Collaboration among Image and Object Level Features for Image Colourisation.
CoRR(2021)
摘要
Image colourisation is an ill-posed problem, with multiple correct solutions which depend on the context and object instances present in the input datum. Previous approaches attacked the problem either by requiring intense user interactions or by exploiting the ability of convolutional neural networks (CNNs) in learning image level (context) features. However, obtaining human hints is not always feasible and CNNs alone are not able to learn object-level semantics unless multiple models pretrained with supervision are considered. In this work, we propose a single network, named UCapsNet, that separate image-level features obtained through convolutions and object-level features captured by means of capsules. Then, by skip connections over different layers, we enforce collaboration between such disentangling factors to produce high quality and plausible image colourisation. We pose the problem as a classification task that can be addressed by a fully self-supervised approach, thus requires no human effort. Experimental results on three benchmark datasets show that our approach outperforms existing methods on standard quality metrics and achieves a state of the art performances on image colourisation. A large scale user study shows that our method is preferred over existing solutions.
更多查看译文
关键词
object level features,collaboration,image
AI 理解论文
溯源树
样例
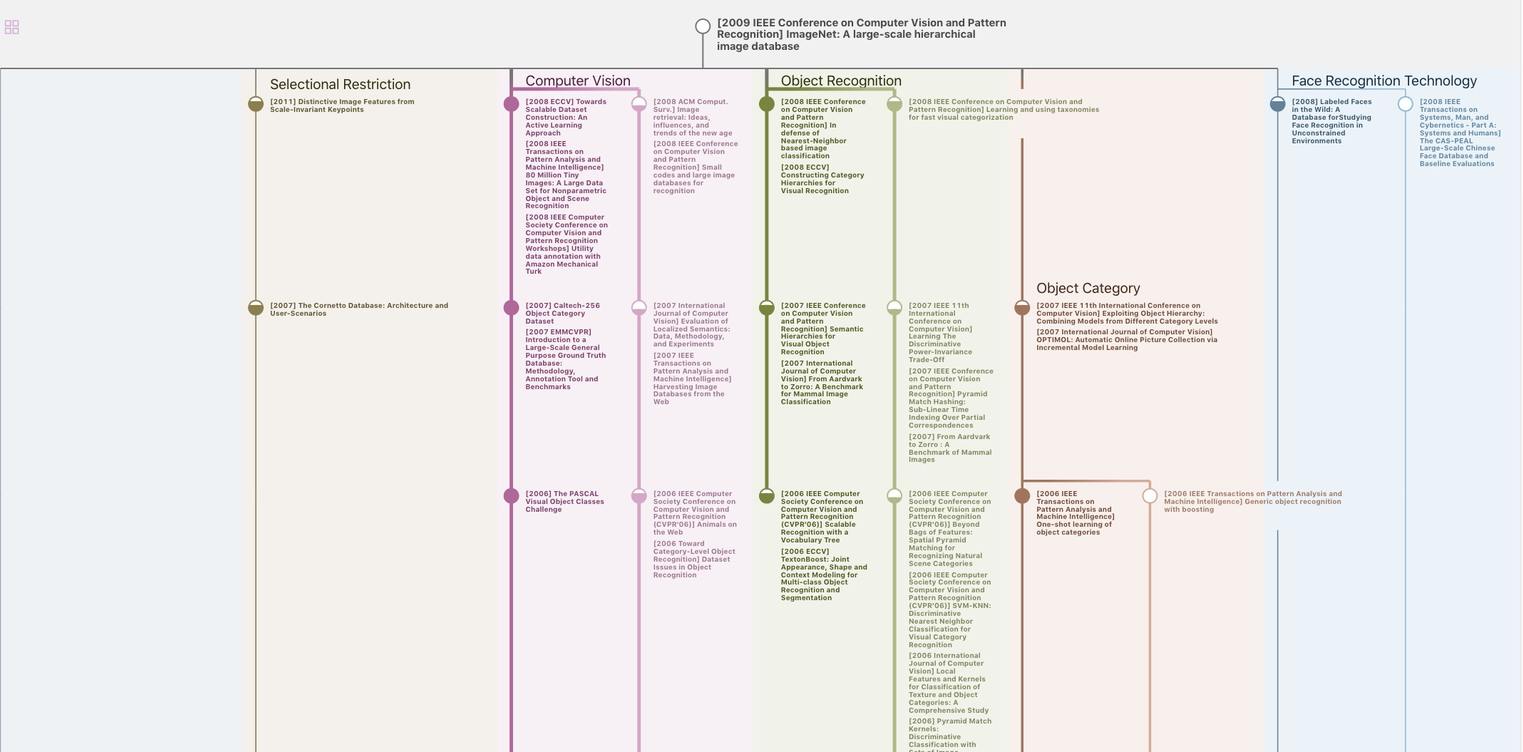
生成溯源树,研究论文发展脉络
Chat Paper
正在生成论文摘要