknown traffic control strategy, the generalization ability of GNN-Behavior is considerably better than that of QT and RouteNet. Compared with flow-level ECMP, flowlet-level LetFlow, and packet-level DRILL, LBPP can reduce average flow completion time by up to 43.9%, 37.4%, and 17.2%, respectively.
更多查看译文
关键词
network modeling prediction,programmable data planes,gnn
AI 理解论文
溯源树
样例
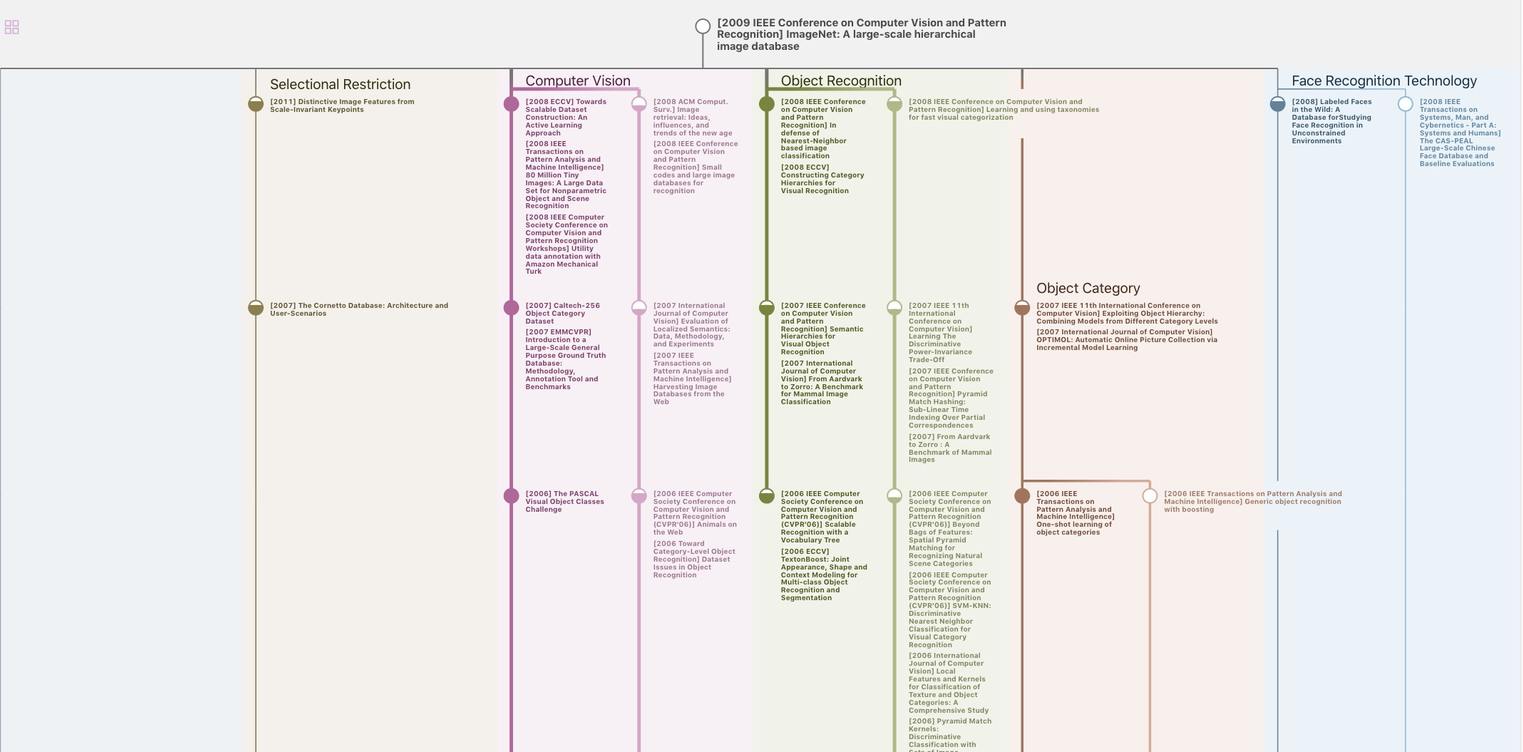
生成溯源树,研究论文发展脉络
Chat Paper
正在生成论文摘要